This article has been reviewed according to Science X's editorial process and policies. Editors have highlighted the following attributes while ensuring the content's credibility:
fact-checked
trusted source
proofread
An AI model to reduce uncertainty in evapotranspiration prediction
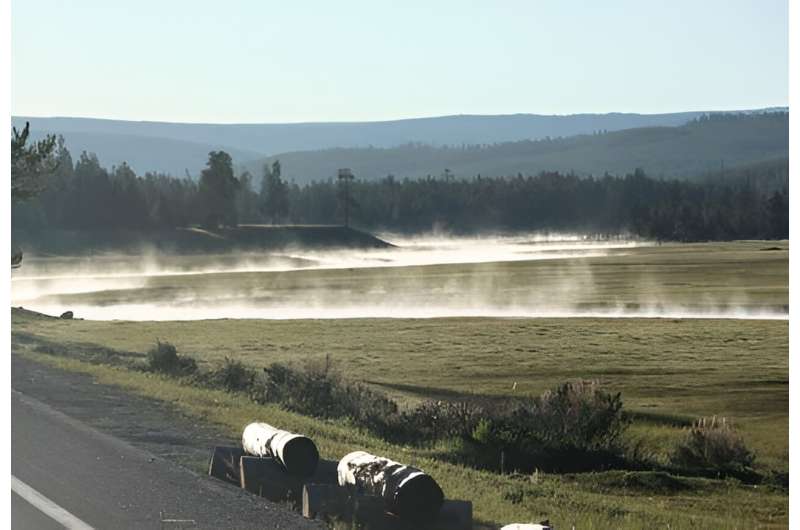
When scientists look at the Earth's available water for ecosystem services, they don't just look at precipitation. They must also account for water moving from the ground to the atmosphere, a process known as evapotranspiration (ET).
ET includes evaporation from soil and open water pools such as lakes, rivers, and ponds, as well as transpiration from plant leaves. The difference between precipitation and ET indicates the water balance available for societal needs, including agricultural and industrial production. However, measuring ET is challenging. A new study from the University of Illinois Urbana-Champaign presents a computer model that uses artificial intelligence (AI) for ET prediction based on remote sensing estimates.
"Ground-based ET estimates capture the local fluxes of water transferred to the atmosphere but are limited in scale. In contrast, satellite data provide ET information on a global scale. Still, they are often incomplete due to clouds or sensor malfunction, and the satellite cycle over an area may require several days."
"We conducted this research to predict missing data and to generate daily continuous ET data that accounts for the dynamics of land use and atmospheric air movement," said lead author Jeongho Han, a doctoral student in the Department of Agricultural and Biological Engineering (ABE), part of the College of Agricultural, Consumer and Environmental Sciences and The Grainger College of Engineering at Illinois.
The researchers created the "Dynamic Land Cover Evapotranspiration Model Algorithm" (DyLEMa) based on decision-tree machine learning models. This algorithm is intended to predict missing spatial and temporal ET data using trained seasonal machine learning models. DyLEMa was evaluated to the scale of Illinois on a daily 30 x 30-meter grid for 20 years using data from NASA, the U.S. Geological Survey, and the National Oceanic and Atmospheric Administration.
"DyLEMa is much more detailed and complex than other models. It distinguishes between different land uses, including forest, urban, and agriculture, and different crops, such as corn and soybean. The model includes precipitation, temperature, humidity, solar radiation, vegetation stage, and soil properties."
"This allows us to capture the surface dynamics accurately and predict ET based on multiple variables. This is especially important for agricultural landscapes where crops change rapidly," said co-author Jorge Guzman, research assistant professor in ABE.
The researchers tested the model's accuracy by comparing its results with existing data. For validation over time, they used ground measurements from 2009 to 2016 at four sites in Illinois. Also, to test spatial accuracy, they created artificial scenarios where they inserted a synthetic cloud in a cloud-free image, then applied their algorithm and compared the results with the original data.
Overall, DyLEMA reduced ET prediction uncertainty in cumulated ET estimates from an average of +30% (overpredicted) to around -7% (underpredicted) compared to existing measurements, indicating much greater accuracy.
The study is part of a larger project on soil erosion. Maria Chu, an associate professor in ABE, is the principal investigator on that project and co-author of the new paper.
"ET controls the soil moisture content and vice versa, which impacts surface processes such as runoff and water erosion. Our next step is to integrate our data in a distributed hydrological model for better estimation of soil erosion," Chu said.
"One of the challenges with land management practices is that people may not see the benefit of implementing changes right away. But with this model, we can show that what you are doing now will have a long-term impact, for instance, 10 or 20 years from now and at locations far from your farm. This is the power of using data and computing capacity for engaging communities and informing policy measures," Chu added.
The research is published in the journal Computers and Electronics in Agriculture.
More information: Jeongho Han et al, Dynamic land cover evapotranspiration model algorithm: DyLEMa, Computers and Electronics in Agriculture (2024). DOI: 10.1016/j.compag.2024.108875
Provided by University of Illinois at Urbana-Champaign