This computer program can find new chemical combos to kill pathogenic yeast
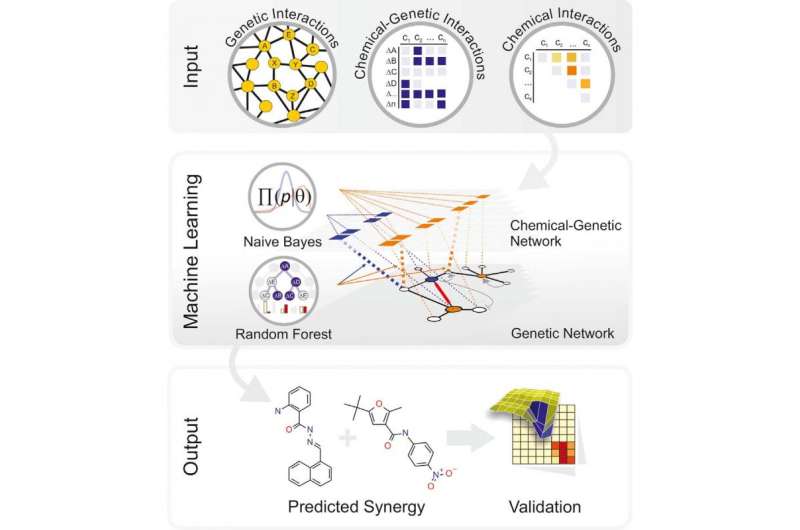
The drugs of tomorrow may be discovered by computers. A proof-of-concept study published December 23 in Cell Systems demonstrates that with the right input of data about infectious yeast, a machine algorithm can learn to identify combinations of existing and previously unknown compounds that can work together as antifungal agents. While the method needs to be perfected, it's a new approach to combat infectious disease with the potential to rapidly identify combinations of agents that might help overcome drug resistance.
We don't yet know how well the newly discovered chemical combos will work to treat yeast infections in animals or humans, but the research team did select 18 potential combinations to treat human pathogenic yeast in laboratory dishes and their high success rates confirms that the combinations have potential for drug development. Notably, some compound combinations were found to be harmless when applied to human cell lines.
"Our study illustrates the power of using relatively simple (but still extremely complicated) models such as yeast to better understand how chemicals and drugs interact with biological systems," says senior author Mike Tyers, a professor of systems biology at Université de Montréal. "These concepts will certainly be transferrable to more complex problems in human health."
Teaching the Machine
Many areas of research now use machine learning to find patterns in complex datasets; for example, in pattern recognition of images on the web or in robotic control systems.
"This trend has recently exploded in the biosciences, where increasingly machine learning is used to help researchers to make sense of enormous genome-scale datasets," says co-first author Jan Wildenhain, a systems developer at the University of Edinburgh. "The amount of biological data has simply become too large and complex to be processed by human intuition alone."
The researchers' first attempt at a machine learning algorithm was made with brewer's yeast (S. cerevisiae), because it is the only yeast that has had its genetic network mapped out. So although drug-resistant bacteria are the current prevailing public health concern, the model yeast system provides a larger and more informative dataset for this type of study.
The investigators began by inputting genetic information derived from decades of yeast research (namely, a selected set of 195 genetically different strains) and the genetic responses of these strains in chemical screens (using a diverse set of 4,915 compounds) so that the computer could build models for chemical-gene interactions. This was not enough information, however, and the initial algorithm had weak predictive power.
"This was a huge initial disappointment that sent us back to the drawing board," says co-first author Michaela Spitzer, now a postdoctoral fellow at McMaster University. "We knew that chemical structures and the genetic network of cells had to be related to chemical synergisms we detected experimentally, but how to deconvolve these relationships from hundreds of thousands of data points was not obvious. We ultimately had to revise our models several times based on training datasets and then test the models on different compound libraries that the model had never encountered before."
The ChemGRID Resource
The algorithms were trained on a set of 1,221 unique compounds that were used to create and experimentally test 8,128 actual combinations. All of the study data is available for unrestricted download and exploration on a database dubbed ChemGRID.
"We hope that other groups will test our models, as we will certainly continue to do, and that perhaps someone will come up with even better models," Spitzer and Wildenhain say. "It would be great to apply our machine learning approach to completely different datasets for synergy prediction."
In addition to collaborating with other labs, the group plans to implement a similar machine learning approach for chemical-gene interactions in human cells using CRISPR/Cas9 gene editing technology. By collecting this data, they could potentially create an algorithm that predicts chemical combinations that discriminate between healthy cells and unhealthy, for example, cancerous, cells.
More information: Cell Systems, Wildenhain and Spitzer et al: "Prediction of Synergism from Chemical-Genetic Interactions by Machine Learning" dx.doi.org/10.1016/j.cels.2015.12.003
Provided by Cell Press