This article has been reviewed according to Science X's editorial process and policies. Editors have highlighted the following attributes while ensuring the content's credibility:
fact-checked
peer-reviewed publication
trusted source
proofread
Unifying behavioral analysis through animal foundation models
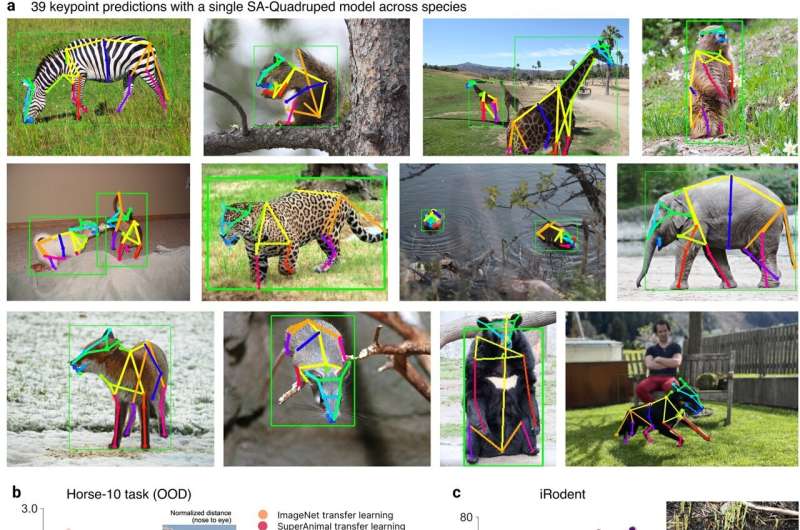
Although there is the saying, "straight from the horse's mouth," it's impossible to get a horse to tell you if it's in pain or experiencing joy. Yet, its body will express the answer in its movements. To a trained eye, pain will manifest as a change in gait, or in the case of joy, the facial expressions of the animal could change. But what if we can automate this with AI? And what about AI models for cows, dogs, cats, or even mice?
Automating animal behavior not only removes observer bias, but it helps humans more efficiently get to the right answer.
A new study marks the beginning of a new chapter in posture analysis for behavioral phenotyping. Mackenzie Mathis' laboratory at EPFL has published a Nature Communications article describing a particularly effective new open-source tool that requires no human annotations to get the model to track animals.
Named "SuperAnimal," it can automatically recognize, without human supervision, the location of "keypoints" (typically joints) in a whole range of animals—over 45 animal species—and even in mythical ones.
"The current pipeline allows users to tailor deep learning models, but this then relies on human effort to identify keypoints on each animal to create a training set," explains Mathis.
"This leads to duplicated labeling efforts across researchers and can lead to different semantic labels for the same keypoints, making merging data to train large foundation models very challenging. Our new method provides a new approach to standardize this process and train large-scale datasets. It also makes labeling 10 to 100 times more effective than current tools."
The "SuperAnimal method" is an evolution of a pose estimation technique that Mathis' laboratory had already distributed under the name "DeepLabCut️."
"Here, we have developed an algorithm capable of compiling a large set of annotations across databases and train the model to learn a harmonized language—we call this pre-training the foundation model," explains Shaokai Ye, a Ph.D. student researcher and first author of the study. "Then users can simply deploy our base model or fine-tune it on their own data, allowing for further customization if needed."
These advances will make motion analysis much more accessible. "Veterinarians could be particularly interested, as well as those in biomedical research—especially when it comes to observing the behavior of laboratory mice. But it can go further," says Mathis, mentioning neuroscience and... athletes (canine or otherwise). Other species—birds, fish, and insects—are also within the scope of the model's next evolution.
"We also will leverage these models in natural language interfaces to build even more accessible and next-generation tools. For example, Shaokai and I, along with our co-authors at EPFL, recently developed AmadeusGPT, published recently at NeurIPS, that allows for querying video data with written or spoken text."
"Expanding this for complex behavioral analysis will be very exciting."
SuperAnimal is now available to researchers worldwide through its open-source distribution (github.com/DeepLabCut).
More information: SuperAnimal pretrained pose estimation models for behavioral analysis, Nature Communications (2024). DOI: 10.1038/s41467-024-48792-2
Journal information: Nature Communications
Provided by Ecole Polytechnique Federale de Lausanne