This article has been reviewed according to Science X's editorial process and policies. Editors have highlighted the following attributes while ensuring the content's credibility:
fact-checked
peer-reviewed publication
trusted source
proofread
Researchers develop AI model that predicts the accuracy of protein–DNA binding
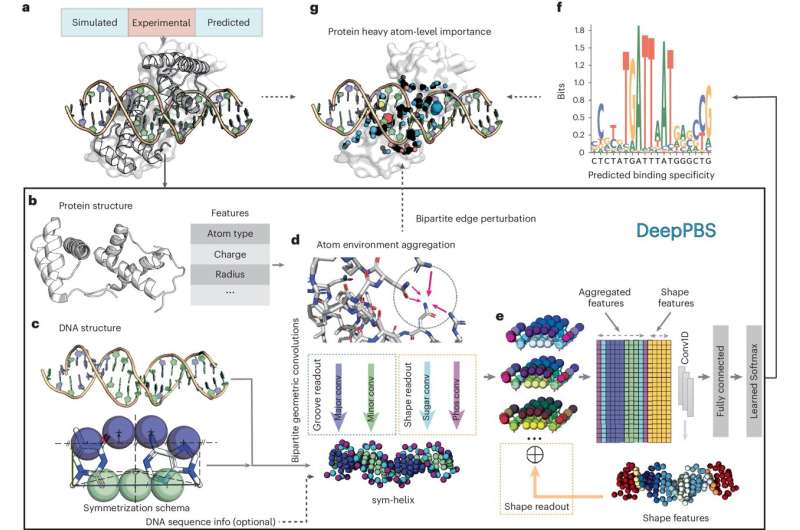
A new artificial intelligence model developed by USC researchers and published in Nature Methods can predict how different proteins may bind to DNA with accuracy across different types of protein, a technological advance that promises to reduce the time required to develop new drugs and other medical treatments.
The tool, called Deep Predictor of Binding Specificity (DeepPBS), is a geometric deep learning model designed to predict protein–DNA binding specificity from protein–DNA complex structures. DeepPBS allows scientists and researchers to input the data structure of a protein–DNA complex into an online computational tool.
"Structures of protein–DNA complexes contain proteins that are usually bound to a single DNA sequence. For understanding gene regulation, it is important to have access to the binding specificity of a protein to any DNA sequence or region of the genome," said Remo Rohs, professor and founding chair in the department of Quantitative and Computational Biology at the USC Dornsife College of Letters, Arts and Sciences.
"DeepPBS is an AI tool that replaces the need for high-throughput sequencing or structural biology experiments to reveal protein–DNA binding specificity."
AI analyzes, predicts protein–DNA structures
DeepPBS employs a geometric deep learning model, a type of machine-learning approach that analyzes data using geometric structures. The AI tool was designed to capture the chemical properties and geometric contexts of protein–DNA to predict binding specificity.
Using this data, DeepPBS produces spatial graphs that illustrate protein structure and the relationship between protein and DNA representations. DeepPBS can also predict binding specificity across various protein families, unlike many existing methods that are limited to one family of proteins.
"It is important for researchers to have a method available that works universally for all proteins and is not restricted to a well-studied protein family. This approach allows us also to design new proteins," Rohs said.
Major advance in protein-structure prediction
The field of protein-structure prediction has advanced rapidly since the advent of DeepMind's AlphaFold, which can predict protein structure from sequence. These tools have led to an increase in structural data available to scientists and researchers for analysis. DeepPBS works in conjunction with structure prediction methods for predicting specificity for proteins without available experimental structures.
Rohs said the applications of DeepPBS are numerous. This new research method may lead to accelerating the design of new drugs and treatments for specific mutations in cancer cells, as well as lead to new discoveries in synthetic biology and applications in RNA research.
More information: Raktim Mitra et al, Geometric deep learning of protein–DNA binding specificity, Nature Methods (2024). DOI: 10.1038/s41592-024-02372-w
Journal information: Nature Methods
Provided by University of Southern California