This article has been reviewed according to Science X's editorial process and policies. Editors have highlighted the following attributes while ensuring the content's credibility:
fact-checked
peer-reviewed publication
trusted source
proofread
Predicting metabolic potential in bacteria from limited genome data
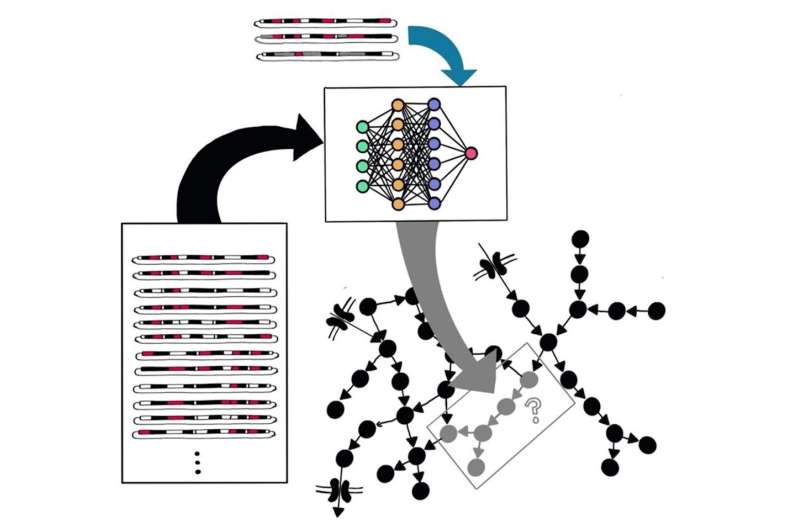
How bacteria eat food, and what kinds of products they can make from that food, is dictated by the metabolic network of enzyme patterns encoded in their genomes. Using computational methods to learn these patterns across a large number of known bacteria allows the genome of a new bacteria to be analyzed. This reveals what kind of metabolism it is capable of—even when only partial information is provided, which is common in environmental samples.
This project was initiated by David Geller-McGrath as part of his graduate thesis project at the Woods Hole Oceanographic Institute under Dr. Edgcomb. He refined the approaches and developed the code during his time as an Office of Science Graduate Student Research fellowship in computational biosciences working with Dr. McDermott at Pacific Northwest National Laboratory, and with Dr. Wheeler at the University of Arizona.
This new computational method, now published in eLife, enables the discovery of new metabolic capabilities for bacteria important for the environment and bioenergy applications. This is important for understanding microbiomes (communities of bacteria and other microorganisms) that support plant growth for improved crop yields. In addition, a better understanding of different metabolic networks will allow new ways of engineering bacteria for other bioenergy and biomedical applications.
The method learns patterns of proteins present in metabolic pathways from a large collection of annotated bacterial genomes using a deep learning approach. A significant advantage of this tool is that it is designed and tested on incomplete genomic data. This allows bacterial genomes to be identified and assessed for metabolic potential in complex microbiomes from soil or other sources, samples that are often incomplete.
More information: David Geller-McGrath et al, Predicting metabolic modules in incomplete bacterial genomes with MetaPathPredict, eLife (2024). DOI: 10.7554/eLife.85749
Journal information: eLife
Provided by Pacific Northwest National Laboratory