April 2, 2021 feature
ProteinGAN: A generative adversarial network that generates functional protein sequences
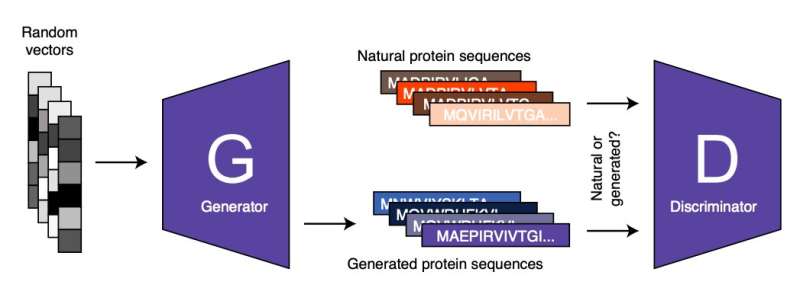
Proteins are large, highly complex and naturally occurring molecules can be found in all living organisms. These unique substances, which consist of amino acids joined together by peptide bonds to form long chains, can have a variety of functions and properties.
The specific order in which different amino acids are arranged to form a given protein ultimately determines the protein's 3D structure, physicochemical properties and molecular function. While scientists have been studying proteins for decades, designing proteins that elicit specific chemical reactions has so far proved to be highly challenging.
Researchers at Biomatter Designs, Vilnius University in Lithuania, and Chalmers University of Technology in Sweden have recently developed ProteinGAN, a generative adversarial network (GAN) that can process and 'learn' different natural protein sequences. This unique network, presented in a paper published in Nature Machine Intelligence, subsequently uses the information it acquired to generate new functional protein sequences.
"Proteins are long sequences of amino acids that make processes occur in all living systems, inducing humans," Aleksej Zelezniak, Associate professor at Chalmers University of Technology who led the study, told Phys.org. " Proteins are commonly used in our daily lives and are included in countless products, from washing powders to therapies against cancer and coronavirus. They are made of 20 amino acids that are arranged in different sequences and their order determines a protein's function."
Creating functional protein sequences is a very challenging task, as even a slight alteration in a given sequence can make a protein non-functional. Non-functional proteins can have harmful and undesirable effects, for instance causing humans or animals to develop cancer or other diseases.
"If one wants to make proteins aligned with human needs, he/she needs to correctly understand the order of amino acids and the given astronomical number of possibilities in making these proteins, which is not a trivial task," Zelezniak said. "Inspired by the latest developments in AI, particularly realistic photo and video generation, we were tempted to ask whether current AI technology is ready to produce the most complex molecules known to humans—proteins."
ProteinGAN, the model developed by Zelezniak and his colleagues is based on a renowned machine learning approach known as adversarial learning. Adversarial learning can be seen as a game 'played' by two or more artificial neural networks. The first of these networks, known as the 'generator' produces a specific type of data (e.g., an image, text, or in ProteinGAN's case a protein sequence). The second network, known as the 'discriminator," tries to distinguish between the artificial data (e.g., protein sequence) created by the 'generator' and authentic or real data.
Subsequently, the generator uses the feedback provided by the discriminator (i.e., the characteristics that allowed it to tell generated data apart from real data) to generate new data. The generator never processes or analyzes real data and the data it produces. Therefore, its learning relies solely on the outcome of the analyses carried out by the discriminator.
"By repeating this process iteratively both networks are getting better at what they do, until the generated sequences cannot be distinguished from the real ones," Zelezniak said. "Using the AI tool that we developed, we were able to generate functional proteins that were active but don't exist in nature or have not been yet discovered."
In initial trials run by the researchers, ProteinGAN generated new and highly diverse protein sequences with physical properties that resemble those of natural protein sequences. Using malate dehydrogenase (MDH) as a template enzyme, Zelezniak and his colleagues showed that many of the sequences generated by ProteinGAN are soluble and exhibit MDH catalytic activity, which means that they could have interesting applications in medical and research settings. In the future, ProteinGAN could be used to uncover new protein sequences with different properties, which may prove valuable for a variety of technological and scientific applications.
"Our research lab focuses on AI-based technologies for synthetic biology applications," Zelezniak said. "We are currently working on solving emerging problems such as plastic pollution and I believe AI will help to build better organisms that are suited for this particular problem."
More information: Expanding functional protein sequence spaces using generative adversarial networks. Nature Machine Intelligence(2021). DOI: 10.1038/s42256-021-00310-5.
Journal information: Nature Machine Intelligence
© 2021 Science X Network