This article has been reviewed according to Science X's editorial process and policies. Editors have highlighted the following attributes while ensuring the content's credibility:
fact-checked
proofread
Enhancing identifiability in plant growth models: A comprehensive framework for precision and reliability
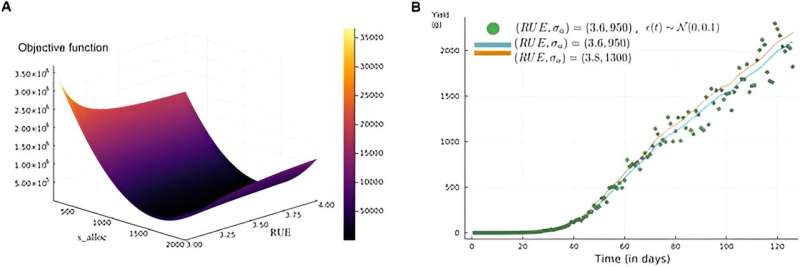
In the evolving landscape of plant growth modeling, there is a distinguished presence of mechanistic models aimed at capturing the intricate dynamics of plant development through parameter estimation from experimental data. However, these models face challenges in ensuring the uniqueness of parameter solutions, a problem addressed by structural and practical identifiability analyses.
Despite a generally consistent definition of structural identifiability in the literature, practical identifiability lacks a unified quantification approach, leading to diverse, sometimes inconsistent indices. This research gap underscores the need for a standardized methodology to assess practical identifiability.
In February 2024, Plant Phenomics published a research article titled "Practical Identifiability of Plant Growth Models: A Unifying Framework and Its Specification for Three Local Indices." This study advances the field of plant growth modeling by establishing a unified framework for identifiability analysis, which adeptly incorporates various definitions tailored to specific application contexts.
In this study, by focusing on three principal methodsâcollinearity indices, profile likelihood, and average relative error, researchers uncover their limitations in local applications and propose a novel risk index based on profile likelihood confidence intervals to enhance practical identifiability analysis. Through meticulous case studies on a discrete-time individual plant growth model (LNAS) and a continuous-time plant population epidemics model, researchers demonstrate the utility of the approach.
Findings also reveal significant insights into the identifiability challenges within these models. For instance, in the LNAS model, designed to predict biomass allocation in sugar beets, researchers highlight compensation effects among parameters, particularly between the extinction coefficient and radiation use efficiency, which obscure their unique identification.
Further, the analysis extends to exploring the dynamics of yield over time, underscoring the near-indistinguishability of output despite varied parameter values. This phenomenon underscores the practical identifiability issues arising from parameter interactions. Similarly, in the plant population epidemics model, researchers identify key parameters that, due to their interdependence, present challenges in achieving reliable estimations.
The innovative risk index and the use of profile likelihood-based confidence intervals offer a refined lens for assessing identifiability, suggesting a shift from binary indicators to a more nuanced quantification of risk.
Overall, this research not only elucidates the intricacies of identifiability analysis but also provides practical guidance for modelers, paving the way for the systematic inclusion of identifiability checks in modeling studies to ensure the credibility of model predictions.
More information: Jean Velluet et al, Practical Identifiability of Plant Growth Models: A Unifying Framework and Its Specification for Three Local Indices, Plant Phenomics (2023). DOI: 10.34133/plantphenomics.0133
Provided by TranSpread