Developing methods for quantifying uncertainty and sensitivity for complex systems
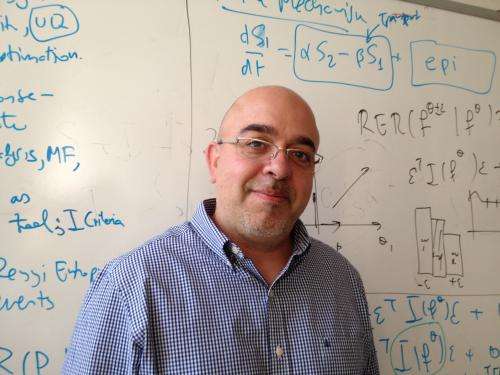
Applied mathematicians Markos Katsoulakis and Luc Rey-Bellet of the University of Massachusetts Amherst will share a three-year, $2.3 million grant from the U.S. Department of Energy, with others, to develop new methods to assess and improve mathematical modeling of multi-scale, complex systems. Once developed, the new methods are expected to have applications in energy research and materials synthesis.
As Katsoulakis explains, predictive mathematical models and algorithms have long complemented theory and experiments in applied sciences and engineering, but such computational models are now more important than ever because of the increased complexity of the problems plus advances in computing capabilities. A recognition of the vast predictive potential of modeling and efficient simulation of complex systems, he points out, is the fact that the 2013 Nobel Prize in chemistry was awarded for the development of multi-scale models for complex chemical systems.
One of the primary practical applications planned by Katsoulakis and Rey-Bellet, with partners at Brown University and the University of Delaware, is to design highly efficient and cost-effective bimetallic catalysts using relatively inexpensive metals, allowing storage and production of clean hydrogen fuel from readily available sources such as ammonia.
Katsoulakis says, "The role of uncertainty and sensitivity quantification in this process turns out to be crucial, because the design of bimetallic catalysts rests on understanding how sensitive the catalyst's performance metrics are on its parent metals. Also, experiments have shown that performance depends on the micro-geometry of the arrangement of the two metals, that is structure and ordering of their layers. Given all the choices we have in selecting materials and geometries for the two-metal catalysts, this becomes a very complex system to model."
Being able to systematically evaluate which metal combinations in the catalyst are the most efficient and cost effective is one of the team's key goals. The challenge is an example of a model where new mathematical and computational techniques for assessing uncertainty and quantifying sensitivity can be extremely productive, the researchers say.
Over the next three years, the multi-institution team will develop new mathematical tools that describe uncertainty and model sensitivity using information theory, probability theory, statistical methods such as model selection and model reduction, rare events methods, multi-scale analysis and parameterization of coarse-grained models from finer scales and data.
Mathematical models are now routinely being asked to account for systems of increasing complexity, that is handling millions or even billions of variables. In addition, a model must integrate data from different scales and must account for different spatial scales, for example from the molecular level all the way to the everyday macroscopic scale, and basic physical processes at different time scales.
The UMass Amherst mathematician says, "Interactions across scales are a unifying feature in all complex systems that we may experience in everyday life. Think of the effect that a single vehicle breakdown during rush hour may have to the overall traffic flow, even at very large distances from the scene." Taking all the different variables and mechanisms such as vehicle speeds, sizes, road network, weather, traffic volume and so on into account, represents a typical complex "multi-scale multi-physics" modeling, simulation and analysis problem that challenges current applied mathematical methods, he adds.
Handling real-life systems with unprecedented levels of complexity and multi-scale features requires not only more powerful computational capabilities, but also new mathematics, Katsoulakis explains. "Though high performance computing can allow us, for the first time, to simulate at least some complex systems, there are important concerns related to the effectiveness and reliability of the predictive computational models."
As in the traffic example, all such models depend on a large number of mechanisms and parameters but it is not immediately obvious which ones critically affect the final predictions and which ones can be ignored. Another, closely related, source of uncertainty is insufficient knowledge of a particular highly complex system.
Katsoulakis and Rey-Bellet believe that their research has great potential for wider impact in a number of fields because it will lay down the mathematical foundations for uncertainty quantification and sensitivity analysis in a broad class of complex systems typically encountered in physicochemical and biological processes, atmosphere and ocean science, and other types of complex networks.
Besides Katsoulakis and Rey-Bellet at UMass Amherst, collaborators are mathematician Petr Plechac and chemical engineer Dion Vlachos at the University of Delaware and applied mathematician Paul Dupuis at Brown University.
Provided by University of Massachusetts Amherst