This article has been reviewed according to Science X's editorial process and policies. Editors have highlighted the following attributes while ensuring the content's credibility:
fact-checked
trusted source
proofread
International collaboration lays the foundation for future AI for materials
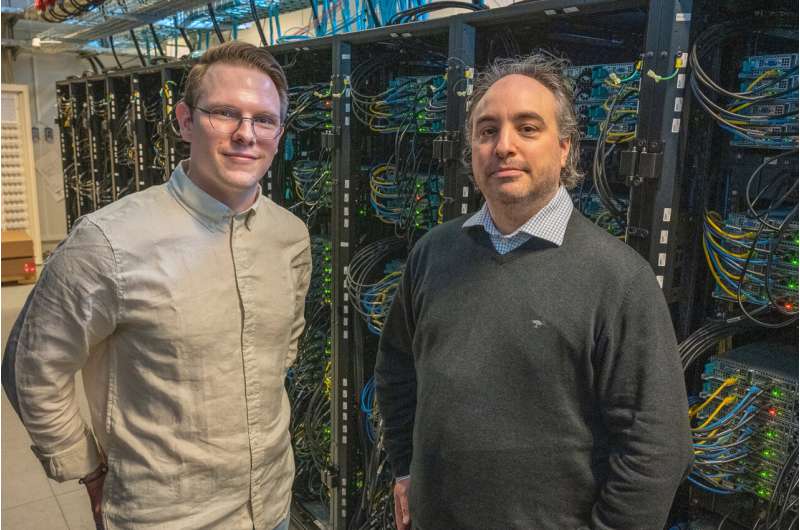
Artificial intelligence (AI) is accelerating the development of new materials. A prerequisite for AI in materials research is large-scale use and exchange of data on materials, which is facilitated by a broad international standard. A major international collaboration now presents an extended version of the OPTIMADE standard.
New technologies in areas such as energy and sustainability involving batteries, solar cells, LED lighting and biodegradable materials require new materials. Many researchers around the world are working to create materials that have not existed before. But there are major challenges in creating materials with the exact properties required, such as not containing environmentally hazardous substances and at the same time being durable enough to not break down.
"We're now seeing an explosive development where researchers in materials science are adopting AI methods from other fields and also developing their own models to use in materials research. Using AI to predict properties of different materials opens up completely new possibilities," says Rickard Armiento, associate professor at the Department of Physics, Chemistry and Biology (IFM) at Linköping University in Sweden.
Today, many demanding simulations are performed on supercomputers that describe how electrons move in materials, which gives rise to different material properties. These advanced calculations yield large amounts of data that can be used to train machine learning models.
These AI models can then immediately predict the responses to new calculations that have not yet been made, and by extension predict the properties of new materials. But huge amounts of data are required to train the models.
"We're moving into an era where we want to train models on all data that exist," says Armiento.
Data from large-scale simulations, and general data about materials, are collected in large databases. Over time, many such databases have emerged from different research groups and projects, like isolated islands in the sea. They work differently and often use properties that are defined in different ways.
"Researchers at universities or in industry who want to map materials on a large scale or want to train an AI model must retrieve information from these databases. Therefore, a standard is needed so that users can communicate with all these data libraries and understand the information they receive," says Gian-Marco Rignanese, professor at the Institute of Condensed Matter and Nanosciences at UCLouvain in Belgium.
The OPTIMADE (Open databases integration for materials design) standard has been developed over the past eight years. Behind this standard is a large international network with over 30 institutions worldwide and large materials databases in Europe and the U.S.. The aim is to give users easier access to both leading and lesser-known materials databases.
A new version of the standard, v1.2, is now being released, and is described in an article titled "Developments and applications of the OPTIMADE API for materials discovery, design, and data exchange" and published in the journal Digital Discovery.
One of the biggest changes in the new version is a greatly enhanced possibility to accurately describe different material properties and other data using common, well-founded definitions.
The international collaboration spans the EU, the UK, the US, Mexico, Japan and China together with institutions such as École Polytechnique Fédérale de Lausanne (EPFL), University of California Berkeley, University of Cambridge, Northwestern University, Duke University, Paul Scherrer Institut, and Johns Hopkins University.
More information: Matthew Evans et al, Developments and applications of the OPTIMADE API for materials discovery, design, and data exchange, Digital Discovery (2024). DOI: 10.1039/D4DD00039K
Provided by Linköping University