Computing solutions for biological problems
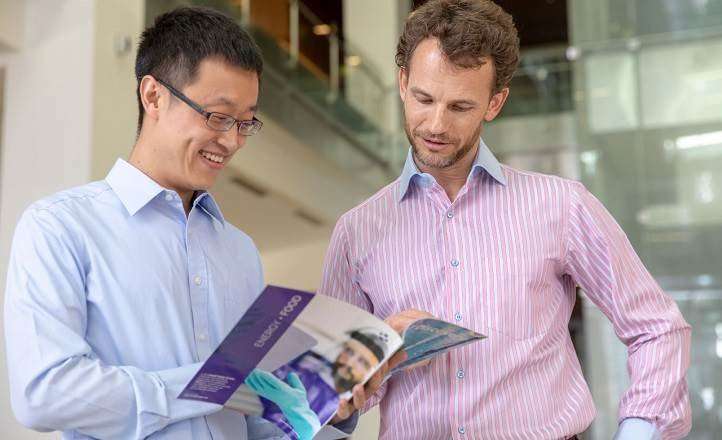
Producing research outputs that have computational novelty and contributions, as well as biological importance and impacts, is a key motivator for computer scientist Xin Gao. His Group at KAUST has experienced a recent explosion in their publications. Since January 1, 2018, they have produced 27 papers, including 11 published in the top three computational biology journals and seven presented at the top artificial intelligence and bioinformatics conferences.
Originally from China, Gao joined KAUST in 2010 after a stint with the University of Waterloo in Canada and a prestigious fellowship at Carnegie Mellon University in U.S. His group collaborates closely with experimental scientists to develop novel computational methods to solve key open problems in biology and medicine, he explains. "We work on building computational models, developing machine-learning techniques, and designing efficient and effective algorithms. Our focus ranges from analyzing protein amino acid sequences to determining their 3-D structures to annotating their functions and understanding and controlling their behaviors in complex biological networks," he says.
Gao describes one third of his lab's research as methodology driven, where the group develops theories and designs algorithms and machine-learning techniques. The other two-thirds is driven by problems and data. One example of his methodology-driven research is work1on improving non-negative matrix factorization (NMF), a dimension-reduction and data-representation tool formed of a group of algorithms that decompose a complex dataset expressed in the form of a matrix.
NMF is used to analyze samples where there are many features that might not all be important for the purpose of study. It breaks down the data to display patterns that can indicate importance. Gao's team improved on NMF by developing max-min distance NMF (MMDNMF), which runs through a very large amount of data to be able to highlight the high-order features that describe a sample more efficiently.
To demonstrate their approach, Gao's team applied the technique to human faces, using the images of 11 people with different expressions. Each image was treated as a sample with 1,024 features. After training MMDNMF to derive data to represent the features of each face, it could more correctly assign any black-and-white facial image than could be done using traditional NMF.
Opening biology's Pandora's box
Gao has many successful collaborations with KAUST researchers, but he says one of the most successful is with structural biologist, Stefan Arold.
Together, they have worked on several projects, including one that has led to a computational pipeline that can help pharmaceutical companies discover new protein targets for existing, approved drugs.
"Drug repositioning is commercially and scientifically valuable," explains Gao. "It can reduce the time needed for drug development from twenty to 6 years, and the costs from around 2 billion USD to 300 million USD. The National Institutes of Health in the United States estimates that 70 percent of drugs on the market can potentially be repositioned for use in other diseases."
Gao discovered that methods for drug repositioning face several challenges: they rely on very limited amounts of information and usually focus on a single drug or disease, leading to results that aren't statistically meaningful.
However, Gao's computational pipeline can integrate multiple sources of information on existing drugs and their known protein targets to help researchers discover new targets.
The model was tested for its ability to predict targets for a number of drugs and small molecules, including a known metabolite in the body called coenzyme A (CoA), which is important in many biological reactions, including the synthesis and oxidation of fatty acids. It predicted 10 previously unknown protein targets for CoA. Gao chose the top two: Arold and his colleagues then tested to see if they really did interact with CoA.
The collaboration verified Gao's predictions, and the computational pipeline is now being patented in several countries. It could eventually be licensed to pharmaceutical companies to enable already-approved drugs to be used for treating other diseases. The method can also help drug companies understand the molecular basis for drug toxicities and side effects.
"What makes our collaboration so synergistic is that our areas of expertise provide the minimal overlap needed to understand each other without creating redundancy," says Arold. "He brings the computational side and I bring the experimental side to the table. Our worlds touch, but don't overlap. Our discussions complement each other in a very stimulating way, without stumbling over too many semantic hurdles."
Another collaboration of Gao and Arold's involves enhancing the analysis of data gathered by electron microscopy. Arold explains that despite much progress in electron microscopy hardware and software—allowing it to be used to determine the 3-D structures of proteins and other biomolecules—the analysis of its data still needs to be improved. Gao and Arold are developing methods to reduce noise and thus improve the resolution of electron microscopic images of complex biomolecular particles.
They are also developing processes that can automate the interpretation of genetic variants and that enhance the process of assigning functions to genes. "If you put us together in a room for more than 15 minutes, we will probably come up with a new idea!" says Arold.
Improving current technologies
Other research by Gao's team includes a computational approach that can simulate a genetic sequencing technology called Nanopore sequencing. Gao's DeepSimulator3can evaluate newly developed downstream software in nanopore sequencing. It can also save time and resources through experimental simulations, reducing the need for real experiments.
His team also recently developed Gracob4, a method used to sift through genetic information and determine what pathways are turned on in microorganisms by stressful conditions, such as changes in acidity or temperature or exposure to antibiotics. This can identify genes that are dispensable under normal conditions but essential when the microorganism is stressed.
More information: Majed Alzahrani et al. Gracob: a novel graph-based constant-column biclustering method for mining growth phenotype data, Bioinformatics (2017). DOI: 10.1093/bioinformatics/btx199
Journal information: Bioinformatics