Researchers move closer to completely optical artificial neural network
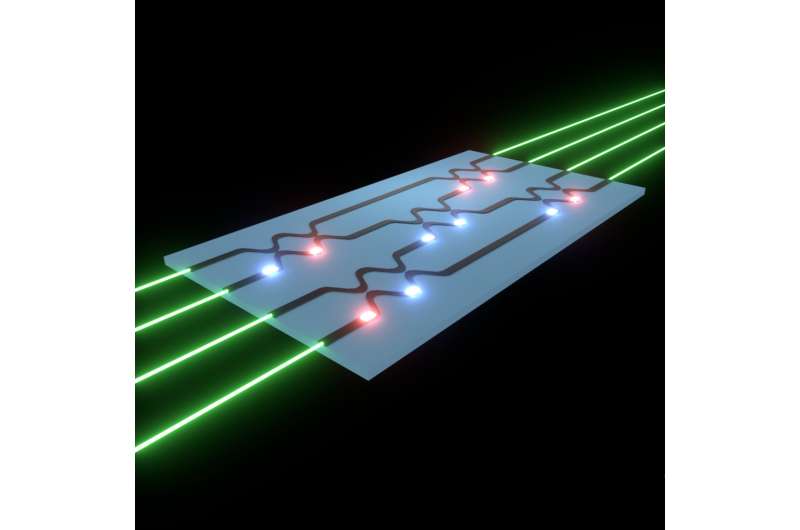
Researchers have shown that it is possible to train artificial neural networks directly on an optical chip. The significant breakthrough demonstrates that an optical circuit can perform a critical function of an electronics-based artificial neural network and could lead to less expensive, faster and more energy efficient ways to perform complex tasks such as speech or image recognition.
"Using an optical chip to perform neural network computations more efficiently than is possible with digital computers could allow more complex problems to be solved," said research team leader Shanhui Fan of Stanford University. "This would enhance the capability of artificial neural networks to perform tasks required for self-driving cars or to formulate an appropriate response to a spoken question, for example. It could also improve our lives in ways we can't imagine now."
An artificial neural network is a type of artificial intelligence that uses connected units to process information in a manner similar to the way the brain processes information. Using these networks to perform a complex task, for instance voice recognition, requires the critical step of training the algorithms to categorize inputs, such as different words.
Although optical artificial neural networks were recently demonstrated experimentally, the training step was performed using a model on a traditional digital computer and the final settings were then imported into the optical circuit. In Optica, The Optical Society's journal for high impact research, Stanford University researchers report a method for training these networks directly in the device by implementing an optical analogue of the 'backpropagation' algorithm, which is the standard way to train conventional neural networks.
"Using a physical device rather than a computer model for training makes the process more accurate," said Tyler W. Hughes, first author of the paper. "Also, because the training step is a very computationally expensive part of the implementation of the neural network, performing this step optically is key to improving the computational efficiency, speed and power consumption of artificial networks."
A light-based network
Although neural network processing is typically performed using a traditional computer, there are significant efforts to design hardware optimized specifically for neural network computing. Optics-based devices are of great interest because they can perform computations in parallel while using less energy than electronic devices.
In the new work, the researchers overcame a significant challenge to implementing an all-optical neural network by designing an optical chip that replicates the way that conventional computers train neural networks.
An artificial neural network can be thought of as a black box with a number of knobs. During the training step, these knobs are each turned a little and then the system is tested to see if the performance of the algorithms improved.
"Our method not only helps predict which direction to turn the knobs but also how much you should turn each knob to get you closer to the desired performance," said Hughes. "Our approach speeds up training significantly, especially for large networks, because we get information about each knob in parallel."
On-chip training
The new training protocol operates on optical circuits with tunable beam splitters that are adjusted by changing the settings of optical phase shifters. Laser beams encoding information to be processed are fired into the optical circuit and carried by optical waveguides through the beam splitters, which are adjusted like knobs to train the neural network algorithms.
In the new training protocol, the laser is first fed through the optical circuit. Upon exiting the device, the difference from the expected outcome is calculated. This information is then used to generate a new light signal, which is sent back through the optical network in the opposite direction. By measuring the optical intensity around each beam splitter during this process, the researchers showed how to detect, in parallel, how the neural network performance will change with respect to each beam splitter's setting. The phase shifter settings can be changed based on this information, and the process may be repeated until the neural network produces the desired outcome.
The researchers tested their training technique with optical simulations by teaching an algorithm to perform complicated functions, such as picking out complex features within a set of points. They found that the optical implementation performed similarly to a conventional computer.
"Our work demonstrates that you can use the laws of physics to implement computer science algorithms," said Fan. "By training these networks in the optical domain, it shows that optical neural network systems could be built to carry out certain functionalities using optics alone."
The researchers plan to further optimize the system and want to use it to implement a practical application of a neural network task. The general approach they designed could be used with various neural network architectures and for other applications such as reconfigurable optics.
More information: T. W. Hughes, M. Minkov, Y. Shi, S. Fan, "Training of photonic neural networks through in situ backpropagation and gradient measurement," Optica, Volume 5,Issue , pages 864-871 (2018) DOI: 10.1364/OPTICA.5.000864
Journal information: Optica
Provided by Optical Society of America