This article has been reviewed according to Science X's editorial process and policies. Editors have highlighted the following attributes while ensuring the content's credibility:
fact-checked
proofread
Unlocking tree genetic diversity: Advanced hyperspectral phenotyping for enhanced Scots pine selection
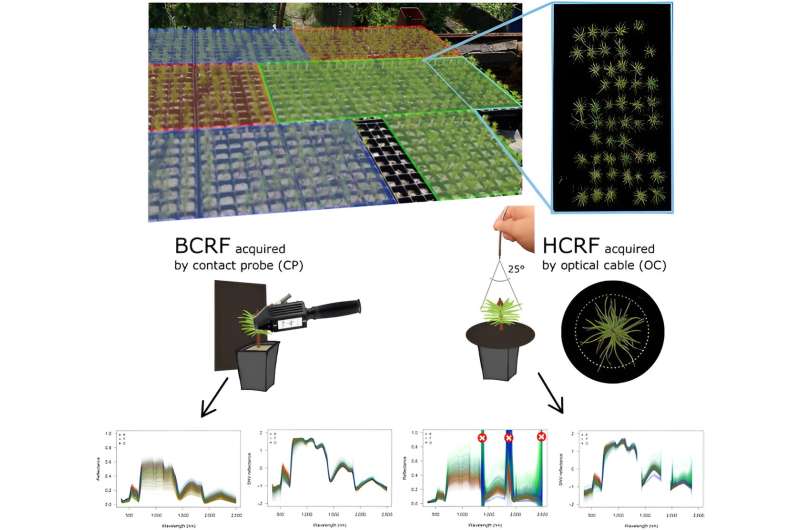
Hyperspectral reflectance reveals critical leaf functional traits indicative of a plant's physiological status, providing a powerful tool for distinguishing seedlings adapted to specific environments.
Current research explores intrapopulation variability and the necessity of high-throughput phenotyping (HTP) in forestry for selection of resilient genotypes under changing climatic conditions. However, challenges persist in managing large-scale phenotypic data and in the compatibility of reflectance data acquired from various measurement approaches.
Plant Phenomics published a research article titled "Making the Genotypic Variation Visible: Hyperspectral Phenotyping in Scots Pine Seedlings."
This research utilized two non-destructive methods to measure hyperspectral reflectance on 1,788 Scots pine seedlings, distinguishing between lowland and upland ecotypes from the Czech Republic.
Leaf level measurements were performed with a spectroradiometer and contact probe (CP) for the biconical reflectance factor (BCRF) of needle samples, while proximal canopy measurements employed the same spectroradiometer with a fiber optical cable (OC) under natural light for hemispherical conical reflectance factor (HCRF). Results showed statistically significant differences among pine populations across the entire spectral range.
Using machine learning algorithms, the proximal data predicted the different Scots pine populations with up to 83% accuracy.
Specifically, BCRF and HCRF indicated significant differences in pairwise comparisons among populations, particularly in visible (VIS) and near-infrared (NIR) regions. The most pronounced differences occurred in VIS and red edge (RE) for BCRF, while HCRF showed more variance in shortwave infrared (SWIR) regions.
Both BCRF and HCRF data maintained similar trends across the very shortwave infrared (VSWIR) spectral range, with BCRF P values generally closer to zero than HCRF in many spectral intervals. Random Forest (RF) and Support Vector Machine (SVM) algorithms were employed to test the prediction accuracy of population origin based on reflectance factors.
The highest accuracy was obtained from raw whole seedling HCRF. The importance of specific spectral regions for RF separation was evidenced by peaks in VIS and RE. HCRF displayed more spectral regions with high importance for RF prediction than BCRF, which was mainly limited to VIS and RE. This difference likely contributed to the higher prediction accuracy of RF models based on HCRF data.
The study concluded that both leaf-level BCRF and whole seedling HCRF are suitable for hyperspectral phenotyping to differentiate the phenotypic and genetic variation within Scots pine seedlings.
Overall, these methods offer valuable tools for forestry and breeding programs, particularly for non-destructive genetic evaluation and effective nursery practices. Despite some limitations related to light conditions and measurement methods, the research demonstrated the potential of using hyperspectral reflectance and machine learning for accurate prediction and classification of tree populations in breeding and conservation efforts.
More information: Jan Stejskal et al, Making the Genotypic Variation Visible: Hyperspectral Phenotyping in Scots Pine Seedlings, Plant Phenomics (2023). DOI: 10.34133/plantphenomics.0111
Provided by Plant Phenomics