Computer scientists and materials researchers collaborate to optimize steel classification
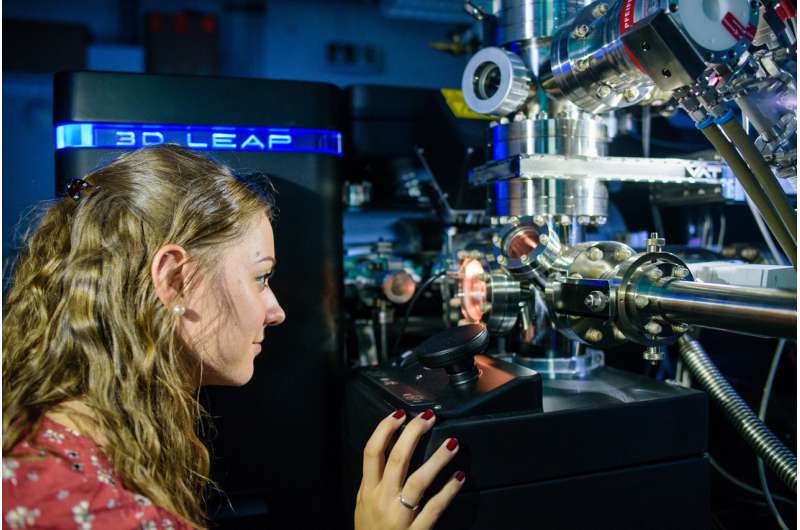
Using machine learning techniques, computer scientists and materials scientists in Saarbrücken have now developed a method that is much more accurate and objective than conventional quality control procedures. Their results have just been published in Scientific Reports.
When scientists from two disciplines collaborate on a research project, they first need to learn to speak the same language. "It took a fair amount of time before the computer scientists had understood why the internal structures of a material and their representation in image form play such an important role for materials scientists," says Dominik Britz, a Ph.D. student in the Department of Functional Materials at Saarland University. These internal structures are importance because they are very closely linked with the properties exhibited by the material.
"As modern steels are being supplied in ever-greater varieties and because they exhibit increasingly complex internal structures, error tolerances are becoming tighter. This poses a huge challenge for the engineers who develop new steels and who need to meet stringent quality requirements," says Britz.
Seyed Majid Azimi at the Max Planck Institute for Informatics sought to explain to Dominik Britz how the deep learning methods that he employs are able to produce significantly more accurate results than any of the image analyses conducted manually by expert materials scientists. To achieve such results, Azimi feeds his high-performance computer with image data that was previously classified by experts. This data is used to train the computer models, and these models are subsequently tested by comparing them against additional sets of human-classified image data. But how is it possible for the computer to generate such strikingly good results without having any real knowledge of the materials involved?
In this particular study, which focused on classifying steel microstructures, the answer lies in understanding the steel production process. "Manufacturing special steels is an extremely complex process that depends on many individual factors including the chemical composition of the material, the rolling process used and the types of heat treatment that the material is subjected to. Every stage of the production process influences the internal structure of the steel," explains Dominik Britz.
Materials scientists refer to this internal structure as the material's "microstructure." The microstructure is composed of "grains," each of which is a tiny crystallite with a particular crystal structure. But neighbouring grains also differ in terms of their spatial orientation. In fact, the grains differ not only in terms of their orientation, but also in terms of their individual shapes and their spatial connectivity, resulting in microstructures of high geometrical complexity. "These extremely complex structures can be made visible during the material development and the quality control stages by taking microscopic images. Specially prepared samples are evaluated using optical and electron microscopy," explains Britz.
Classifying a material involves comparing these microscope images with reference images that exhibit a typical geometrical microstructure. Over time, experienced engineers in company quality assurance departments develop a discerning eye that enables them to decide which particular steel microstructure they are dealing with. "But even these practised experts will sometimes make an incorrect call, as the differences between the images are sometimes barely discernible with the naked eye. Although humans are pretty good at distinguishing small relative differences, we are not very good at recognizing absolute geometric standards," explains Professor Frank Muecklich, who supervised the study. Muecklich is also Director of the Steinbeis Materials Engineering Center Saarland (MECS) in Saarbrücken whose staff were involved in the study.
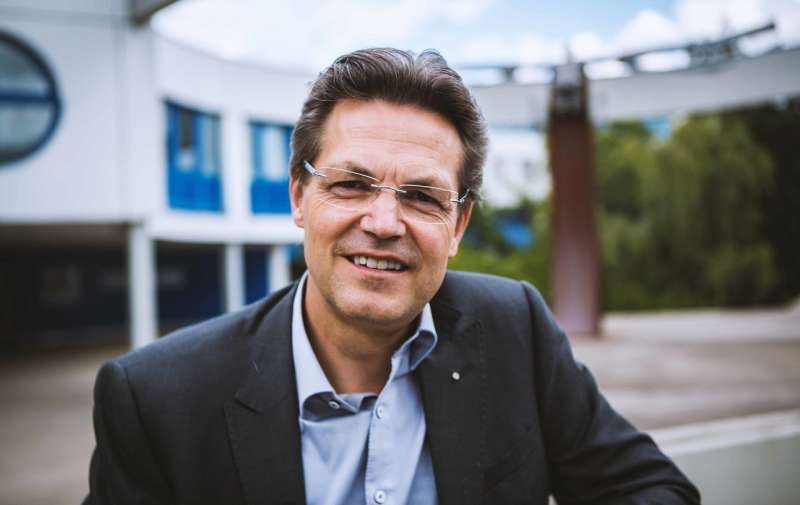
The materials scientists were interested in finding an objective procedure that was far less prone to user error and that could be applied irrespective of the user's level of expertise. "Machine learning methods allow computers to recognize complex patterns very rapidly and to assign the geometry of the microstructures in microscope images. They can learn the features of previously classified microstructures and compare these with recognized patterns," explains Muecklich. Using this approach, the research team in Saarbrücken was able to determine the microstructures of low-carbon steel at a level of accuracy that was not previously possible. "When using our system for microstructural classification, we achieved a level of accuracy of around 93 per cent. With conventional methods, only about 50 per cent of the material samples are correctly classified," says Muecklich.
The results are published in Scientific Reports.
More information: Seyed Majid Azimi et al, Advanced Steel Microstructural Classification by Deep Learning Methods, Scientific Reports (2018). DOI: 10.1038/s41598-018-20037-5
Journal information: Scientific Reports , Nature
Provided by Saarland University