This article has been reviewed according to Science X's editorial process and policies. Editors have highlighted the following attributes while ensuring the content's credibility:
fact-checked
trusted source
proofread
Autonomous measuring instruments systematically detect new materials
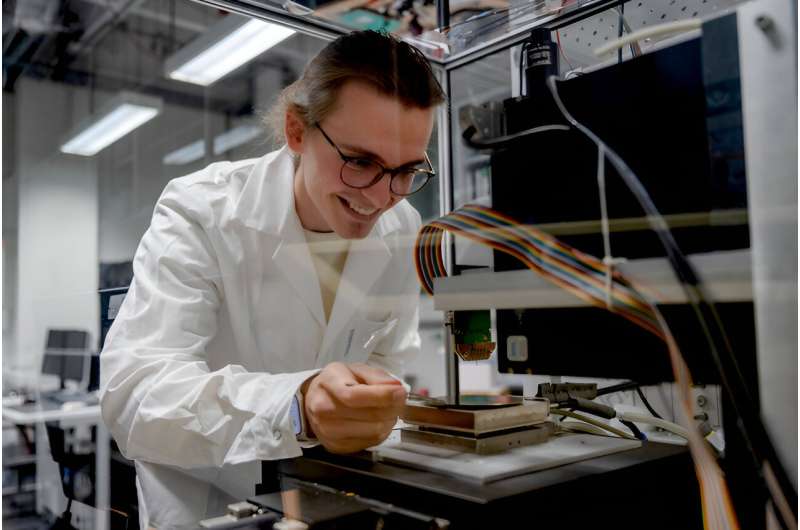
Researchers are working tirelessly to find new materials for future technologies that are essential for the energy transition—as electrocatalysts, for example. Due to their versatile properties, materials consisting of five or more elements are of great interest for this purpose. With approximately 50 usable elements of the periodic table, there is an almost infinite number of feasible materials.
Felix Thelen from the Chair of Materials Discovery and Interfaces at Ruhr University Bochum, headed by Professor Alfred Ludwig, has developed an algorithm that can scan material candidates four times faster than before. This is enabled by the concept of active learning, a subfield of machine learning. The research team published its findings in the journal Digital Discovery on 19 September 2023.
Despite highly specialized methods that can simultaneously produce a range of materials on a single sample and then measure them automatically, every minute counts when analyzing them: because days or even weeks can pass before the characterization of a sample is complete. The new algorithm can be integrated into existing measuring instruments to boost their efficiency significantly.
"Through active learning, a measuring instrument is able to independently select the next measurement area on a sample, based on the information already available about the material," explains Thelen, developer of the autonomous measurement algorithm.
In the background, a mathematical model of the measured material property is refined point by point until sufficient accuracy is achieved. At one point, the measurement can be stopped—and the results at the remaining measurement areas will be predicted by the generated model.
By analyzing 10 materials libraries using electrical resistance measurements, the Bochum research team demonstrated how the algorithm works. "Our work is only just beginning at this point," says Thelen. "This is because in materials research there are far more complex measurement methods than resistance measurement, which also need to be optimized."
In cooperation with the manufacturers of the instruments, solutions must now be developed that enable the integration of such active learning algorithms.
More information: Felix Thelen et al, Speeding up high-throughput characterization of materials libraries by active learning: autonomous electrical resistance measurements, Digital Discovery (2023). DOI: 10.1039/D3DD00125C
Provided by Ruhr-Universitaet-Bochum