Researchers work to predict the future of transit
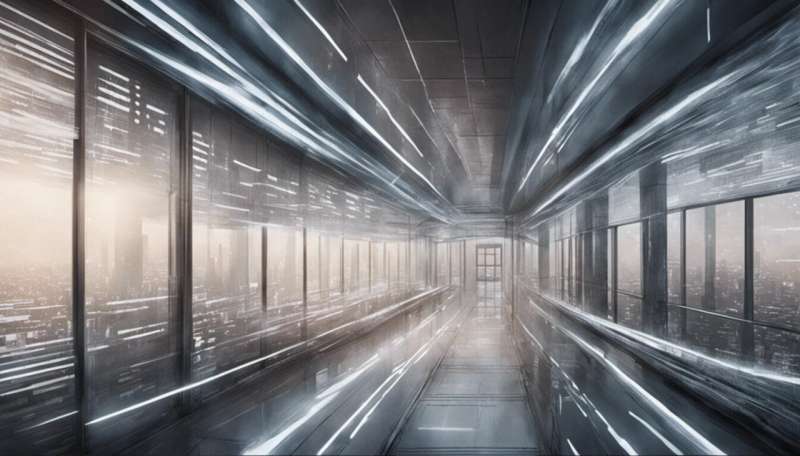
Haris N. Koutsopoulos believes that our ability to predict the future has the potential to enable various innovations in public transit. The short-term future, that is.
Is a subway station or train about to get overcrowded during rush hour? Is an impending storm about to wreak havoc on the system, or is a bus station about to be flooded with fans leaving a baseball game or concert? Should I, as a commuter, consider taking an alternative route home or leaving work a bit later?
These are the types of public transit questions Koutsopoulos, professor of civil and environmental engineering at Northeastern, is focused on addressing by way of real-time predictive analysis. In one project involving Transport for London—the body that oversees London's transit system, including the London Underground, which is one of the world's busiest metro systems—he and Peyman Noursalehi, one of his doctoral students, are developing real-time predictive models that forecast subway transit activity 15 or 30 minutes into the future. Such models, he explains, are based upon analyses of large swaths of automated fare-collection data that can reveal past travel patterns as well as real-time factors such as weather, events, and eventually, even social media chatter. Koutsopoulos' team has developed an initial prototype of a predictive model as well as an accompanying visualization tool.
"A lot of the work with this data has basically looked at what happened yesterday, where passengers enter and where they exit the system," Koutsopoulos says. "Now what we're thinking about is what can we learn from the travel patterns in all this data and using what we learn from the past to make short-term predictions about the future. It's about being proactive, not reactive. For example, sometimes in those systems, if a station gets too crowded, the gates are closed and passengers aren't allowed to come in until the crowd subsides. This is reactive. But if you can predict that demand will increase in the near future, maybe you can take action earlier and prevent the problem from becoming bigger later on."
The goal of Koutsopoulos' research is to develop tools that help transit operators optimally manage their systems and commuters make informed trip decisions. For example, he says, predictive analytics could be used for real-time gate management to prevent overcrowding at major stations or feed into a transit app that sends real-time predictive information to riders.
The London project is part of Koutsopoulos' work in the MIT-NEU Transit Lab, a collaboration between Northeastern and the Massachusetts Institute of Technology. In addition to Transport for London, Koutsopoulos and his doctoral students are studying how passengers on the MTR in Hong Kong use the underground transit system with the goal of developing strategies to alleviate congestion in the main parts of the network. These strategies include helping operators improve crowd management and incentivizing riders to alter their travel patterns.
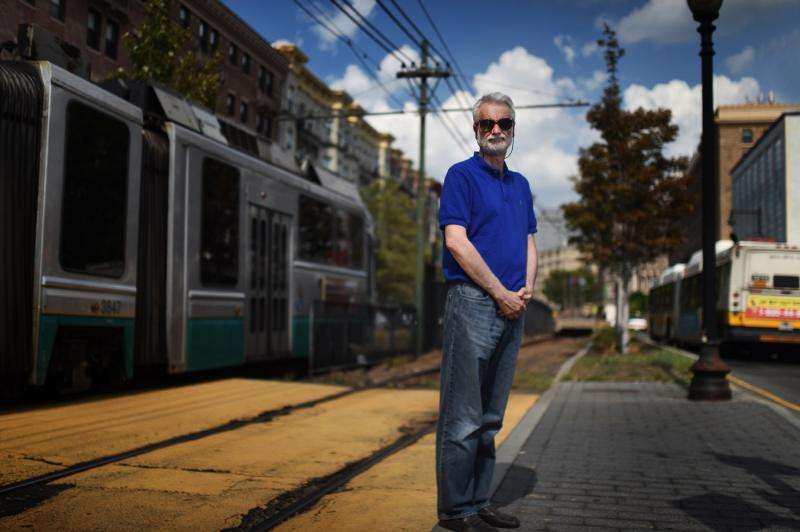
Koutsopoulos and his students are also pursuing separate, but related, research focused on observing how different riders use a transit system and then inferring underlying traits about these travel behaviors. By clustering riders into different groups based on these behaviors, he explains, you can better understand ridership patterns—and therefore improve your predictive models.
A traffic simulation pioneer
His work represents an example of the next-generation of how we think about transportation—using Big Data to make informed decisions about how, when, and where people move. Koutsopoulos describes his research as being focused primarily on intelligent transportation systems. As he puts it, "The idea is to use technology to improve how well we use the capacity that is actually available in the system to minimize inefficiencies."
Koutsopoulos has been a pioneer in the field of traffic simulation modeling for more than 20 years, and earlier this year he was honored with the Traffic Simulation Lifetime Achievement Award by the Transportation Research Board.
Prior to joining Northeastern in 2014, Koutsopoulos founded the iMobility lab at the KTH Royal Institute of Technology in Stockholm, where he used real-time GPS data from taxis to develop traffic management and prediction tools for local authorities.
As a globally renowned researcher in this field, Koutsopoulos recently co-hosted an international conference at Northeastern, called TransitData2016, where scholars and transportation officials worldwide convened to discuss new research and advancements centered on using data from automated sources to improve planning and operations at public transit systems. He noted that three trends he observed were more discussion about data fusion—using data from a variety of sources to make transportation management, evidence based decisions—increased use of visualization tools by researchers and practitioners to better communicate how transportation systems are functioning, and increased interest by agencies in data warehouses and open data.
"We view all the work we're doing with transit agencies as building blocks that they can use to improve service, be more responsive, communicate better, plan their systems better, and overall be more competitive in providing mobility options," Koutsopoulos says.
Provided by Northeastern University