This article has been reviewed according to Science X's editorial process and policies. Editors have highlighted the following attributes while ensuring the content's credibility:
fact-checked
proofread
From trash to treasure: Machine learning enhances organic waste recycling
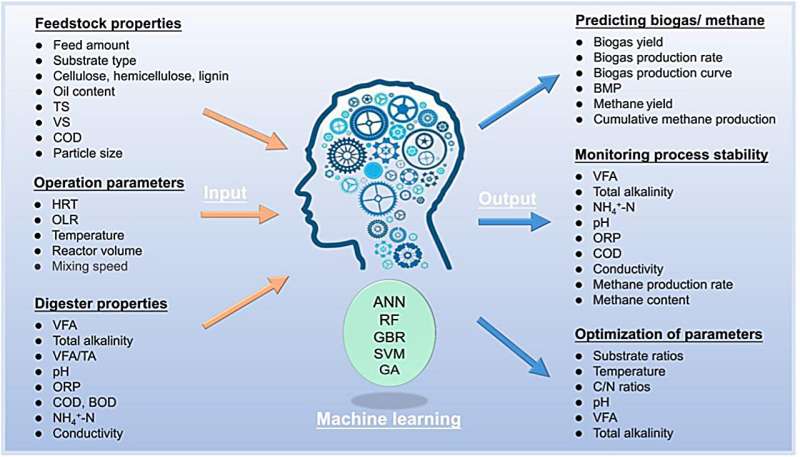
Biological treatment methods such as anaerobic digestion, composting, and insect farming are essential for managing organic waste, converting it into valuable resources like biogas and organic fertilizers. However, these processes often face challenges due to their inherent complexity and instability, which can affect efficiency and product quality.
Traditional control strategies have limited success in addressing these issues. Therefore, advanced methods like machine learning (ML) are being explored to enhance prediction, optimization, and monitoring of these biological treatments, aiming to improve overall performance and sustainability.
A research team from Tongji University published a review in Circular Economy that explores the application of ML in the biological treatment of organic wastes. The article, available online, delves into the effectiveness of various ML algorithms in optimizing processes such as anaerobic digestion, composting, and insect farming, aiming to enhance treatment efficiency and product quality.
This review provides an in-depth evaluation of ML applications in biological treatment processes, focusing on key algorithms such as artificial neural networks, tree-based models, support vector machines, and genetic algorithms. The research demonstrates how ML can accurately predict treatment outcomes, optimize process parameters, and enable real-time monitoring, significantly improving the efficiency and stability of processes like anaerobic digestion, composting, and insect farming.
For example, ML models have been successfully used to forecast biogas production, determine compost maturity, and optimize growth conditions in insect farming. Additionally, the study addresses the challenges faced in applying ML, including model selection, parameter adjustment, and the need for practical engineering validation. By overcoming these hurdles, ML has the potential to revolutionize biological waste treatment, making it more efficient, reliable, and sustainable.
Dr. Fan Lü, the corresponding author, emphasized, "ML offers unprecedented opportunities to enhance the efficiency and stability of biological treatment processes. By leveraging advanced algorithms, we can better predict and optimize these complex systems, ultimately contributing to more sustainable waste management solutions."
The application of ML in biological treatment holds significant potential for improving waste management practices. By optimizing processes and ensuring consistent product quality, ML can help reduce environmental impacts and enhance resource recovery.
Future research should focus on overcoming current challenges, such as improving model explainability and conducting practical engineering validations, to fully harness the potential of ML in this field.
More information: Long Chen et al, Applications of machine learning tools for biological treatment of organic wastes: Perspectives and challenges, Circular Economy (2024). DOI: 10.1016/j.cec.2024.100088
Provided by Tsinghua University Press