The AI-driven initiative that's hastening the discovery of drugs to treat COVID-19
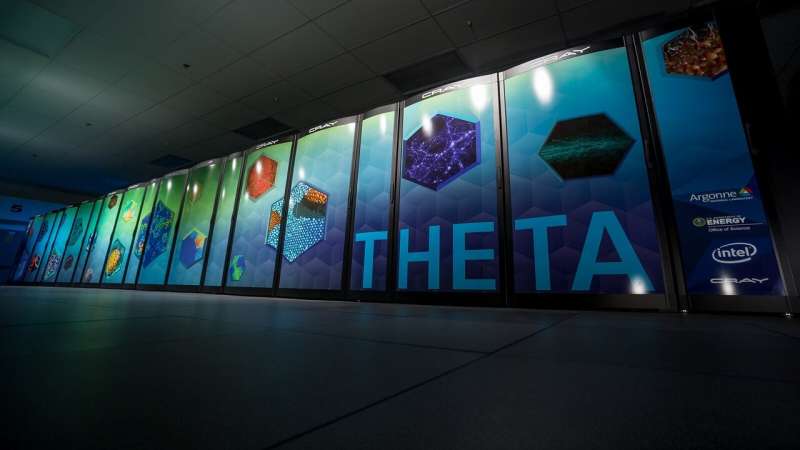
A novel pipeline of AI and simulation tools may make the process of screening drug candidates for COVID-19 50,000 times faster.
To find a drug that can stop the SARS-CoV-2 virus, scientists want to screen billions of molecules for the right combination of properties. The process is usually risky and slow, often taking several years. However, an international team of scientists say they've found a way to make the process 50,000 times faster using artificial intelligence (AI).
Ten organizations, including the U.S. Department of Energy's (DOE) Argonne National Laboratory, have developed a pipeline of AI and simulation techniques to hasten the discovery of promising drug candidates for COVID-19, the disease caused by the SARS-CoV-2 virus. The pipeline is named IMPECCABLE, short for Integrated Modeling PipelinE for COVID Cure by Assessing Better Leads.
"With the AI we've implemented, we've been able to screen four billion potential drug candidates in a matter of a day, while existing computational tools might only realistically screen one to 10 million," said Thomas Brettin, strategic program manager at Argonne.
Why an integrated approach is needed
IMPECCABLE integrates multiple techniques for data processing, physics-based modeling and simulation, and machine learning, a form of AI that uses patterns in data to generate predictive models.
"We integrate multiple approaches because there's no single algorithm or method that can single-handedly work with great efficiency and accuracy," said Argonne computational biologist Arvind Ramanathan. "If we only relied on simulations, it would take us years to find a likely target, even with the fastest supercomputers."
Components of the pipeline
At the start of the pipeline, computational techniques are used to calculate the basic properties of billions of molecules. This data is used in the next stage of the pipeline to create machine learning models that can predict how likely it is that a given molecule will bind with a known viral protein. Those found to be most promising are then simulated on high-performance computing systems.
"Proteins are fluid structures, and simulations show us new conformations for them. We use those to improve our machine learning models," said Argonne computational scientist Austin Clyde. "The iterative process continues until we can validate that the molecules we've identified as likely to bind to SARS-CoV-2 proteins have promise."
Very large experimental data sets are also being gathered from thousands of protein crystals using X-rays at the Advanced Photon Source (APS), a DOE Office of Science User Facility on Argonne's campus. The technique they're using to get this data is known as X-ray crystallography. With it, researchers can capture detailed images of viral proteins and their chemical states to improve the accuracy of their machine learning models.
"Since the beginning of the pandemic, we've been able to determine over 45 high-resolution crystal structures of SARS-CoV-2 proteins and their complexes with other compounds. This information, when combined with computational analysis, can provide critical insights for further structure-based drug design efforts and enable the design of higher affinity inhibitors and, ultimately therapeutics that can be used to treat COVID-19," said Andrzej Joachimiak, director of the Structural Biology Center (SBC) at beamline 19-ID-D of the APS.
The ultimate goals of the pipeline are to (1) understand the function of viral proteins; (2) identify molecules with a high potential to bind with these proteins and, as a result, block SARS-CoV-2 proliferation; and (3) deliver this insight to drug designers and developers for further research and development.
"Unlike the traditional approach, where you rely on the scientist to think really hard and, based on what they know, come up with ideas for a molecule, with our pipeline you can screen huge numbers of molecules automatically, dramatically increasing your chance of finding a likely candidate," said Ian Foster, director of Argonne's Data Science and Learning division.
Provided by Argonne National Laboratory