This article has been reviewed according to Science X's editorial process and policies. Editors have highlighted the following attributes while ensuring the content's credibility:
fact-checked
peer-reviewed publication
trusted source
proofread
Novel neural network framework advances large-scale simulations of zeolites
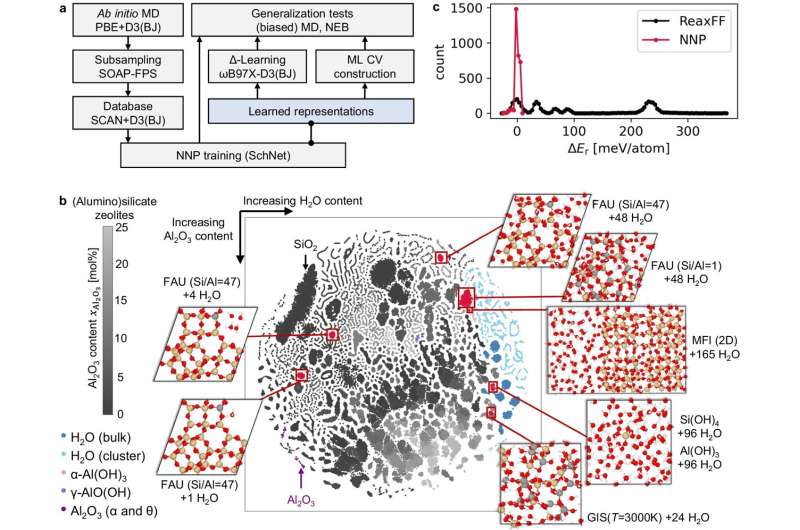
The Nanomaterials Modeling group of Dr. Grajciar and Dr. Heard from the Faculty of Science, Charles University develops and applies a range of computational methods to study materials with significant industrial potential as well as existing industrially exploited materials with the aim of their optimization.
They have established a novel machine-learning based framework allowing for comprehensive investigation of such materials under operating conditions. Their results have been published in Nature Communications.
Zeolites are a class of microporous aluminosilicates with tremendous structural and chemical diversity, which originates from the myriad stable three-dimensional arrangements of covalently connected silica/alumina tetrahedra. This makes zeolites a versatile material class with applications ranging from thermal energy storage to gas separation and water purification, but predominantly in heterogeneous catalysis.
However, up to now, a comprehensive exploration of their enormous structural and chemical diversity has been based largely on a trial-and-error experimental approach and on simplified theoretical models.
With the advent of machine learning, the window of opportunity is open for both a huge acceleration of the computational simulations and the adoption of much more realistic and complex models of the (catalytic) materials. This is what the group of Dr. Grajciar and Dr. Heard tapped into, developing a model based on convolutional neural networks that are capable of accelerating the atomistic simulations of various classes of materials by orders of magnitude.
In particular, they focused on the extremely important class of proton-exchanged aluminosilicate zeolites, which are one of the cornerstones of existing petrochemical processes, being produced at the Megaton scale, as well as one of the main candidates for emerging applications in sustainable chemistry.
Importantly, besides the acceleration of the atomistic simulations, the machine-learning models were shown to be able to discover hitherto unseen chemical processes and species in these materials. Moreover, it was also exemplified how these baseline neural network models can be extended to improve accuracy and sampling efficiency further in combination with other advanced machine learning–based tools.
In summary, the ML-based framework introduced in the work of the Nanomaterials Modeling group represents a big step towards large-scale simulations of an extremely important class of catalytic materials—zeolites—tackling long-lasting challenges in the field, ranging from understanding the mechanistic underpinnings of zeolite hydrothermal (in)stability to the determination of the character of active species and defects under operating conditions.
This work represents an important use case of the potential of machine learning for rational materials design.
More information: Andreas Erlebach et al, A reactive neural network framework for water-loaded acidic zeolites, Nature Communications (2024). DOI: 10.1038/s41467-024-48609-2
Journal information: Nature Communications
Provided by Charles University