Artificial intelligence sheds new light on cell developmental dynamics
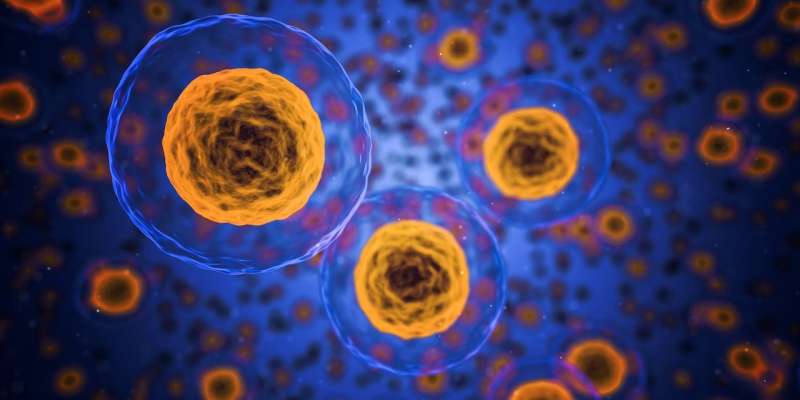
What happens inside a cell when it is activated, changing, or responding to variations in its environment? Researchers from the VIB-UGent Center for Inflammation Research have developed a method to best model these cellular dynamics. Their work not only highlights the outstanding challenges of tracking cells throughout their growth and lifetime, but also pioneers new ways of evaluating computational biology methods that aim to do this.
Identifying the trajectories of individual cells
Cells are constantly changing: They divide, change, or are activated by the environment. Cells can take many alternative paths in each of these processes and they have to decide which direction to follow based on internal and external clues. Studying these cellular trajectories has recently become a lot easier thanks to advances in single-cell technologies, which allows scientists to profile individual cells at unprecedented detail. Combined with computational methods, it is possible to see the different trajectories that cells take inside a living organism and have a closer look at what goes wrong in diseases.
Yvan Saeys (VIB-Ghent University), heading the research group, says, "If you would take a random sample of thousands of cells that are changing, you would see that some are very similar, while others are really different. Trajectory inference methods are a novel class of Artificial Intelligence techniques that unveil complex structures such as cell trajectories in a data-driven way. In recent years there has been a proliferation of tools that construct such a trajectory. But the availability of a wide variety of such tools makes it very difficult for researchers to find the right one that will work in the biological system they are studying."
Evaluating the available tools
Two researchers in the Saeys lab, Robrecht Cannoodt and Wouter Saelens, set out to bring more clarity to the field by evaluating and comparing the available tools. Robrecht Cannoodt says: "From the start, we envisioned to make the benchmark as comprehensive as possible by including almost all methods, a varied set of datasets and metrics. We included the nitty-gritty details, such as the installation procedure, and put everything together in one large figureāa funky heatmap as we like to call it."
Wouter Saelens adds: "Apart from improving the trajectory inference field, we also attempted to improve the way benchmarking is done. In our study we ensured an easily reproducible and extensible benchmarking using the most recent software technologies such as containerization and continuous integration. In that way, our benchmarking study is not the final product, but only the beginning of accelerated software development and ultimately better understanding of our biomedical data."
User guidelines
Based on the benchmarking results, the team developed a set of user guidelines that can assist researchers in selecting the most suitable method for a specific research question, as well as an interactive app. This is the first comprehensive assessment of trajectory inference methods. In the future, the team plans to add a detailed parameter tuning procedure. The pipeline and tools for creating trajectories are freely available on dynverse.org, and the team welcomes discussion aimed at further development.
The results are reported in Nature Biotechnology.
More information: A comparison of single-cell trajectory inference methods, Nature Biotechnology (2019). DOI: 10.1038/s41587-019-0071-9 , www.nature.com/articles/s41587-019-0071-9
Journal information: Nature Biotechnology
Provided by VIB (the Flanders Institute for Biotechnology)