This article has been reviewed according to Science X's editorial process and policies. Editors have highlighted the following attributes while ensuring the content's credibility:
fact-checked
trusted source
proofread
Dual-branch network enhances plant disease detection for enhanced crop protection
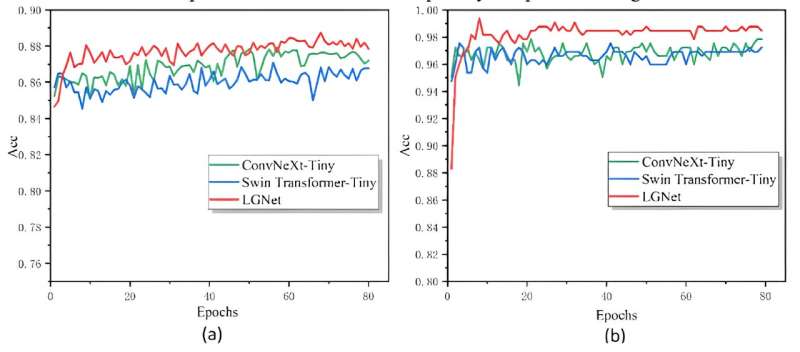
A research team has developed LGNet, a dual-branch network that combines convolutional neural networks (CNNs) and visual transformers (VTs) for plant disease identification. LGNet effectively fuses local and global features, achieving state-of-the-art recognition accuracies of 88.74% on the AI Challenger 2018 dataset and 99.08% on the self-collected corn disease dataset.
This innovative approach enhances disease sensing capabilities and offers the potential for the development of efficient and robust plant disease recognition models, which are crucial for improving agricultural production and ensuring crop safety in diverse environments.
Safeguarding agricultural production is vital for economic growth, as plant diseases significantly threaten crop yields. The traditional methods of identifying plant diseases, which rely on the farmers' experience, are time-consuming and inadequate for large-scale cultivation.
Recent advancements in image processing and deep learning have improved plant disease recognition, yet existing methods using only CNNs or VTs fall short due to their limited feature perception.
A study published in Plant Phenomics on 21 Jun 2024, proposes LGNet, a dual-branch network combining CNNs and VTs that enhances both local and global feature extraction, achieving state-of-the-art performance on major datasets.
The research divided LGNet's parameters into two parts for training, utilizing pretrained weights on ImageNet 1k for the dual-branch backbone network and fine-tuning with different learning rates. The model was optimized with SGD, momentum, and weight decay, and trained on a Windows 11 system with an NVIDIA GeForce RTX 3090 GPU and PyTorch.
For evaluation purposes, cross-entropy loss was used, while online data augmentation enhanced generalization. LGNet's performance was compared to single models ConvNeXt-Tiny and Swin Transformer-Tiny. The initial training accuracies were high for all models, but LGNet's accuracy improved significantly, surpassing the others by 1%–2%. On the AI Challenger 2018 and SCD datasets, LGNet achieved 88.74% and 99.08% accuracy, respectively, outperforming the single models.
Ablation experiments showed that both the AFF and HMUFF modules enhanced performance, with the full LGNet model achieving the best results, demonstrating the effectiveness of the dual-branch network and feature fusion techniques.
According to one of the study's lead researchers, Xin Zhang, "The development of robust plant disease recognition models, and improving the generalization ability of these models in real-world environments, is highly important for agricultural production."
In summary, this study presents LGNet, a dual-branch network combining CNNs and VTs for enhanced plant disease identification. Future research will focus on knowledge distillation to create lightweight, high-performance models for mobile deployment and on obtaining more real-world data to enhance model robustness, thereby improving precision agriculture and ensuring crop safety.
More information: Jianwu Lin et al, Local and global feature-aware dual-branch networks for plant disease recognition, Plant Phenomics (2024). DOI: 10.34133/plantphenomics.0208
Provided by Chinese Academy of Sciences