This article has been reviewed according to Science X's editorial process and policies. Editors have highlighted the following attributes while ensuring the content's credibility:
fact-checked
proofread
LIBRA: An adaptative integrative tool for paired single-cell multi-omics data
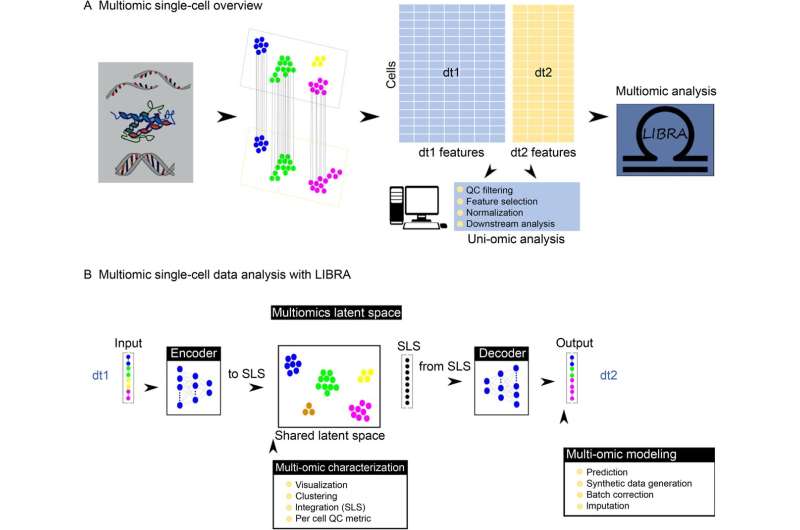
Single-cell multi-omics technologies allow a profound system-level biology understanding of cells and tissues. However, an integrative and possibly systems-based analysis capturing the different modalities is challenging. In response, bioinformatics and machine learning methodologies are being developed for multi-omics single-cell analysis.
It is unclear whether current tools can address the dual aspect of modality integration and prediction across modalities without requiring extensive parameter fine-tuning.
Now, researchers have designed LIBRA, a neural network-based framework, to learn translation between paired multi-omics profiles so that a shared latent space is constructed. Additionally, they implemented a variation, aLIBRA, that allows automatic fine-tuning by identifying parameter combinations that optimize both the integrative and predictive tasks. The paper is published in the journal Quantitative Biology.
All model parameters and evaluation metrics are made available to users with minimal user iteration. Furthermore, aLIBRA allows experienced users to implement custom configurations. The LIBRA toolbox is freely available as R and Python libraries at GitHub (TranslationalBioinformaticsUnit/LIBRA).
LIBRA was evaluated in eight multi-omic single-cell data sets, including three combinations of omics. We observed that LIBRA is a state-of-the-art tool when evaluating the ability to increase cell-type (clustering) resolution in the integrated latent space.
Furthermore, when assessing the predictive power across data modalities, such as predictive chromatin accessibility from gene expression, LIBRA outperforms existing tools. As expected, adaptive parameter optimization (aLIBRA) significantly boosted the performance of learning predictive models from paired data-sets.
More information: Xabier Martinez‐de‐Morentin et al, LIBRA: an adaptative integrative tool for paired single‐cell multi‐omics data, Quantitative Biology (2023). DOI: 10.15302/J-QB-022-0318
Provided by Higher Education Press