This article has been reviewed according to Science X's editorial process and policies. Editors have highlighted the following attributes while ensuring the content's credibility:
fact-checked
trusted source
proofread
Harnessing hyperspectral imaging and machine learning for rubber tree nutrient management
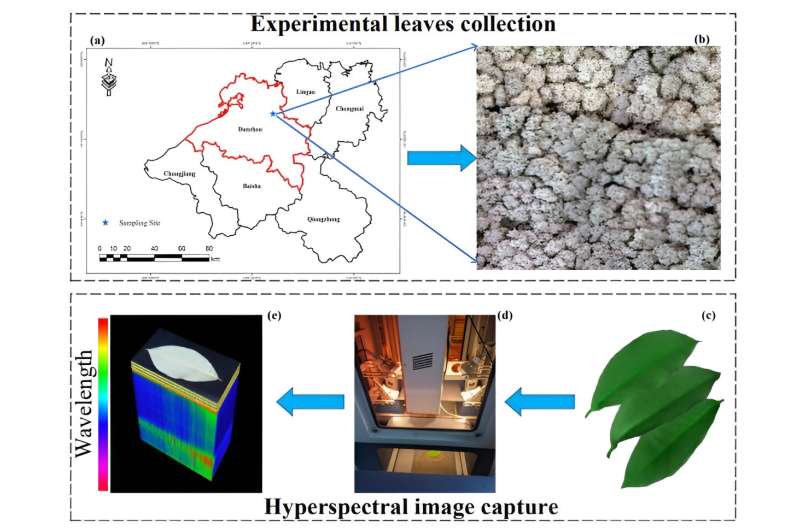
Rubber trees are essential for natural rubber, and require precise nutrient management. Traditional methods for assessing nutrient levels are expensive and destructive, but near-infrared (NIR) hyperspectral techniques offer a promising nondestructive alternative. Challenges arise with high-dimensional data, leading to biased results from small and imbalanced datasets. Current research focuses on overcoming these limitations using machine learning and radiative transfer models.
A potential solution involves integrating unlabeled hyperspectral data with labeled samples through semi-supervised learning and resampling techniques, aiming for accurate and efficient monitoring of nitrogen and potassium levels in rubber leaves without the intensive labor required by traditional methods.
In January 2024, Plant Phenomics published a research article titled "Handling the challenges of small-scale labelled data and class imbalances in classifying the N and K statuses of rubber leaves using hyperspectroscopy techniques."
The research utilized various resampling techniques to balance data classes during model learning. The results demonstrated that models with data balance constraints significantly outperformed those without. Visualizations showed how techniques like MES and SMOTE evenly distributed samples across classes, while the RES method effectively reduced class distribution skewness. The MES and SMOTE techniques emerged as the most effective, notably enhancing classification performance in semi-supervised learning (SSL) environments, particularly for nitrogen (N) and potassium (K) levels in rubber leaves.
SSL methods, leveraging unlabeled hyperspectral imaging (HSI) data, outshone traditional supervised learning, with notable improvements in weighted average precision (WAP), mean average precision (MAP), and weighted recall (WR) scores. The research showed that certain NIR wavelengths in identifying N and K levels, underscoring the precision and reliability of the spectral classifiers used.
Further analysis revealed that a balanced mix of labeled and unlabeled data significantly boosts the classification accuracy, with the optimal ratio of labeled to unlabeled data enhancing performance metrics.
Overall, the study's approach to rebalancing the data and utilizing SSL with HSI data not only addressed the challenges of small sample sizes and data imbalance but also proved efficient and effective for nutrient level detection in rubber leaves. This methodology opens new avenues for applying near-infrared hyperspectral imaging in agriculture, particularly for monitoring crop nutrient levels under conditions of limited spectral data samples and imbalance.
More information: Wenfeng Hu et al, Handling the challenges of small-scale labelled data and class imbalances in classifying the N and K statuses of rubber leaves using hyperspectroscopy techniques, Plant Phenomics (2024). DOI: 10.34133/plantphenomics.0154
Provided by NanJing Agricultural University