This article has been reviewed according to Science X's editorial process and policies. Editors have highlighted the following attributes while ensuring the content's credibility:
fact-checked
trusted source
proofread
Enhancing crop nutritional analysis: Precision agriculture with multi-target regression and hyperspectral imaging
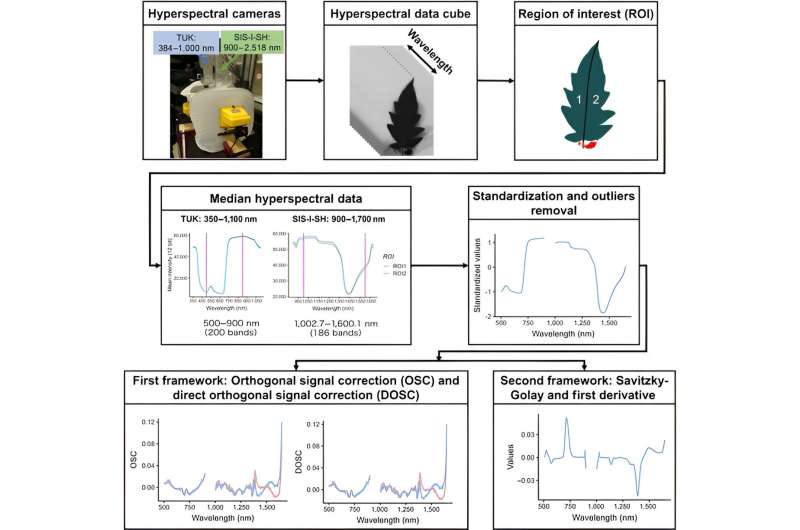
Recent advancements in hyperspectral imaging and machine learning have revolutionized the non-destructive monitoring of crop nutritional status, enabling accurate prediction of plant element concentrations. Despite successes, the single-target regression method, which predicts concentrations individually, faces accuracy limitations for certain elements.
Traditional methods offer accuracy but at the cost of being destructive and inefficient for large-scale use. Current research highlights the potential of multi-target regression, which considers relationships between multiple elements, as a promising avenue for enhancing prediction accuracy. Yet, the application of multi-target regression in predicting multiple element concentrations in plants remains underexplored and represents a critical area for future investigation.
Plant Phenomics published research titled "A Multi-Target Regression Method to Predict Element Concentrations in Tomato Leaves Using Hyperspectral Imaging."
This study aimed to enhance the accuracy of predicting element concentrations in tomato leaves through a multi-target regression (MTSC) method, which integrates sequential chaining of predicted element values into original hyperspectral imaging inputs.
Researchers applied this approach to 17 elements and compared it against traditional single-target regression (STR) outcomes, utilizing five different machine learning models. The MTSC method showed a significant improvement in prediction accuracy for 10 elements, with notable increases in the coefficient of determination (R2) for elements like Mn, Cu, Co, Fe, and Mg, demonstrating its superiority over STR.
Their experiments evaluated the variability of the hyperspectral data and showed significant differences within and across tomato plants, which underlines the data's complexity. Initial predictions using STR revealed that certain elements, such as Mg, Mo, and Ca, had relatively higher R2 values, indicating decent model performance.
However, for elements with lower prediction accuracies in STR, MTSC offered a promising alternative, significantly improving accuracy for a subset of elements, including Mg, P, S, Mn, Fe, Co, Cu, Sr, Mo, and Cd. This improvement was most pronounced in the Random Forest (RF) model, which showed the highest average increases in R2 and reductions in root mean square error (RMSE).
The effectiveness of the MTSC method was further validated on a test dataset, with predictions for the 10 improved elements outperforming STR predictions. This method's success highlights the importance of considering inter-element relationships and the potential of non-linear machine learning models like RF to exploit these connections for better prediction accuracy.
Overall, these findings underscore the MTSC method's capability to enhance element concentration predictions in plant tissues significantly and illustrate the method's advantage in incorporating complex inter-elemental relationships, thereby offering a more accurate and holistic approach to predicting plant nutritional status.
More information: Andrés Aguilar Ariza et al, A Multi-Target Regression Method to Predict Element Concentrations in Tomato Leaves Using Hyperspectral Imaging, Plant Phenomics (2024). DOI: 10.34133/plantphenomics.0146
Provided by NanJing Agricultural University