This article has been reviewed according to Science X's editorial process and policies. Editors have highlighted the following attributes while ensuring the content's credibility:
fact-checked
proofread
A deep learning–based method for high-precision stomata detection and conductance analysis
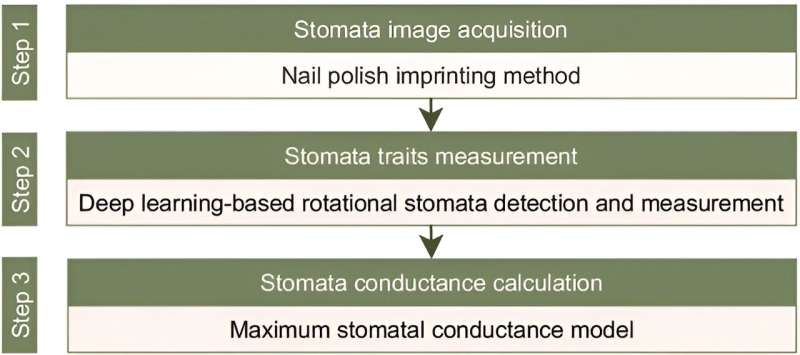
Stomata are vital for regulating water and carbon dioxide in plants, affecting photosynthesis. Traditional stomata analysis was manual and error-prone, but deep learning (DL) methods, such as DCNN, have been introduced for enhanced detection and measurement. However, these advanced techniques still face challenges in accurately calculating stomatal traits due to the random orientation of stomata, requiring additional image processing.
Plant Phenomics published a research article titled "Rotating Stomata Measurement Based on Anchor-Free Object Detection and Stomata Conductance Calculation."
This paper introduces DeepRSD, a deep learning-based method designed to detect rotating stomata and calculate their traits. By incorporating a stomata conductance loss function, DeepRSD achieves 94.3% accuracy for maize leaf stomata, enhancing detection and conductance calculation.
In this study, the method begins with image preprocessing, including geometric correction and resolution standardization. Manual labeling is vital for training, involving software like labelimg2 to define stomata with the smallest enclosing rectangles.
The training utilizes 2,192 maize leaf images, expanded to 24,112 through data augmentation. An AdamW optimization algorithm, coupled with specific learning rate adjustments and a GPU-accelerated software environment, facilitates efficient training.
Five distinct loss functions, including heatmap, width-height, offset, angular, and stomata conductance loss, are utilized to optimize the model's accuracy. The results demonstrate that DeepRSD significantly outperforms other models in precision, recall, and F1 score after 20 epochs. However, challenges remain, including missed detections and false positives attributed to leaf impurities or similar objects. Future improvements aim to enhance recognition accuracy.
In conclusion, the research asserts that DeepRSD's anchor-free detection and rapid calculation capabilities make it a valuable tool for large-scale analysis of stomata traits and conductance.
This method not only improves efficiency and accuracy but also provides a deeper understanding of stomata responses to environmental stressors, aiding research on crop yield and plant stress resistance. While currently focused on maize leaves, this approach has potential applications for other monocotyledons, offering a comprehensive tool for botanists in their research endeavors.
More information: Fan Zhang et al, Rotating Stomata Measurement Based on Anchor-Free Object Detection and Stomata Conductance Calculation, Plant Phenomics (2023). DOI: 10.34133/plantphenomics.0106
Provided by Plant Phenomics