Deep learning and holography create a better point-of-care sensor
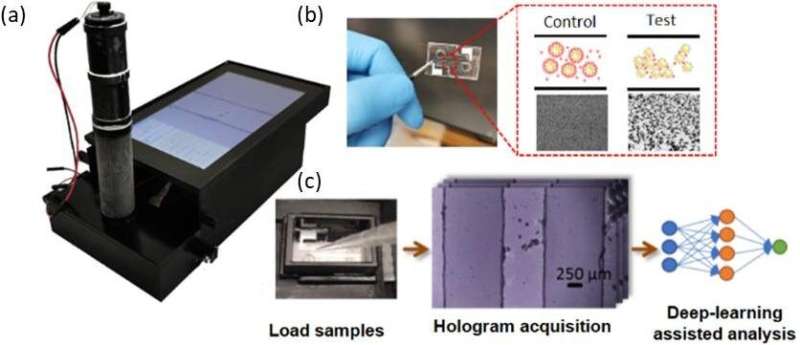
Agglutination assays are widely used immunological sensors based on antigen-antibody interactions that result in clumping of antibody-coated microscopic particles. Once the sample—for example, a patient's serum—is introduced, the corresponding target antigens in the sample rapidly attach to the antibody binding sites and the particles start to form clusters due to the target antigen's capability of binding to different sites simultaneously. The level of clustering among the particles is indicative of the amount of antigen present in a sample. These particle-based sensors have been used to test for antigens in a number of bodily fluids, and to diagnose a wide range of diseases. Its major advantages in point-of-care diagnostics include short reaction time, low sample volume, low-cost, and high specificity. One of the barriers to its wider adoption lies in the assay's low sensitivity and lack of quantitative measurements.
In a new paper published in Lab on a Chip, a team of scientists from the University of California, Los Angeles (UCLA) has developed a rapid and cost-effective particle agglutination based sensor that is powered by holographic imaging and deep learning. A disposable capillary-based flow device is designed to host the agglutination reaction with a material cost of under 2 cents per test. A mobile and inexpensive holographic microscope captures a movie of the sample, monitoring the particle clustering process over 3 minutes. This captured holographic movie is rapidly processed by trained neural networks to automatically measure the target analyte concentration within the sample.
The efficacy of this deep learning-enabled point-of-care sensor was demonstrated by accurately measuring C-reactive protein concentration in human serum samples. C-reactive protein (CRP) is a general biomarker produced by the liver in response to inflammation within the body and is commonly used as an indicator of myocardial dysfunction and heart failure. Tested on various serum samples from unique patients, this computational sensor was able to accurately measure the concentration of CRP within the high-sensitivity range, spanning 0-10µg/mL. Importantly, this mobile sensor was also able to successfully detect very high CRP concentrations, far exceeding 10µg/mL, which is in general difficult for other sensors to measure due to their limited dynamic range of analyte concentration.
"This mobile, deep learning-enabled holographic sensor is highly accurate and cost-effective, which makes it ideal for a variety of point-of-care related diagnostics applications," said Professor Aydogan Ozcan, the Chancellor's Professor of Electrical and Computer Engineering at UCLA and an associate director of the California NanoSystems Institute, who is the senior corresponding author of the work.
This research was led by Dr. Ozcan, in collaboration with Dr. Omai Garner, a Professor of Clinical Microbiology and the Director of Point of Care Testing at UCLA. The other authors of this work are Yi Luo, Hyou-Arm Joung, Sarah Esparza, and Jingyou Rao of UCLA. Dr. Ozcan also has UCLA faculty appointments in bioengineering and surgery, and is an HHMI Professor. This research was funded by the National Science Foundation, PATHS-UP Engineering Research Center.
More information: Yi Luo et al. "Quantitative particle agglutination assay for point-of-care testing using mobile holographic imaging and deep learning", Lab on a Chip (2021). DOI: 10.1039/d1lc00467k
Journal information: Lab on a Chip