Learning to synthesize: Robust phase retrieval at low photon counts
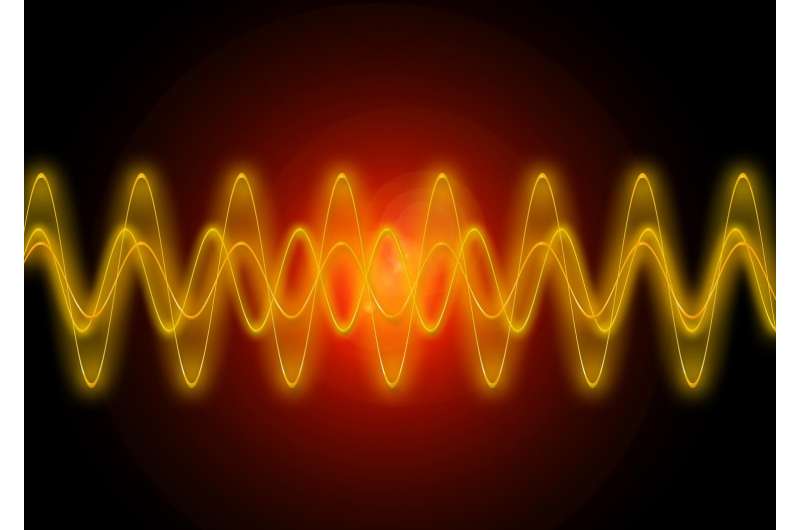
An artifact-free computational approach to extract the phase of light from noisy intensity signals improves imaging of transparent objects, such as biological cells, under low light conditions. The procedure separates intensity signals into high- and low-frequency spectral channels. Deep neural networks are trained to operate on these two frequency bands, before a final algorithm recombines them into a full-band phase image. This method avoids the tendency of automatic phase extraction programs to over-represent low frequencies.
The retrieval of phase of electromagnetic fields is one of the most important problems in optics as it allows the shape of transparent objects, including cells, to be quantified using visible light. Phase is a quantity that relates to the wave nature of light; it is not directly detectable by our eyes or common cameras, and yet carries important information about objects the light went through. Measuring the phase with very little light can be even more interesting and useful. With low light incidence, light toxicity to biological samples, for example, is reduced; however, the problem of recovering the phase also becomes much more difficult. Previous deep learning-based algorithms did improve over traditional methods under low light conditions, but displayed a tendency to over-represent the low spatial frequencies in the reconstructions, meaning that the reconstructions looked blurry.
In a new paper published in Light Science & Applications, scientists from the 3-D Optical Systems Group of Massachusetts Institute of Technology (MIT) proposed the learning to synthesize by DNN (LS-DNN) approach to combat this uneven fidelity by splitting the input signal into low and high spatial frequency bands. It then became possible for deep neural networks to process these two frequency bands, respectively; subsequently, a third neural network learned to synthesize the two frequency bands into the final reconstruction that is of high fidelity in all frequency bands. The authors discovered that the LS method is particularly robust at handling extremely noisy intensity signals.
The scientists summarize the impact of their LS-DNN algorithm as:"We proposed a framework such that when we intentionally operate the learning algorithms outside their zone of comfort, i.e. with types of examples different than the algorithms were trained with, does not lead to disaster as with alternate approaches. That's thanks to the splitting and recombining structure of the architecture that we came up with. For example, the low frequency bands are processed by a neural network that knows how to handle low frequencies but doesn't necessarily care what type of objects the low frequencies came from. Similarly for the high frequencies. The synthesizer is also trained to recombine the two bands optimally. This kind of robustness suggests that the algorithm is readily applicable in practical situations."
"The main advantage of operating in the low light regime is that you can reduce the amount of light you deliver to the specimen. Our experiments were carried out with visible light; however, the same principles apply to other bands of electromagnetic radiation, e.g. X-rays. We know that X-rays are harmful, so if you can obtain the same image quality but with much lower dose of radiation, that would be a big advance."
"The LS method is versatile and adaptable to a great variety of such problems that we call inverse, meaning you have an indirect and incomplete or noisy observation of an object, and you're trying to reveal the object itself correctly. From X-rays we mentioned before to sonograms, MRI, geoscience investigations to discover petrol—all these are examples where similar problems occur. Our technique, in principle, is applicable because the competition between low and high frequencies is common to all these problems, and so is the noise and limited signal. So we have high hopes that very soon clearer images will be obtained in all these diverse domains," they concluded.
More information: Mo Deng et al, Learning to synthesize: robust phase retrieval at low photon counts, Light: Science & Applications (2020). DOI: 10.1038/s41377-020-0267-2
Journal information: Light: Science & Applications
Provided by Chinese Academy of Sciences