This article has been reviewed according to Science X's editorial process and policies. Editors have highlighted the following attributes while ensuring the content's credibility:
fact-checked
proofread
Machine learning reveals how to dissolve polymeric materials in organic solvents
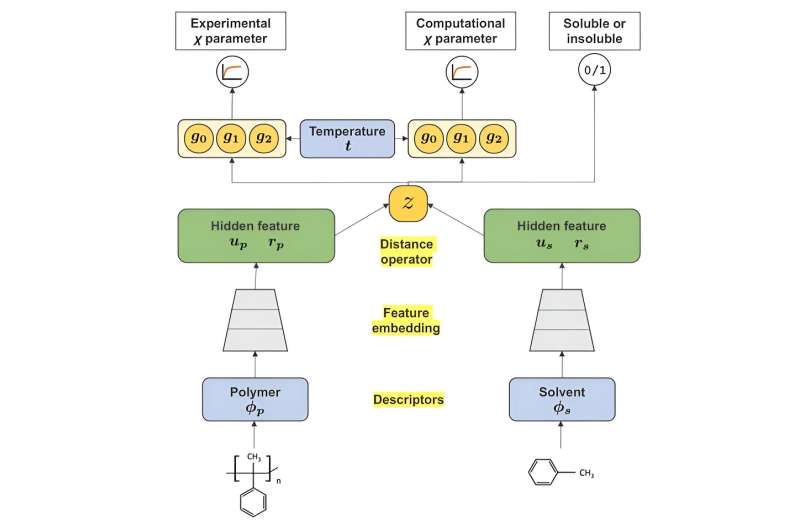
Dissolving polymers with organic solvents is the essential process in the research and development of polymeric materials, including polymer synthesis, refining, painting, and coating. Now more than ever recycling plastic waste is a particularly imperative part of reducing carbon produced by the materials development processes.
Polymers, in this instance, refer to plastics and plastic-like materials that require certain solvents to be able to effectively dissolve and therefore become recyclable, though it's not as easy as it sounds. Utilizing Mitsubishi Chemical Group's (MCG) databank of quantum chemistry calculations, scientists developed a novel machine learning system for determining the miscibility of any given polymer with its solvent candidates, referred to as χ (chi) parameters.
This system has enabled scientists to overcome the limitations arising from a limited amount of experimental data on the polymer-solvent miscibility by integrating massive data produced from the computer experiments using high-throughput quantum chemistry calculations.
Researchers published their work in the journal Macromolecules.
By integrally handling the two different datasets from quantum chemistry calculations and real experiments in a methodological framework called multitask learning, researchers have successfully constructed a model that can predict the miscibility of any polymer-solvent mixture system with extremely high accuracy.
This model can be used to select and newly design solvent molecules that are added to plastic waste consisting of a mixture of different types of plastics to selectively separate only certain materials. Such designed solvents are also used as "miscibilizers" to create high-performance polymer blends.
"In recent years in particular, expectations for technological innovations for recycling waste plastic resources are growing rapidly as we move toward realizing decarbonized societies. The development of miscibilizers for various types of polymers will be essential in improving the recycling rates of waste plastics," said Ryo Yoshida, author and researcher of the study.
The predictive model developed by researchers can calculate the χ parameters about 40 times as fast as conventional quantum chemistry calculations. Using this model, screening of millions of orders of magnitude of candidate solvent molecules can be performed at ultrahigh speed.
So far, the model has proven to be accurate when it comes to figuring out what is needed to make the polymer and solvent one homogenous mixture suitable for recycling appropriately, taking a lot of the guess work and trial and error out of creating a miscible substance suitable for the appropriate method of recycling.
But with any new and emerging technology, there is always some work that can be done to streamline the process and work out the kinks before it's truly ready for large-scale use.
"In order to further improve and extend machine learning techniques and to promote open innovation and open science in the field of materials informatics, we have made part of the developed source code and data available to the public," said Yoshida.
Some limitations are the lack of the model's capacity to determine the dependence of a polymer's miscibility on its molecular weight or other compositional features, but opening the data and some parts of the source code to the public can increase the data set available to the model, allowing it to intake that information and learn more for better representation of a polymer's true miscibility. Open innovation and crowd-sourcing data can be extremely useful in getting a lot of data in a relatively short period of time.
The ability to predict and understand polymer miscibility could prove to be a significant innovation in future technology when it comes to materials development and the continued need to intelligently recycle waste plastics as our society moves away from plastic-based materials.
More information: Yuta Aoki et al, Multitask Machine Learning to Predict Polymer–Solvent Miscibility Using Flory–Huggins Interaction Parameters, Macromolecules (2023). DOI: 10.1021/acs.macromol.2c02600
Provided by Research Organization of Information and Systems