Researchers use artificial neural networks to streamline materials testing
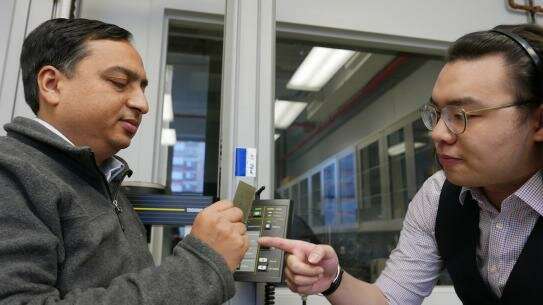
Optimizing advanced composites for specific end uses can be costly and time consuming, requiring manufacturers to test many samples to arrive at the best formulation. Investigators at the NYU Tandon School of Engineering have designed a machine learning system employing artificial neural networks (ANN) capable of extrapolating from data derived from just one sample, thereby quickly formulating and providing analytics on theoretical graphene-enhanced advanced composites.
The work, led by Nikhil Gupta, associate professor of mechanical and aerospace engineering at NYU Tandon, with Ph.D. student Xianbo Xu and collaborators at 2-D graphene materials manufacturer GrapheneCa, is detailed in "Artificial Neural Network Approach to Predict the Elastic Modulus from Dynamic Mechanical Analysis Results," which will be featured on the inside cover of the journal Advanced Theory and Simulations.
Tensile tests and dynamic mechanical analysis (DMA) are widely used to characterize the viscoelastic properties of materials at different loading rates and temperatures. But this requires an elaborate experimental campaign involving a large number of samples.
The Tandon team found a way to bypass this process by designing an ANN-based approach that builds a model and then feeds it data from DMA—a test of a material's response to a given temperature and loading frequency (a measure of load applied in cycles)—to predict how it will respond to any other temperature and pressure combination. Gupta explained that ANN extrapolated from measures of samples' ability to store and dissipate energy under different conditions.
"Testing materials under different conditions during the product development cycle is a major cost for manufacturers who are trying to create composites for numerous applications," noted Gupta. "This system allows us to conduct one test and then predict the properties under other conditions. It therefore considerably reduces the amount of experimentation needed."
"Applying an artificial neural network approach to predict the properties of nanocomposites can help in developing an approach where modeling can guide the material and application development and reduce the cost over time," continued Gupta.
"Working with the researchers at NYU Tandon's Department of Mechanical and Aerospace Engineering, we have developed a new method for predicting the behavior of thermosetting nanocomposites over a wide range of temperature and loading rates," said Dr. Sergey Voskresensky, Head of Research & Development at GrapheneCa's New York-based production facility. "Furthermore, the same approach can potentially be applied to predict a behavior of thermoplastic materials. This is a critical step towards advanced composite production."
More information: Xianbo Xu et al, Artificial Neural Network Approach to Predict the Elastic Modulus from Dynamic Mechanical Analysis Results, Advanced Theory and Simulations (2019). DOI: 10.1002/adts.201800131
Provided by NYU Tandon School of Engineering