April 13, 2024 feature
This article has been reviewed according to Science X's editorial process and policies. Editors have highlighted the following attributes while ensuring the content's credibility:
fact-checked
peer-reviewed publication
trusted source
proofread
A machine learning-based approach to discover nanocomposite films for biodegradable plastic alternatives
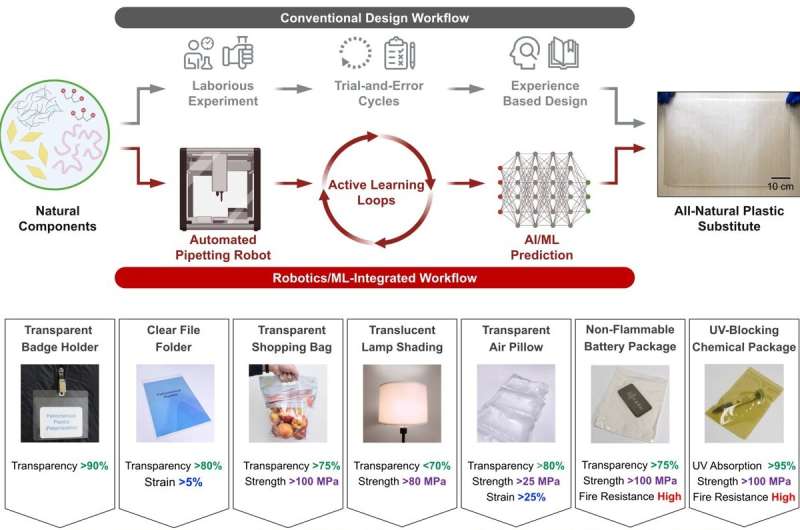
The accumulation of plastic waste in natural environments is of utmost concern, as it is contributing to the destruction of ecosystems and is causing harm to aquatic life. In recent years, material scientists have thus been trying to identify all-natural alternatives to plastic that could be used to package or manufacture products.
Researchers at University of Maryland, College Park, recently devised a new approach to discover promising biodegradable plastic alternatives. Their proposed method, outlined in a paper published in Nature Nanotechnology, combines state-of-the-art machine learning techniques with molecular science.
"My inspiration for this research was sparked by a 2019 visit to Palau in the Western Pacific," Prof. Po-Yen Chen, co-author of the paper, told Tech Xplore. "The impact of plastic pollution on marine life there—floating plastic films deceiving fish and sea turtles mistaking plastic waste for food—was deeply disturbing. This motivated me to apply my expertise to this environmental issue and led to my focus on finding a solution when setting up my research lab at UMD."
Conventional and previously employed methods to search for sustainable plastic alternatives are time-consuming and inefficient. In many cases, they also yield poor results, for instance, identifying materials that are biodegradable but do not have the same desirable properties as plastic.
The innovative approach for identifying plastic alternatives introduced in this recent paper relies on a machine learning model developed by Chen.
In addition to being faster than conventional methods of searching for materials, this approach could be more effective in discovering materials that can be realistically employed in manufacturing and industry settings. Chen applied his machine learning technique to the discovery of all-plastic alternatives in close collaboration with his colleagues Teng Li and Liangbing Hu.
"Combining automated robotics, machine learning, and molecular dynamics simulations, we accelerated the development of environmentally friendly, all-natural plastic substitutes that meet essential performance standards," Chen explained. "Our integrated approach combines automated robotics, machine learning, and active learning loops to expedite the development of biodegradable plastic alternatives."
First, Chen and his colleagues compiled a comprehensive library of nanocomposite films derived from various natural sources. This was done using an autonomous pipetting robot, which can independently prepare laboratory samples.
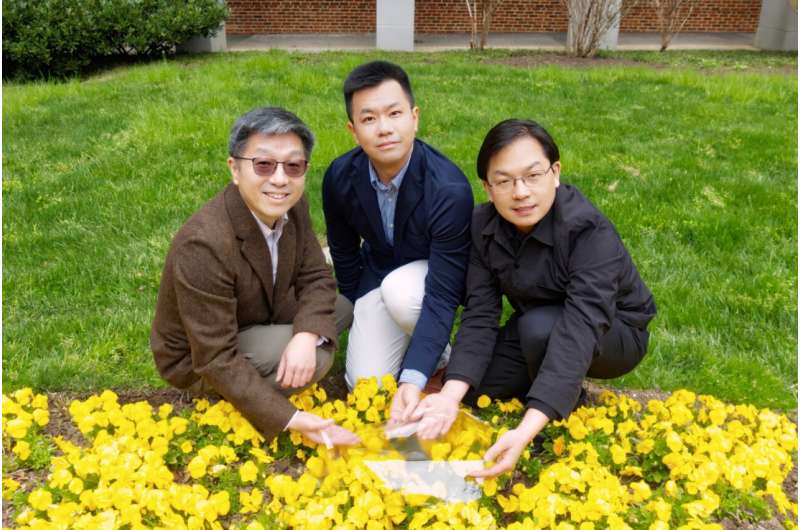
Subsequently, the researchers used this sample library to train Chen's machine learning-based model. During training, the model gradually became more proficient in predicting the properties of materials based on their composition, through a process known as iterative active learning.
"The synergy of robotics and machine learning not only expedites the discovery of natural plastic substitutes but also allows for the targeted design of plastic alternatives with specific properties," Chen said. "Our approach significantly reduces the time and resources required, compared to the traditional trial-and-error research method."
This recent study and the approach it introduced could expedite the future search for eco-friendly plastic alternatives. The team's model could soon be used by teams worldwide to produce all-natural nanocomposites with adjustable and advantageous properties.
"By coupling robotics, machine learning, and simulation tools, we have established a workflow that accelerates the discovery of new functional materials and enables customization for specific applications," Chen said.
"Our integrated approach lowers the design barrier for a green alternative to petrochemical plastics while remaining environmentally safe. It also provides an open and expandable database focused on green, eco-friendly, and biodegradable functional materials."
In the future, the innovative approach developed by Chen could help to reduce plastic pollution worldwide, by facilitating the transition of multiple sectors towards more sustainable materials. In their next studies, the researchers plan to continue working to address the environmental issues caused by petrochemical plastics.
For instance, they hope to expand the range of natural materials that manufacturers can choose from. In addition, they will try to broaden the possible applications of materials identified by their model and ensure that these materials can be produced on a large scale.
"We are now working on finding the right biodegradable and sustainable materials for packaging fresh produce after harvest, replacing single-use plastic food packaging, and improving the shelf life of these post-harvest products," Chen added.
"We are also investigating how to manage the disposal of these biodegradable plastics, including recycling them or converting them into other useful chemicals. These efforts are crucial steps toward making our solutions not only environmentally friendly but also economically viable alternatives to conventional plastics. This work contributes significantly to the worldwide initiative to reduce plastic pollution."
More information: Tianle Chen et al, Machine intelligence-accelerated discovery of all-natural plastic substitutes, Nature Nanotechnology (2024). DOI: 10.1038/s41565-024-01635-z
Journal information: Nature Nanotechnology
© 2024 Science X Network