Evolutionary lessons for wind farm efficiency
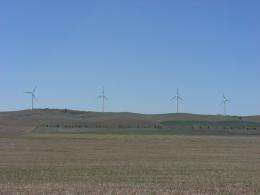
Evolution is providing the inspiration for University of Adelaide computer science research to find the best placement of turbines to increase wind farm productivity.
Senior Lecturer Dr Frank Neumann, from the School of Computer Science, is using a "selection of the fittest" step-by-step approach called "evolutionary algorithms" to optimise wind turbine placement. This takes into account wake effects, the minimum amount of land needed, wind factors and the complex aerodynamics of wind turbines.
"Renewable energy is playing an increasing role in the supply of energy worldwide and will help mitigate climate change," says Dr Neumann. "To further increase the productivity of wind farms, we need to exploit methods that help to optimise their performance."
Dr Neumann says the question of exactly where wind turbines should be placed to gain maximum efficiency is highly complex. "An evolutionary algorithm is a mathematical process where potential solutions keep being improved a step at a time until the optimum is reached," he says.
"You can think of it like parents producing a number of offspring, each with differing characteristics," he says. "As with evolution, each population or `set of solutions' from a new generation should get better. These solutions can be evaluated in parallel to speed up the computation."
Other biology-inspired algorithms to solve complex problems are based on ant colonies.
"Ant colony optimisation" uses the principle of ants finding the shortest way to a source of food from their nest.
"You can observe them in nature, they do it very efficiently communicating between each other using pheromone trails," says Dr Neumann. "After a certain amount of time, they will have found the best route to the food - problem solved. We can also solve human problems using the same principles through computer algorithms."
Dr Neumann has come to the University of Adelaide this year from Germany where he worked at the Max Planck Institute. He is working on wind turbine placement optimisation in collaboration with researchers at the Massachusetts Institute of Technology.
"Current approaches to solving this placement optimisation can only deal with a small number of turbines," Dr Neumann says. "We have demonstrated an accurate and efficient algorithm for as many as 1000 turbines."
The researchers are now looking to fine-tune the algorithms even further using different models of wake effect and complex aerodynamic factors.
Provided by University of Adelaide