This article has been reviewed according to Science X's editorial process and policies. Editors have highlighted the following attributes while ensuring the content's credibility:
fact-checked
trusted source
proofread
Few-shot learning transforms leaf trait analysis in bioenergy crop Populus trichocarpa
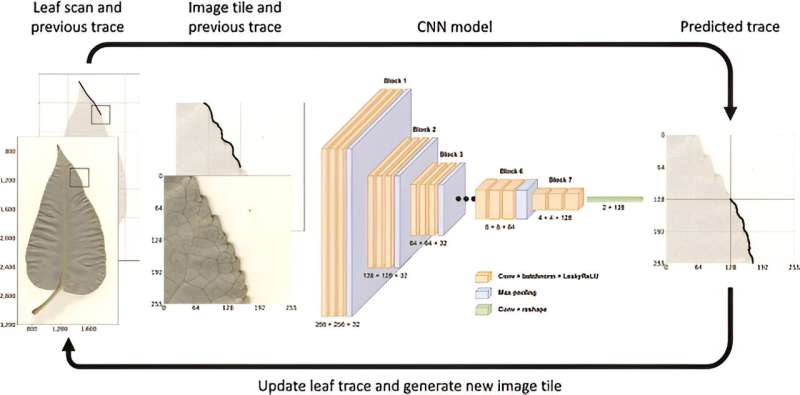
Image-based plant phenotyping has become crucial in understanding plant biology and agriculture. However, distinguishing relevant biological structures from the background remains a challenge, particularly in low-contrast situations. While deep learning has enhanced feature extraction in computer vision, its application in plant phenotyping is hindered by the need for extensive image annotation.
To address this, the current research explores few-shot learning, a subset of machine learning requiring fewer labeled samples. Poplar trees, particularly Populus trichocarpa, are significant for their genetic diversity and potential in bioenergy.
Traditional methods of leaf and vein segmentation in phenotyping have limitations, including time-consuming processes and the need for large annotated datasets. Therefore, utilizing few-shot learning to efficiently segment leaf images with minimal training data will be a critical step in advancing plant phenotyping research.
In July 2023, Plant Phenomics published a research article titled "Few-Shot Learning Enables Population-Scale Analysis of Leaf Traits in Populus trichocarpa."
In this study, few-shot learning combined with convolutional neural networks (CNNs) was employed to segment the leaf body and venation of 2,906 P. trichocarpa leaf images. The segmentation accuracy was remarkable, with the leaf tracing CNN and the baseline U-Net model showing high Jaccard scores, indicating a close match with ground truth segmentations.
Vein segmentation presented additional challenges due to the intricate nature of vein architecture and minor human errors in manual annotation; however, the vein growing framework still achieved high recall values. This underscores its effectiveness in capturing the complex vein structure accurately.
The number of connected components in each vein segmentation served as a proxy for biological accuracy, with the vein growing CNN outperforming U-Net in producing biologically realistic vein segmentations.
Real-world caliper measurements validated the digital measurements of leaf traits. Genomic analysis further leveraged the segmentation and feature extraction methods, revealing significant genetic influences on vein density. The identified genes and their Arabidopsis thaliana orthologs provided deeper insights into leaf development processes.
In conclusion, this study presents a robust and efficient workflow from image acquisition to phenotype extraction, greatly enhancing the understanding of plant genetics. This further allows researchers to assess how vein traits relate to other physiological processes, such as stomatal conductance, gas exchange, and overall plant productivity with important implications for developing Populus as a bioenergy crop.
Genes detected from the quantitative genetic analysis can be used in future biotechnology experiments for optimizing traits targeted for climate resilience, biomass production, and accelerated domestication for agriculture and biofuel production.
More information: John Lagergren et al, Few-Shot Learning Enables Population-Scale Analysis of Leaf Traits in Populus trichocarpa, Plant Phenomics (2023). DOI: 10.34133/plantphenomics.0072
Provided by NanJing Agricultural University