Artificial intelligence sets sights on the sun
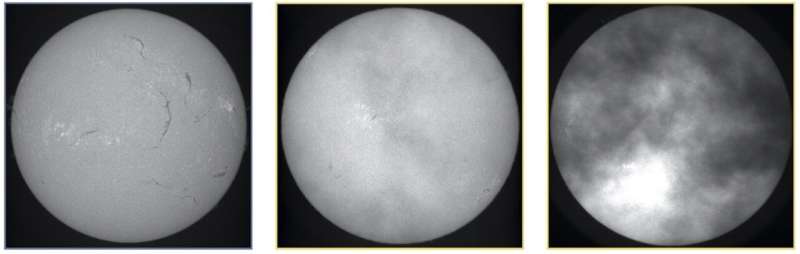
Scientists from the University of Graz and the Kanzelhöhe Solar Observatory (Austria) and their colleagues from the Skolkovo Institute of Science and Technology (Skoltech) developed a new method based on deep learning for stable classification and quantification of image quality in ground-based full-disk solar images. The research results were published in the journal Astronomy & Astrophysics and are available via open access.
The Sun is the only star where we can discern surface details and study plasma under extreme conditions. The solar surface and atmospheric layers are strongly influenced by the emerging magnetic field. Features such as sunspots, filaments, coronal loops, and plage regions are a direct consequence of the distribution of enhanced magnetic fields on the Sun, which challenges our current understanding of these phenomena. Solar flares and coronal mass ejections result from a sudden release of free magnetic energy stored in the strong fields associated with sunspots. They are the most energetic events in our solar system and have a direct impact on the Sun-Earth system called 'space weather.' Modern society strongly relies on space and ground-based technology which is highly vulnerable to hazardous space weather events. Continuous monitoring of the Sun is essential for better understanding and predicting solar phenomena and the interaction of solar eruptions with the Earth's magnetosphere and atmosphere. In recent decades, solar physics has entered the era of big data, and the large amounts of data constantly produced by ground- and space-based observatories can no longer be analyzed by human observers alone.
Ground-based telescopes are positioned around the globe to provide continuous monitoring of the Sun independently of the day-night schedule and local weather conditions. Earth's atmosphere imposes the strongest limitations on solar observations since clouds can occult the solar disk and air fluctuations can cause image blurring. In order to select the best images from multiple simultaneous observations and detect local quality degradations, objective image quality assessment is required.
"As humans, we assess the quality of a real image by comparing it to an ideal reference image of the Sun. For instance, an image with a cloud in front of the solar disk—a major deviation from our imaginary perfect image—would be tagged as a very low-quality image, while minor fluctuations are not that critical when it comes to quality. Conventional quality metrics struggle to provide a quality score independent of solar features and typically do not account for clouds," says Tatiana Podladchikova, an assistant professor at the Skoltech Space Center (SSC) and a research co-author.
In their recent study, the researchers used artificial intelligence (AI) to achieve quality assessment that is similar to human interpretation. They employed a neural network to learn the characteristics of high-quality images and estimate the deviation of real observations from an ideal reference.
The paper describes an approach based on Generative Adversarial Networks (GAN) that are commonly used to obtain synthetic images, for example, to generate realistic human faces or translate street maps into satellite images. This is achieved by approximating the distribution of real images and picking samples from it. The content of the generated image can be either random or defined by a conditional description of the image. The scientists used the GAN to generate high-quality images from the content description of the same image: the network first extracted the important characteristics of the high-quality image, such as the position and appearance of solar features, and then generated the original image from this compressed description. When this procedure is applied to lower quality images, the network re-encodes the image content, while omitting low-quality features in the reconstructed image. This is a consequence of the approximated image distribution by the GAN which can only generate images of high quality. The difference between a low-quality image and the envisioned high-quality reference of the neural network provides the basis for an image quality metric and is used to identify the position of quality degrading effects in the image.
"In our study, we applied the method to observations from the Kanzelhöhe Observatory for Solar and Environmental Research and showed that it agrees with human observations in 98.5% of cases. From the application to unfiltered full observing days, we found that the neural network correctly identifies all strong quality degradations and allows us to select the best images, which results in a more reliable observation series. This is also important for future network telescopes, where observations from multiple sites need to be filtered and combined in real-time," says Robert Jarolim, a research scientist at the University of Graz and the first author of the study.
"In the 17th century, Galileo Galilei was the first to dare look at the Sun through his telescope, while in the 21st century, dozens of space and ground observatories continuously track the Sun, providing us with a wealth of solar data. With the launch of the Solar Dynamics Observatory (SDO) 10 years ago, the amount of solar data and images transmitted to Earth soared to 1.5 terabytes per day, which is equivalent to downloading half a million songs daily. The Daniel K. Inouye Solar Telescope, the world's largest ground-based solar telescope with a 4-meter aperture, took the first detailed images of the Sun in December 2019 and is expected to provide six petabytes of data per year. Solar data delivery is the biggest project of our times in terms of total information produced. With the recent launches of groundbreaking solar missions, Parker Solar Probe and Solar Orbiter, we will be getting ever-increasing amounts of data offering new valuable insights. There are no beaten paths in our research. With so much new information coming in daily, we simply must invent novel efficient AI-aided data processing methods to deal with the biggest challenges facing humankind. And whatever storms may rage, we wish everyone good weather in space," Podladchikova says.
The new method was developed with the support of Skoltech's high-performance cluster for the anticipated Solar Physics Research Integrated Network Group (SPRING) that will provide autonomous monitoring of the Sun using cutting-edge technology of observational solar physics. SPRING is pursued within the SOLARNET project, which is dedicated to the European Solar Telescope (EST) initiative supported by the EU research and innovation funding program Horizon 2020. Skoltech represents Russia in the SOLARNET consortium of 35 international partners.
Currently, the authors are further elaborating their image processing methods to provide a continuous data stream of the highest possible quality and developing automated detection software for continuous tracking of solar activity.
More information: R. Jarolim et al, Image-quality assessment for full-disk solar observations with generative adversarial networks, Astronomy & Astrophysics (2020). DOI: 10.1051/0004-6361/202038691
Journal information: Astronomy & Astrophysics
Provided by Skolkovo Institute of Science and Technology