This article has been reviewed according to Science X's editorial process and policies. Editors have highlighted the following attributes while ensuring the content's credibility:
fact-checked
trusted source
proofread
Advanced DeepLabv3+ algorithm enhances safflower filament harvesting with high accuracy
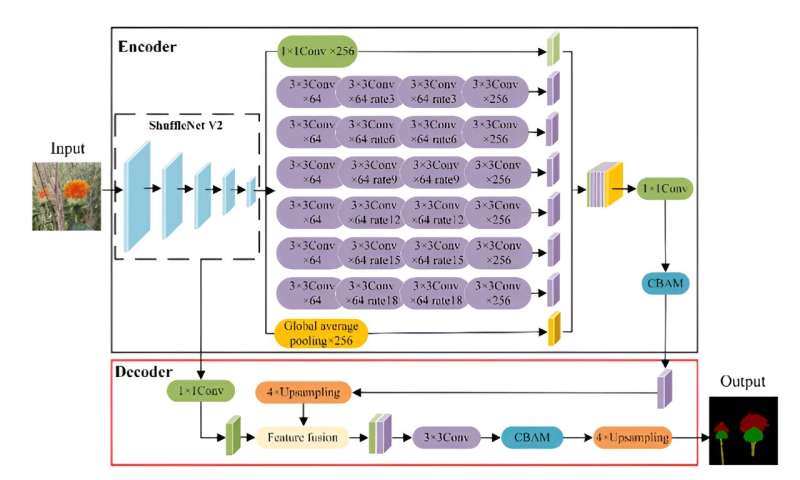
A research team has developed an improved DeepLabv3+ algorithm for accurately detecting and localizing safflower filament picking points. By utilizing the lightweight ShuffleNetV2 network and incorporating convolutional block attention, the method achieved high accuracy with a mean pixel accuracy of 95.84% and mean intersection over union of 96.87%.
This advancement reduces background interference and enhances filament visibility. The method shows potential for improved harvesting robot performance, offering promising applications for precise filament harvesting and agricultural automation.
Safflower is a crucial crop for various uses, but current labor-intensive harvesting methods are inefficient. Existing research on flower segmentation using deep learning shows promise but struggles with near-color backgrounds and blurred contours.
A study published in Plant Phenomics on 7 May 2024. This study addresses these challenges by proposing a filament localization method based on an improved DeepLabv3+ algorithm, incorporating a lightweight network and attention modules.
To improve the algorithm's performance and decrease overfitting, the SDC-DeepLabv3+ algorithm was trained with an initial learning rate of 0.01, a batch size of eight, and 1,000 iterations. Using the SGD optimizer, the learning rate was adjusted if accuracy did not increase within 15 rounds.
The training process showed a rapid decrease in loss value in the first 163 rounds, stabilizing after 902 rounds. The mean pixel accuracy (mPA) reached 92.61%, indicating successful convergence. Ablation tests revealed that integrating ShuffletNetV2 and DDSC-ASPP improved the mean intersection over union (mIoU) to 95.84% and mPA to 96.87%.
Compared to traditional DeepLabv3+, the enhanced algorithm reduced parameters and increased FPS, highlighting its efficiency. Further comparisons showed that SDC-DeepLabv3+ outperformed other segmentation algorithms, achieving higher accuracy and faster prediction speeds.
Tests under various weather conditions confirmed the algorithm's robustness, with the highest success rates for filament localization and picking observed on sunny days. Depth-measurement tests identified an optimal range of 450–510 mm, minimizing visual-localization errors. The improved algorithm demonstrated significant potential for precise and efficient safflower harvesting in complex environments.
According to the study's lead researcher, Zhenguo Zhang, "The results show that the proposed localization method offers a viable approach for accurate harvesting localization."
In summary, this study developed a method to accurately detect and localize safflower filament picking points using an improved DeepLabv3+ algorithm. Future research will focus on extending the algorithm to different safflower varieties and similar crops, and optimizing the attention mechanisms to further improve segmentation performance.
More information: Zhenyu Xing et al, SDC-DeepLabv3+: Lightweight and precise localization algorithm for safflower-harvesting robots, Plant Phenomics (2024). DOI: 10.34133/plantphenomics.0194
Provided by Chinese Academy of Sciences