This article has been reviewed according to Science X's editorial process and policies. Editors have highlighted the following attributes while ensuring the content's credibility:
fact-checked
trusted source
proofread
Using artificial intelligence for culture medium optimization
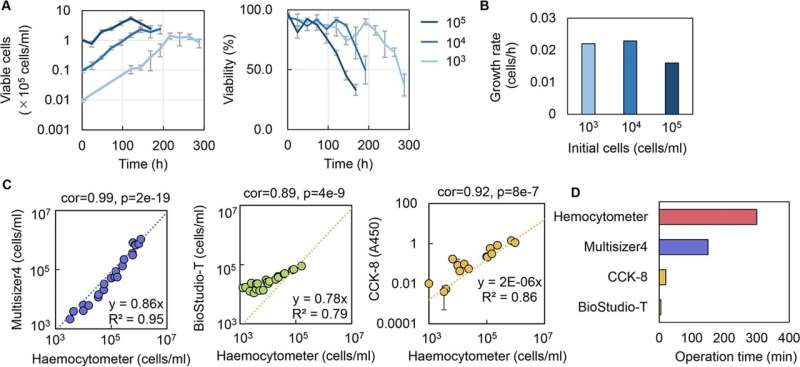
Cell culture is a vital technology used in pharmaceutical production and regenerative medicine. It is heavily influenced by the composition of the medium, a nutrient-rich solution facilitating cell growth. Optimizing and developing culture media is a critical task in various sectors, including food, pharmaceuticals, bioenergy, and materials.
However, as the culture media varies according to cell type, creating a specific medium for each purpose demands substantial time and labor. Therefore, more efficient techniques for culture medium development are needed. A study, recently published in the journal npj Systems Biology and Applications, uses artificial intelligence, specifically machine learning, to develop high-performance culture media, reducing the associated labor.
A total of 232 media, containing 31 different nutrients, were used to culture cells derived from human cervical cancer. The experimental data obtained was then subjected to machine learning to predict superior medium compositions that would yield a higher cellular activity. Active learning was used to enhance prediction accuracy.
As a result, a culture medium was developed that promoted higher cell activity than the commercial medium. Moreover, the optimized compositions for the early and late stages of cell culture were found to differ, and the decision-making components were identified.
These results demonstrate the practicality of efficiently optimizing medium compositions using artificial intelligence. The methodology used in this study can be applied to develop culture media for various cell lines and culture purposes. This considerably contributes to a broad spectrum of industrial and academic research that uses cell culture as a foundational technology.
More information: Takamasa Hashizume et al, Employing active learning in the optimization of culture medium for mammalian cells, npj Systems Biology and Applications (2023). DOI: 10.1038/s41540-023-00284-7
Provided by University of Tsukuba