This article has been reviewed according to Science X's editorial process and policies. Editors have highlighted the following attributes while ensuring the content's credibility:
fact-checked
peer-reviewed publication
trusted source
proofread
A simple and robust experimental process for protein engineering
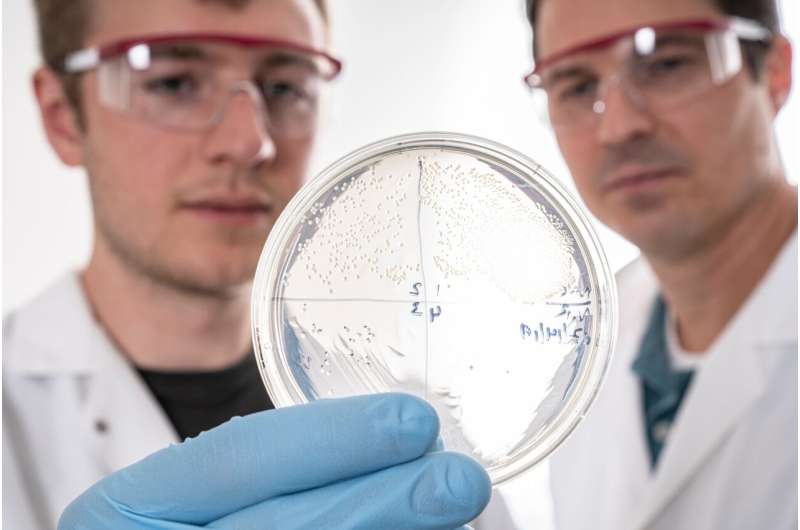
A protein engineering method using simple, cost-effective experiments and machine learning models can predict which proteins will be effective for a given purpose, according to a new study by University of Michigan researchers.
The method has far-reaching potential to assemble proteins and peptides for applications from industry tools to therapeutics. For instance, this technique can help speed up the development of stabilized peptides for treating diseases in ways that current medicines can't, including improving how exclusively antibodies bind to their targets in immunotherapy.
"The rules that govern how proteins work, from sequence to structure to function, are so complicated. Contributing to the interpretability of protein engineering efforts is particularly exciting," said Marshall Case, a doctoral graduate of chemical engineering at U-M and first author of the study.
Currently, most protein engineering experiments use complex, labor-intensive methods and expensive instruments to attain very precise data. The long process limits how much data can be acquired, and the complicated methods are challenging to learn and execute—a trade-off for precision.
"Our method has shown that for many applications, you can avoid these complicated methods," said Case, now a computational biologist at Manifold Biotechnologies.
The updated method starts by sorting cells into two groups, known as binary sorting, based on whether they express a desired trait—like binding to fluorescent molecules—or not. Then, the cells are sequenced to get the underlying DNA codes for the proteins of interest. Machine learning algorithms then reduce the noise in the sequencing data to identify the best possible protein.
"Rather than selecting the 'best book' from the library, it's like reading many books, then piecing together different pages from different stories to come up with the best book possible, even if it wasn't in your original library," said Greg Thurber, U-M associate professor of chemical engineering and corresponding author on the paper. "I was surprised to see the robustness of this technique using simple, binary sorting data."
Further enhancing its accessibility, the method uses linear machine learning models, which are easier to interpret compared to models with dozens of parameters.
"Because we can learn physical rules about how the proteins are actually working, we can use linear equations to model nonlinear protein behavior and make better drugs that way," Case said.
The research is published in the journal Proceedings of the National Academy of Sciences and was conducted at the Advanced Genomics Core, Center for Structural Biology, Biological Mass Spectrometry Facility and Proteomics & Peptide Synthesis Core.
More information: Marshall Case et al, Machine learning to predict continuous protein properties from binary cell sorting data and map unseen sequence space, Proceedings of the National Academy of Sciences (2024). DOI: 10.1073/pnas.2311726121
Journal information: Proceedings of the National Academy of Sciences
Provided by University of Michigan