This article has been reviewed according to Science X's editorial process and policies. Editors have highlighted the following attributes while ensuring the content's credibility:
fact-checked
peer-reviewed publication
trusted source
proofread
Novel algorithm able to detect mutations in single-cell sequencing data sets
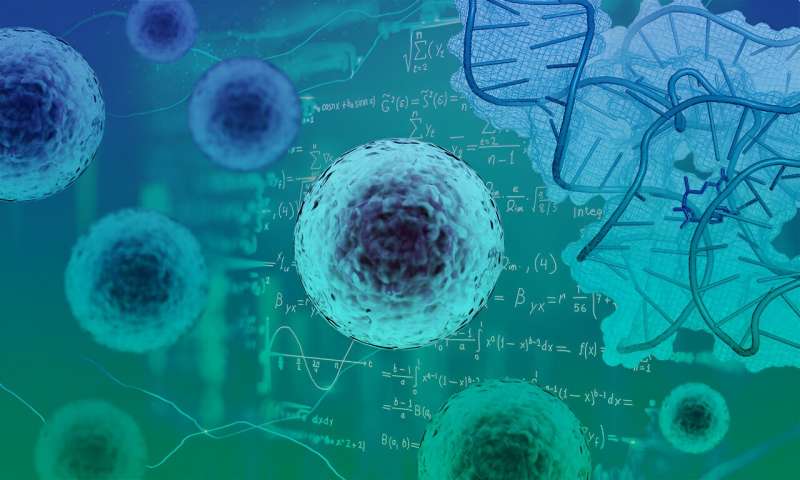
Single-cell RNA sequencing data are useful for studying cell phenotypes and function. However, deciphering the clonal relationships of cells is critical to understanding the patterns of cell migration during development and tissue growth, and to studying the relationship between genomic mutations and cell function.
Mapping clonal relationships to cell phenotypes can be achieved by detecting somatic mutations in single cells. Until now, detecting somatic mutations in individual cells remained technically challenging because single-cell RNA data are sparse by definition—meaning only a small fraction of the data is captured—and have many sequencing errors.
EMBL's European Bioinformatics Institute (EMBL-EBI) has developed a new algorithm able to detect somatic mutations in single-cell profiling data without requiring a reference sample, such as matched genome sequencing data. This can be done at cell type and single-cell resolution. The work is published in the journal Nature Biotechnology.
The algorithm, called SComatic, allows researchers to study cancer evolution and patterns of mutations in healthy cells within tissues. It can also be used to study a number of fundamental biological processes, including:
- clonal mosaicism—where subpopulations of cells in a tissue have slightly different genetic information than the rest due to the accumulation of somatic mutations
- cell plasticity—a cell's ability to change its phenotypes in response to environmental factors, without changes in the genotype
- cancer evolution and intra-tumor heterogeneity
- tissue architecture and patterns of cell migration during development
SComatic also allows researchers to answer questions such as what mutation events have taken place in a specific cell, or how many mutations there are in a specific cell or cell type compared to others. More widely, SComatic allows scientists to map genotype to phenotype at single-cell resolution. This is particularly useful for scientific initiatives analyzing single-cell data, such as the Human Cell Atlas.
"SComatic is specifically designed for de novo detection of somatic mutations in high throughput single-cell profiling data," said Francesc Muyas Remolar, postdoctoral fellow at EMBL-EBI.
"It's at least five times more precise than other somatic detection algorithms, enabling scientists to study topics that were inaccessible before, such as the cell of origin from which some cancers and diseases originate. I look forward to seeing how colleagues apply SComatic to address diverse research questions."
"Being able to bypass the need for a reference sample in this context is a major technical advancement," said Isidro Cortes-Ciriano, research group leader at EMBL-EBI. "We can now harness the large collections of existing and upcoming single-cell data sets to study somatic mutations at unprecedented resolution."
More information: Francesc Muyas et al, De novo detection of somatic mutations in high-throughput single-cell profiling data sets, Nature Biotechnology (2023). DOI: 10.1038/s41587-023-01863-z
Journal information: Nature Biotechnology
Provided by European Molecular Biology Laboratory