This article has been reviewed according to Science X's editorial process and policies. Editors have highlighted the following attributes while ensuring the content's credibility:
fact-checked
peer-reviewed publication
trusted source
proofread
New tool to help AI track animals could boost biology research
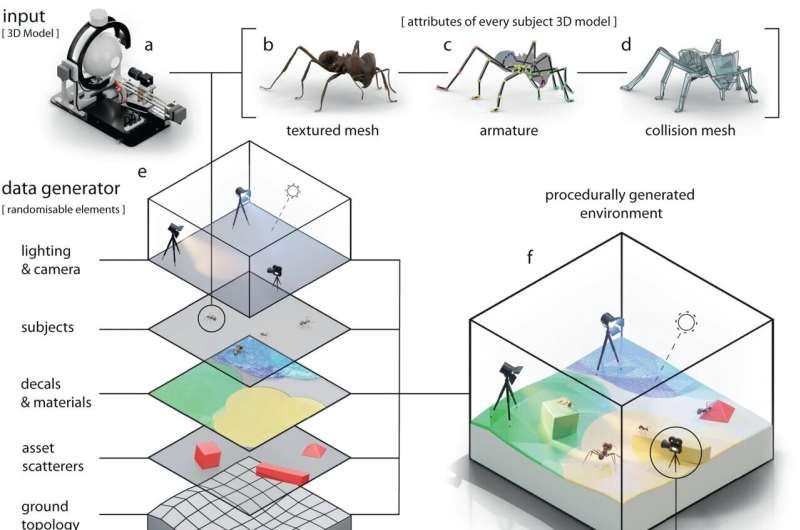
Biologists often study large numbers of animals to collect data on collective and individual behavior. New machine learning tools promise to help scientists process the huge amount of data this work generates more quickly while lessening workload.
Now, a new tool called replicAnt simplifies and streamlines the way the training images for these machine-learning tools are created, making it quicker and easier to record observations about lots of animals at once, starting with insects.
Animal database
Existing AI-enabled tools for this purpose require users to painstakingly hand annotate hundreds of frames to provide a database for the computer to learn from. To combat this, replicAnt automatically creates thousands of annotated images with the click of a mouse, seamlessly incorporating variations in species and environments. Ultimately, these AI-generated data may increase the speed and robustness of using AI tools in animal research.
The work is published in Nature Communications.
Lead author Fabian Plum, Ph.D. researcher at Imperial College London's Department of Bioengineering, said, "It takes a lot of time to set up studies on large numbers of animals and to learn how to use new tools. replicAnt lowers the entry barrier for biologists to use machine learning to optimize their work."
The tool builds on the research team's previous tool, scAnt—a 3D scanner that photographs small animals in meticulous detail to produce high-resolution 3D models of critters. The 3D models generated by scAnt were used within replicant, which uses the 3D software Unreal Engine to produce training images for detecting and tracking animals in the lab and in nature, freeing up researchers' time and streamlining their work.
To demonstrate the utility of replicAnt, the researchers trained neural networks—sets of algorithms that recognize underlying relationships in data—on these images. This allowed the neural networks to recognize individuals and track their movements across different environments out-of-the-box. For others, the required hand-labeling of real images was reduced by an order of magnitude.
Fabian said, "Understanding animal behavior, particularly as our climate changes, is crucial. We hope our tool can help to make the time-intensive process of collecting crucial data easier and faster."
Further applications might include using real-time movement data to inform character movement in film and video games.
More information: Fabian Plum et al, replicAnt: a pipeline for generating annotated images of animals in complex environments using Unreal Engine, Nature Communications (2023). DOI: 10.1038/s41467-023-42898-9
Journal information: Nature Communications
Provided by Imperial College London