This article has been reviewed according to Science X's editorial process and policies. Editors have highlighted the following attributes while ensuring the content's credibility:
fact-checked
trusted source
proofread
Machine learning techniques enhance the discovery of excited nuclear levels in sulfur-38
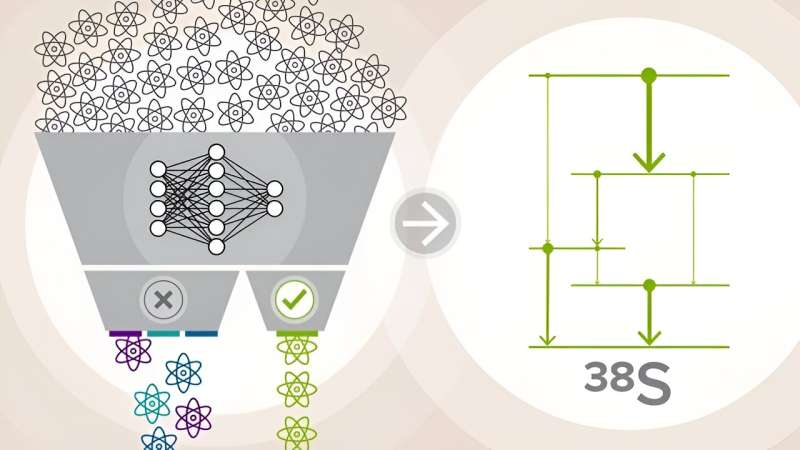
Fixed numbers of protons and neutrons—the building blocks of nuclei—can rearrange themselves within a single nucleus. The products of this reshuffling include electromagnetic (gamma ray) transitions. These transitions connect excited energy levels called quantum levels, and the pattern in these connections provide a unique "fingerprint" for each isotope.
Determining these fingerprints provides a sensitive test of scientists' ability to describe one of the fundamental forces, the strong (nuclear) force that holds protons and neutrons together.
In the laboratory, scientists can initiate the movement of protons and neutrons through an injection of excess energy using a nuclear reaction.
In a paper, published in Physical Review C, researchers successfully used this approach to study the fingerprint of sulfur-38. They also used machine learning and other cutting-edge tools to analyze the data.
The results provide new empirical information on the "fingerprint" of quantum energy levels in the sulfur-38 nucleus. Comparisons with theoretical models may lead to important new insights. For example, one of the calculations highlighted the key role played by a particular nucleon orbital in the model's ability to reproduce the fingerprints of sulfur-38 as well as neighboring nuclei.
The study is also important for its first successful implementation of a specific machine learning-based approach to classifying data. Scientists are adopting this approach to other challenges in experimental design.
Researchers used a measurement that included a machine learning (ML) assisted analysis of the collected data to better determine the unique quantum energy levels—a "fingerprint" formed through the rearrangement of the protons and neutrons—in the neutron-rich nucleus sulfur-38.
The results doubled the amount of empirical information on this particular fingerprint. They used a nuclear reaction involving the fusion of two nuclei, one from a heavy-ion beam and the second from a target, to produce the isotope and introduce the energy needed to excite it into higher quantum levels.
The reaction and measurement leveraged a heavy-ion beam produced by the ATLAS Facility (a Department of Energy user facility), a target produced by the Center for Accelerator and Target Science (CATS), the detection of electromagnetic decays (gamma-rays) using the Gamma-Ray Energy Tracking Array (GRETINA), and the detection of the nuclei produced using the Fragment Mass Analyzer (FMA).
Due to complexities in the experimental parameters—which hinged between the production yields of the sulfur-38 nuclei in the reaction and the optimal settings for detection—the research adapted and implemented ML techniques throughout the data reduction.
These techniques achieved significant improvements over other techniques. The ML-framework itself consisted of a fully connected neural network that was trained under supervision to classify sulfur-38 nuclei against all other isotopes produced by the nuclear reaction.
More information: C. R. Hoffman et al, Experimental study of the 38S excited level scheme, Physical Review C (2023). DOI: 10.1103/PhysRevC.107.064311. On arXiv (2023): DOI: 10.48550/arxiv.2305.16969
Provided by US Department of Energy