This article has been reviewed according to Science X's editorial process and policies. Editors have highlighted the following attributes while ensuring the content's credibility:
fact-checked
peer-reviewed publication
trusted source
proofread
A graph neural network for fast evaluation of the adsorption energy in heterogeneous catalysis
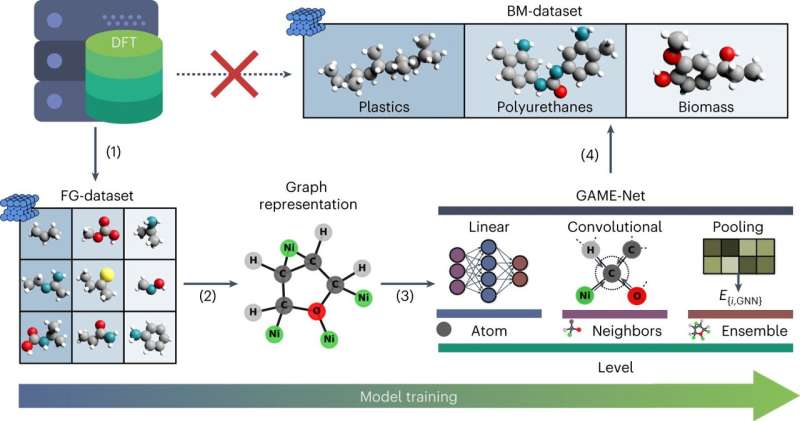
Achieving sustainability goals will require the use of feedstocks such as plastics and biomass instead of oil. Moreover, industries are constantly searching for more efficient and sustainable processes, which often require precise simulations. In the field of heterogeneous catalysis, determining the energy of molecules adsorbed on surfaces is crucial to estimate catalyst performance, which is typically done using density functional theory (DFT). However, for large organic molecules like those in plastics and biomass, this cannot be done.
To address this issue, scientists from the Prof. López research group at the Institute of Chemical Research of Catalonia (ICIQ-CERCA) in collaboration with researchers from The Matter Lab (University of Toronto), have created a new model called GAME-Net, a graph neural network that rapidly evaluates adsorption energy when molecules attach to surfaces. Their research is published in the journal Nature Computational Science.
Trained on a diverse dataset of small and medium-sized molecules containing functional groups, GAME-Net achieves a mean absolute error (MAE) of 0.17 eV on the test set and is six orders of magnitude faster than DFT. When applied to biomass (wood) and plastic molecules with up to 30 atoms, the model predicts adsorption energies with a MAE of 0.016 eV/atom.
GAME-Net is capable of estimating adsorption energy with an accuracy comparable to DFT, while utilizing a simple molecular representation. This breakthrough model opens up the possibility of predicting adsorption energy for larger molecules derived from biomass, polyurethanes, and plastics, enabling the study of chemical systems that are not easily amenable to DFT calculations. This revolutionary technology can be easily run on a laptop, making it accessible to scientists and researchers around the world.
"For us, this work represents a starting point for the practical implementation of Machine Learning algorithms for quantum chemistry in the typical workflow of both, experimental and computational, researchers in heterogenous catalysis. Even though GAME-Net presents some limitations, its speed, coupled with the simplicity in its structure, and the way of interfacing it, makes this model a valuable tool for the community," states Santiago Morandi from ICIQ.
Let's 'KISS': Keep it small and simple
The growing popularity of artificial intelligence has led to numerous attempts to improve its predictive capabilities. Among them are Graph Neural Networks (GNNs), a type of neural networks capable of learning the "shape" of data.
The algorithm proposed by the researchers employs a simple graph representation where nodes correspond to atoms and edges represent chemical bonds. In order to predict the energy change when a catalyst interacts with a reactant molecule, a graph neural network is used, when typically, this change is obtained from DFT.
GAME-Net was trained on a diverse set of small molecules containing different heteroatoms such as nitrogen, oxygen, and sulfur. To initiate the process, researchers created the "functional groups" (FG)-dataset from scratch, which comprises 207 organic molecules adsorbed on 14 transition metals (Ag, Au, Cd, Co, Cu, Fe, Ir, Ni, Os, Pd, Pt, Rh, Ru, and Zn) on their lowest surface energy facets.
To assure high reproducibility standards all the computational data generated in this study is accessible from the ioChem-BD repository. The code of the GAME-Net, together with the pre-trained model, have been released as open-source under the MIT license and can be downloaded from a repository.
"With this work we wanted to see if we were able to teach complex chemical interactions to an AI using the chemical representations we teach kids in high school. Surprisingly, our AI turned out to be an excellent student," says Dr. Pablo-García Ph.D. student at ICIQ now at the University of Toronto.
This innovative AI framework offers a powerful tool for quick screening of catalytic materials, especially for systems that are too challenging to simulate using traditional methods. Chemical industries can benefit from the integration of artificial intelligence in their research, to accelerate the understanding and design of heterogeneous catalysts for the development of more sustainable processes. GAME-Net is open to new and exciting improvements, such as specific reaction steps and, as well, more complex catalyst materials.
"Beyond the short-term impact our work may have, our model demonstrates that AIs are capable of describing complex chemical interactions using extremely simple models, making them more accessible to scientists who want to accelerate their research," conclude Dr. Pablo-García and Santiago Morandi.
More information: Sergio Pablo-García et al, Fast evaluation of the adsorption energy of organic molecules on metals via graph neural networks, Nature Computational Science (2023). DOI: 10.1038/s43588-023-00437-y
Journal information: Nature Computational Science
Provided by Institute of Chemical Research of Catalonia