This article has been reviewed according to Science X's editorial process and policies. Editors have highlighted the following attributes while ensuring the content's credibility:
fact-checked
peer-reviewed publication
trusted source
proofread
Researchers develop high-performance digital system for tailoring polymers
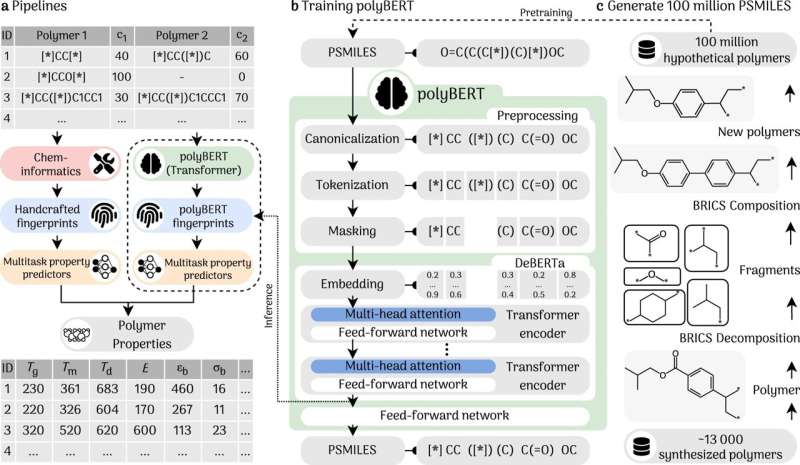
Polymers have become an indispensable part of everyday life. However, the current polymers represent only a small fraction of the huge number of polymers that theoretically exist.
Prof. Dr. Christopher Kuenneth at the University of Bayreuth, Germany, together with research partners in Atlanta, U.S., have now developed a digital system that promises extraordinarily high economical, technological and ecological benefits: from around 100 million theoretically possible polymers, their system can precisely select those materials that have an ideal property profile for targeted applications at unprecedented speed.
The new system is presented in Nature Communications.
Kuenneth, Professor of Computational Materials Science at the Faculty of Engineering at the University of Bayreuth, and Prof. Dr. Rampi Ramprasad at the Georgia Institute of Technology in Atlanta have named their new system "polyBERT." The name comes from the interdisciplinarity from which polyBERT emerged: insights, concepts and techniques of polymer chemistry, linguistics and natural language processing, and the new artificial intelligence paradigm.
polyBERT is a system that treats the chemical structure of polymers like a chemical language: each word that can be formed in this language is a unique name for a theoretically possible polymer. The molecular building blocks and structures of respective polymers are reflected in these names. Building on new insights from linguistics and computer science, polyBERT has been trained and developed to a learning system by the research team in Bayreuth and Atlanta.
From polymer language to digital 'fingerprints'
In a first step, polyBERT has learned the names of about 100 million theoretically possible polymers. These names are combinations of molecular units contained in approximately 13,000 polymers. The training of polyBERT makes it understand the polymer language, and correctly identify building blocks and structures of about 100 million polymers. The learning digital system can even use the polymer language on its own. This means that polyBERT can generate further names of previously unknown but theoretically possible polymers.
Linked to the chemical language expertise is another capability: polyBERT automatically translates polymer names that it knows into numerical representations, so-called "fingerprints." Each fingerprint is a unique code word consisting of numbers from which the building blocks and structure of the respective polymer can be inferred. This automatic generation of digital fingerprints is far less error-prone and much faster than human-generated fingerprints for each chemical structure of polymers.
Rapid and precise prediction of polymer properties
polyBERT derives its enormous practical relevance from the teaching process, by the researchers in Bayreuth and Atlanta, about numerous characteristic polymer properties that are particularly relevant for technological applications. The system is therefore able to unambiguously correlate fingerprints and properties of polymers.
Novel techniques from the field of artificial intelligence enable polyBERT to precisely select, with high accuracy and at unprecedented speed, those polymers required for specific applications from the 100 million theoretically possible polymers.
"polyBERT is an exceptionally high-performance system for rapid and accurate prediction of polymer properties. Therefore, our research has the potential to significantly accelerate the design, synthesis and technological application of polymers," says Kuenneth.
Past study identifies bioplastics
The importance of machine learning approaches to polymer research is already demonstrated by a past study that Kuenneth published in the journal Communications Materials in December 2022. Here, he and research partners at Atlanta and the Los Alamos National Laboratories in the United States present a similar artificial neural network-based system for predicting polymer properties.
This system is capable of countering global plastic waste pollution. About 75 percent of industrially produced plastics are based on fossil raw materials. The new system can significantly accelerate the search for biopolymers which can replace these plastics: The authors of the study identified 14 biologically producible and degradable polymers from 1.4 million possible candidates that can replace the current industrial plastics as soon as fast and cost-effective synthesis processes become available.
More information: Christopher Kuenneth et al, polyBERT: a chemical language model to enable fully machine-driven ultrafast polymer informatics, Nature Communications (2023). DOI: 10.1038/s41467-023-39868-6
Journal information: Nature Communications
Provided by Bayreuth University