This article has been reviewed according to Science X's editorial process and policies. Editors have highlighted the following attributes while ensuring the content's credibility:
fact-checked
peer-reviewed publication
trusted source
proofread
Scientists create AI model that rivals top methods for weather and climate forecasts
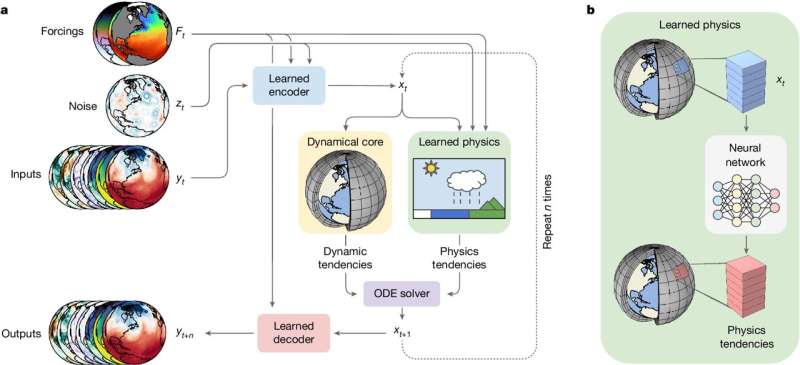
A machine learning model capable of both accurate weather predictions and climate simulations was published in Nature this week. The model, named NeuralGCM, outperforms some existing weather and climate prediction models and has the potential to make large savings in computational power over conventional models.
General circulation models (GCMs), representing physical processes of the atmosphere, ocean and land, are the basis for weather and climate predictions. Reducing the uncertainty around long-term forecasting and estimating extreme weather events are key to helping understand climate mitigation and adaptation.
Machine learning models have been suggested as an alternative approach to weather prediction with the benefit of reduced computational costs, but they often do not perform as well as GCMs when it comes to long-term forecasting.
Stephan Hoyer and colleagues designed NeuralGCM, a model that combines machine learning and physics-based methods, which can make short- and medium-range weather forecasts as well as simulate climate over a number of decades.
The model can compete with the accuracy of the European Center for Medium-Range Weather Forecasts (ECMWF, one of the best conventional physics-based weather models) predictions for 1- to 15-day forecasts. For forecasts up to 10 days in advance, NeuralGCM competes with and sometimes exceeds the accuracy of existing machine learning approaches.
NeuralGCM produces climate simulations at the same level of accuracy as the best machine learning and physics-based methods. When the authors included sea surface temperatures in 40-year climate predictions using NeuralGCM, they found that the outcomes the model produced mapped with the global warming trends seen in data from the ECMWF.
NeuralGCM also outperformed pre-existing climate models in predicting cyclones and their trajectories. Together, these findings suggest that machine learning is a viable approach for improving GCMs, the authors conclude.
More information: Dmitrii Kochkov et al, Neural general circulation models for weather and climate, Nature (2024). DOI: 10.1038/s41586-024-07744-y
Journal information: Nature
Provided by Nature Publishing Group