This article has been reviewed according to Science X's editorial process and policies. Editors have highlighted the following attributes while ensuring the content's credibility:
fact-checked
peer-reviewed publication
trusted source
proofread
Developing a machine learning model to explore DNA methylation
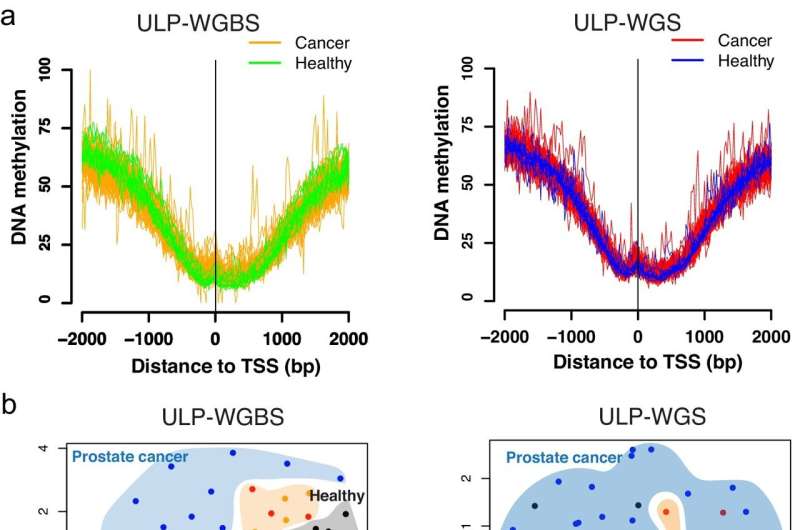
A Northwestern Medicine study has detailed the development of a machine learning model to predict DNA methylation status in cell-free DNA by its fragmentation patterns, according to findings published in Nature Communications.
DNA methylation, the biological process by which methyl groups are added to a DNA molecule, functions as an "off switch" for certain genes and is commonly dysfunctional in diseases such as cancer.
Cell-free DNA—small amounts of DNA leftover from various cellular processes—can be measured by whole-genome bisulfite sequencing, the current gold standard, but an imperfect process that can damage the DNA being sequenced, limiting scientists' ability to study it.
"Cell-free DNA are these short DNA fragments: When a cell is dying, it will release the DNA to the blood," said Yaping Liu, Ph.D., assistant professor of Biochemistry and Molecular Genetics, who was first and a co-corresponding author of the study. "This cell-free DNA, which is outside the cell, represents the cell death signatures."
Unlike normal DNA, cell-free DNA breaks apart in specific patterns and is highly correlated with the epigenetic status, which led Liu to wonder if he could use cell-free DNA fragmentation patterns to predict the levels of DNA methylation, he said.
In the study, Liu and his collaborators trained an unsupervised machine learning model to analyze small sections of DNA, called CpG sites, using characteristics from the circulating cell-free DNA fragments.
The investigators then used the model to analyze human blood samples from healthy patients and those with different types of cancer and performed separate whole-genome sequencing on the samples to compare the model's accuracy.
The model accurately predicted DNA methylation status mostly at the CpG rich regions on the genome compared to traditional sequencing, according to the study.
"Clinicians already generate a lot of cell-free DNA genomic sequencing data with tests available today," Liu said. "With our model, we can do more with that data and predict DNA methylation and the changes happening in our genes."
The model could also accurately predict which tissues the cell-free DNA came from, thereby pinpointing the origin of abnormal methylation signatures which occur in various cancers, Liu said.
Moving forward, Liu's laboratory will continue to develop computational methods to better understand gene regulation information from cell-free DNA fragments, he said.
"Our goal is to use the epigenetic information hidden in the cell-free DNA to understand the non-coding regions of the human genome," said Liu, who is also a member of the Robert H. Lurie Comprehensive Cancer Center of Northwestern University. "We want to not only detect disease earlier but also get the opportunity to understand what's happening in the genome at that time point."
More information: Yaping Liu et al, FinaleMe: Predicting DNA methylation by the fragmentation patterns of plasma cell-free DNA, Nature Communications (2024). DOI: 10.1038/s41467-024-47196-6
Journal information: Nature Communications
Provided by Northwestern University