Deep learning extends imaging depth and speeds up hologram reconstruction
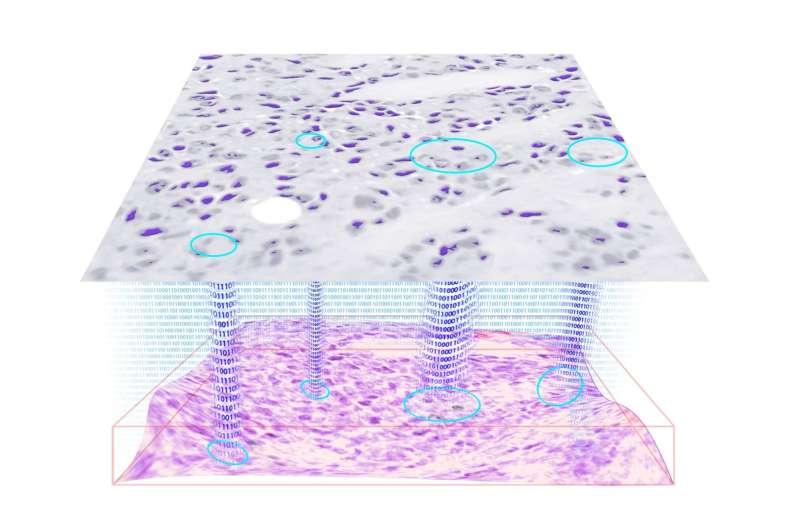
Deep learning, which uses multi-layered artificial neural networks, is a form of machine learning that has demonstrated significant advances in many fields, including natural language processing, image/video labeling and captioning. In image processing, deep learning demonstrates significant potential for automated identification and labeling of features of interest, such as abnormal regions in a medical image.
UCLA researchers have demonstrated an innovative application of deep learning to significantly extend the imaging depth of a hologram. In holography, image reconstruction requires performing autofocusing and phase recovery, which are in general cumbersome and time-consuming to perform over a large sample volume. In a recent article published in Optica, a journal of the Optical Society of America, UCLA researchers have demonstrated a new approach they termed HIDEF based on a convolutional neural network that simultaneously performs autofocusing and phase recovery to significantly extend the image depth of field and the reconstruction speed in holography.
This research was led by Dr. Aydogan Ozcan, the Chancellor's Professor of electrical and computer engineering at UCLA and an HHMI Professor with the Howard Hughes Medical Institute, along with Yichen Wu, a graduate student, and Dr. Yair Rivenson, a postdoctoral scholar, both at the UCLA electrical and computer engineering department.
The authors validated this deep learning based approach by successfully reconstructing holograms of aerosols and human tissue samples. Overall, this approach significantly boosts the computational efficiency and the reconstruction speed of high-resolution holographic imaging by simultaneously performing autofocusing and phase recovery, which also increases the robustness of the image reconstruction process to potential misalignments in the optical setup by extending the depth of the reconstructed images.
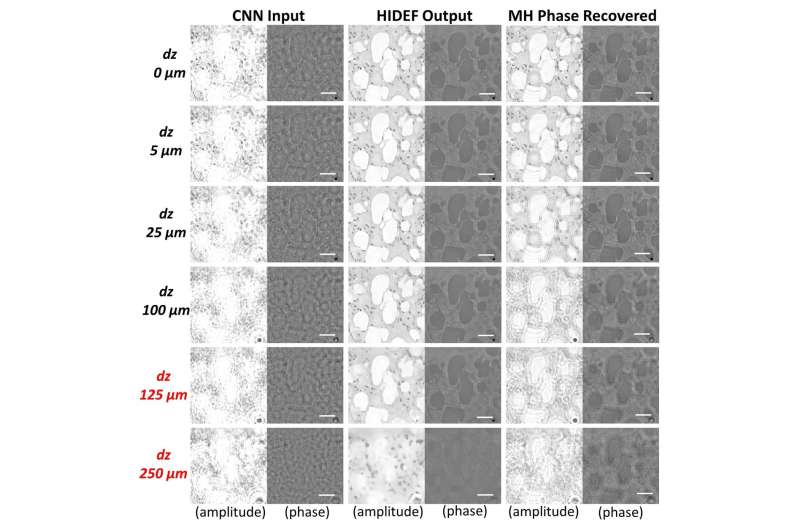
"Deep learning is mysteriously powerful and has been surprising optics researchers in what it can achieve for advancing optical microscopy, and introducing new image reconstruction methods. From physics-inspired optical designs/devices, we are moving toward data-driven designs that will holistically change both optical hardware and software of next generation microscopy, blending the two in new ways," added Ozcan.
Other members of the research team were Yibo Zhang, Zhensong Wei, Harun Günaydin and Xing Lin, members of the Ozcan Research Lab at UCLA.
More information: Yichen Wu et al. Extended depth-of-field in holographic imaging using deep-learning-based autofocusing and phase recovery, Optica (2018). DOI: 10.1364/OPTICA.5.000704
Journal information: Optica
Provided by UCLA Ozcan Research Group