Paying for "extras" in freemium products and services
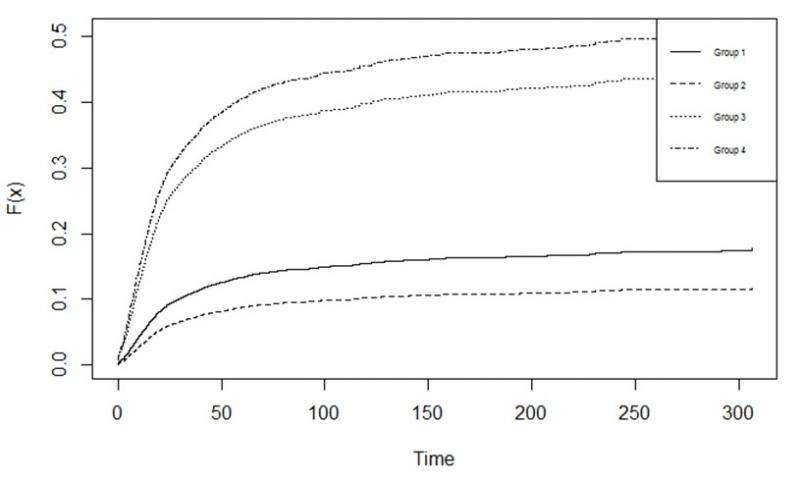
NUS statisticians have developed a better methodology to study user behaviour for freemium products and services.
Freemium refers to a business strategy where a basic version of a product or service is provided for free to the user. If a user wishes to have more advanced features or functionalities, these have to be purchased. Businesses are interested to know when a user gets sufficiently interested in the product or service to be willing to pay for them. They can make use of this information to refine and make better decisions on their marketing strategy. NUS researchers have developed a better way to analyse user behaviour for such schemes, particularly for the online gaming industry.
Prof LI Jialiang and his Ph.D. student CHEN Dacheng from the Department of Statistics and Applied Probability, NUS, have developed a competing risks approach to analyse customer behaviours in freemium products and services. This new approach is built on an existing model (Fine-Gray regression model). They are particularly interested in understanding when a user decides to start making payment for the additional features or functionalities that come with a product or service. If a user stops using the product before making payment, such an event will not happen. They have used this new model to analyse data from the online activities of online gaming players to understand the various factors involved in their decision-making process. These factors are hypothesised to have different effects at multiple change points, which determine the probability in which a player will be willing to pay for the additional features in the online game. This new methodology can be applied to analyse similar marketing strategies in other industry settings.
The worldwide online gaming industry has developed rapidly over the past decades. Industry sales worldwide were US$9 billion in 2009 and almost doubled in three years. Over the years, many different types of revenue models appeared (e.g. entrance purchase, periodic subscription, game token purchase, etc). The free-to-play coupled with the in-game (token) purchase model (freemium model) has become the dominant revenue model for this industry. This freemium model allows game players to play for free. Players can opt to purchase game tokens in the form of virtual coins or game points to enhance their gaming experience. It is estimated that about 75% of total sales from the online gaming industry were generated from the freemium revenue model. The research team has modelled the paying behaviour of customers in online games to better understand this type of freemium revenue model.
In the analysis, the researchers paid special attention to the point when a player starts to pay in the game (known as the "paying hazard"). However, a player may quit the game before he or she starts to pay in the game (known as the "quitting hazard"). The new methodology takes into account these two competing hazards which affect players' online behaviour. It also made provisions for cases when a player's paying hazard in a freemium product is affected by threshold effects to have a more realistic modelling of the player's behaviour.
Prof Li said, "The estimated effects of the factors allow business decision-makers to gain a better understanding of the impact of various aspects of customer behaviours and how they contribute to the 'paying hazard,' which is the most crucial element for the long-term feasibility of a product. This allows for more meaningful and informed business decisions."
More information: Dacheng Chen et al. Hazards regression for freemium products and services: a competing risks approach, Journal of Statistical Computation and Simulation (2017). DOI: 10.1080/00949655.2017.1292275
Jason P. Fine et al. A Proportional Hazards Model for the Subdistribution of a Competing Risk, Journal of the American Statistical Association (2006). DOI: 10.2307/2670170
Li J; Ma S, "Survival analysis in medicine and genetics" New York: Chapman and Hall Published: 2013.
Provided by National University of Singapore