Using neural networks to predict outcomes of organic chemistry
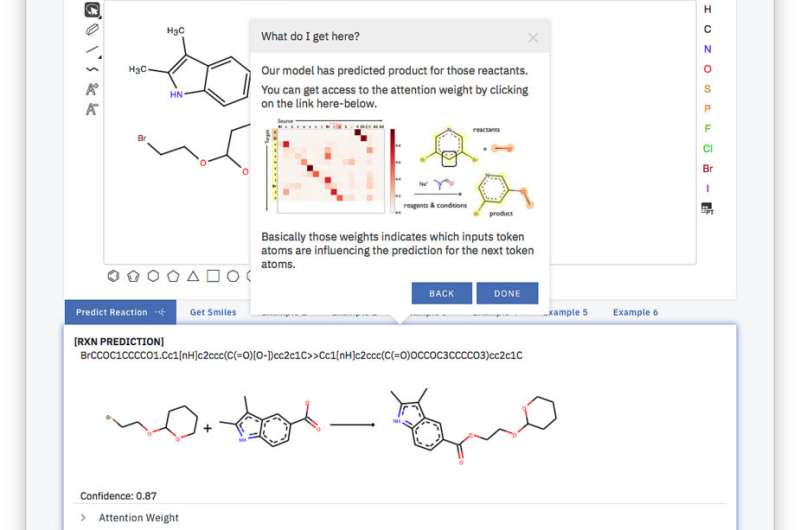
For more than 200 years, the synthesis of organic molecules remains one of the most important tasks in organic chemistry. The work of chemists has scientific and commercial implications that range from the production of Aspirin to that of Nylon. Yet, little has been done to dramatically change ages old practices and allow a new era of productivity based on pioneering artificial intelligence (AI) science and technologies.
The challenge for organic chemists in fields such as chemistry, materials science, oil and gas, and life sciences is that there are hundreds of thousands of reactions and, while it is manageable to remember a few dozen in a narrow specialist's field, it's impossible to be an expert generalist.
To address this we asked ourselves, can we use deep learning and artificial intelligence to predict reactions of organic compounds?
First, since we studied engineering and material sciences, but not organic chemistry, we had to hit the books. It wasn't long before we started seeing organic chemistry everywhere—morning, noon and night. Atoms appeared instead of letters, molecules materialized from words and, then, something incredible happened: an idea was born.
We realized that organic chemistry datasets and language datasets have a lot in common: they both depend on grammar, on long range dependencies, and a small particle or word like "not" can change the entire meaning of a sentence just like the stereochemistry can turn Thalidomide into either a medication or a deadly poison.
As non-native English speakers we are both familiar with online translation tools, which were work wonders in turning English to French, and German to English, so why not try to use them to turn random chemicals into functional compounds?
At the NIPS 2017 Conference we present our results: a web-based app which takes the idea of relating organic chemistry to a language and applies state-of-the-art neural machine translation methods to go from designing materials to generating products using sequence-to-sequence (seq2seq) models.
Chemistry 101
Back in high school, we had to draw by hand the hexagons and pentagons and all the various lines representing bonds of organic molecules. Now we've brought up a system that takes the exact same representation and can predict how molecules will react within a click.
The overall tool is simple, and the model is trained end-to-end, fully data-driven and without to aid of querying a database or any additional external information. With this approach, we outperform current solutions using their own training and test sets by achieving a top-1 accuracy of 80.3 percent and set a first score of 65.4 percent on a noisy single product reactions dataset extracted from US patents.
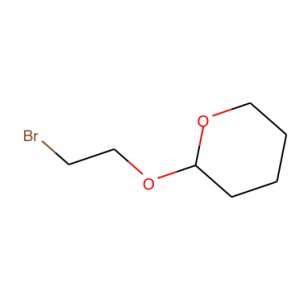
The secret behind our tool is what is called a simplified molecular-input line-entry system or SMILES. SMILES represents a molecule as a sequence of character. For instance, the image on the right, becomes BrCCOC1OCCCC1.
We trained our model using an openly available chemical reaction dataset, which correspond to 1 million patent reactions.
In the future, we aim to enhance the model and improve our accuracy by expanding our dataset. Currently our data is taken from information publicly available in US patents published online, but there is no reason why the tool couldn't be trained on data coming from other sources, such as chemistry text books and scientific publications.
We also plan to make this tool publicly available for free on the cloud in early 2018.
Sign up at www.zurich.ibm.com/foundintranslation to receive an alert when the web-tool is ready.
Provided by IBM