This article has been reviewed according to Science X's editorial process and policies. Editors have highlighted the following attributes while ensuring the content's credibility:
fact-checked
trusted source
proofread
AI algorithm keeps a mile-long particle accelerator healthy
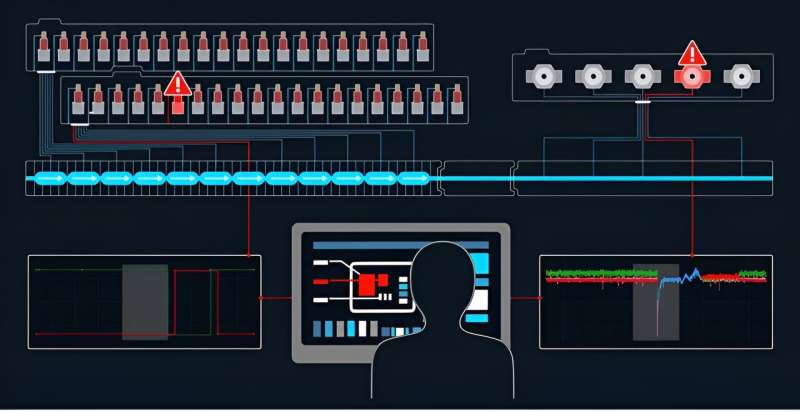
Particle accelerators are among the most intricate scientific instruments ever devised. With millions of sensors and thousands of subsystems at risk of failure, these accelerators' human operators must continuously monitor performance and comb through a sea of sensors to identify problems. That's the situation at the Linac Coherent Light Source, a Department of Energy user facility at SLAC National Accelerator Laboratory.
Researchers have now developed an artificial intelligence (AI) algorithm that mimics how human operators approach this challenge. The automated system keeps an eye on the accelerator. It alerts operators when performance dips and identifies the specific subsystem that is responsible. This can simplify accelerator operation, reduce downtime, and enhance the scientific data these tools collect. The research was published in Physical Review Accelerators and Beams.
The automated AI solution shows SLAC operators which components should be switched off and replaced to keep an accelerator running around the clock. Improved reliability also keeps more subsystems online. This allows the accelerator to reach its full operating capability. This AI approach could benefit many complex systems. For example, it could improve reliability in other experimental facilities, advanced manufacturing plants, the electric grid, and nuclear power plants.
Modern accelerators record millions of data streams, far too many signals for a small operations team to monitor in real time and reliably avoid subsystem faults leading to costly downtime. For instance, in the Linac Coherent Light Source, one of the world's first X-ray lasers, faults in the radiofrequency (RF) stations that accelerate the electrons are a primary cause of downtime and drops in performance.
An existing automated algorithm tries to identify RF station problems, but almost 70% of the algorithm's predictions are false positives, and operators resort to manual inspection to identify RF station anomalies.
Inspired by the operators, the AI method simultaneously runs anomaly detection algorithms on both the RF station diagnostics and shot-to-shot measurements of the final beam quality. A fault is predicted only when both algorithms simultaneously identify anomalies. This approach—now incorporated into the control room—can be entirely automated and identifies more events with fewer false positives than the RF station diagnostics alone.
Recent patent-pending work has extended the coincidence concept to deep-learning algorithms, such as neural networks, which can identify faults on raw, unlabeled data without expert input. Researchers expect these machine learning-driven algorithms to have broad applications in complex systems across science and industry.
More information: Ryan Humble et al, Beam-based rf station fault identification at the SLAC Linac Coherent Light Source, Physical Review Accelerators and Beams (2022). DOI: 10.1103/PhysRevAccelBeams.25.122804
Provided by US Department of Energy