This article has been reviewed according to Science X's editorial process and policies. Editors have highlighted the following attributes while ensuring the content's credibility:
fact-checked
trusted source
proofread
Deep learning reveals molecular secrets of explosive perchlorate salts
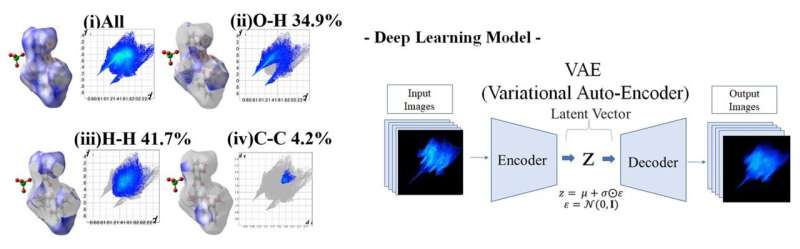
Perchlorates are a class of compounds that are notorious for their explosive nature. This raises safety concerns during experiments involving complex compounds that contain perchlorate ions, since explosions can be triggered even by the slightest shock or heat. It is, therefore, important to study their molecular structure and understand the reason behind their explosive nature.
In this context, a method called the Hirschfield surface analysis has been extensively used for visualizing and quantifying the crystal structure and molecular interactions of crystal compounds. A two-dimensional fingerprint plot derived from the Hirschfield analysis vividly shows the complex interactions in crystals.
Despite their advantages, however, these methods rely only on the judgment of the human eye, limiting their overall effectiveness. Looking for a way to overcome these limitations, recent studies have explored the use of deep learning and artificial intelligence (AI) methods for analysis. These studies have pointed to the potential benefit of using AI to unveil the features that are challenging to discern for humans.
Now, to fully realize the potential of Hirschfield surface analysis, a team of researchers, led by Professor Takashiro Akitsu from the Department of Chemistry and the Center for Fire Science and Technology at the Tokyo University of Science (TUS) in Japan, recently employed deep learning to analyze the Hirschfield surface of salen-type metal complexes. The study team also included Yuji Takiguchi, Shintaro Suda, and Assistant Professor Daisuke Nakane, all from TUS.
Salen-type complexes are an emerging and lucrative area of research, primarily due to their diverse functions. "Actual experiments on explosive and thermal properties of these materials are accurate but extremely dangerous, and therefore, using AI to study these properties by solely analyzing the crystal structure can be quite advantageous," explains Akitsu. The findings of this study were published in the journal FirePhysChem on 30 December 2023.
The team developed extensive datasets of the Hirschfield fingerprint plots of the salen-type metal complexes stored in the Cambridge Crystal Database (CCDC) and used deep learning to study the features of the crystal structure that contribute to their explosiveness. To this end, the researchers also employed a special variational autoencoder using which they transformed the information embedded in the fingerprint plot images into a low-dimensional vector. This enabled them to quantitatively study the shapes of the plots, which, until now was only done qualitatively.
Their analysis revealed that the salen-type metal complexes lack any distinctive structural features, indicating that their explosive nature is linked to the chemical bonding of the perchlorate ions and their surrounding intermolecular interactions.
Highlighting the importance of this study, Prof. Akitsu explains, "Traditionally, the field of crystal engineering has concentrated solely on the interactions of small molecules in crystals. But in the future, interactions in complex systems will gain significance. This means that studying their intermolecular interactions will become even more crucial. Our novel method can help in studying such interactions by understanding the crystal structure alone. Moreover, it can also contribute to the discovery of new drugs and advance catalytic research."
This study also makes use of the CCDC, which, despite having more than 1 million entries, is still underutilized. Moreover, the innovative method proposed in this study can promote the use of this database, and can lead to the discovery of new and interesting compounds.
Overall, the study offers insights into the explosive nature of perchlorates, besides also presenting a safer data-driven method for studying the physical properties of compounds, advancing crystal engineering and energetic materials research.
More information: Takashiro Akitsu et al, Deep-learning prediction of safety moiety of salen-type complex crystals towards explosive perchlorate salts, FirePhysChem (2023). DOI: 10.1016/j.fpc.2023.12.004
Provided by Tokyo University of Science