This article has been reviewed according to Science X's editorial process and policies. Editors have highlighted the following attributes while ensuring the content's credibility:
fact-checked
peer-reviewed publication
trusted source
proofread
Automated bioacoustics: Researchers are listening in on insects to better gauge environmental health
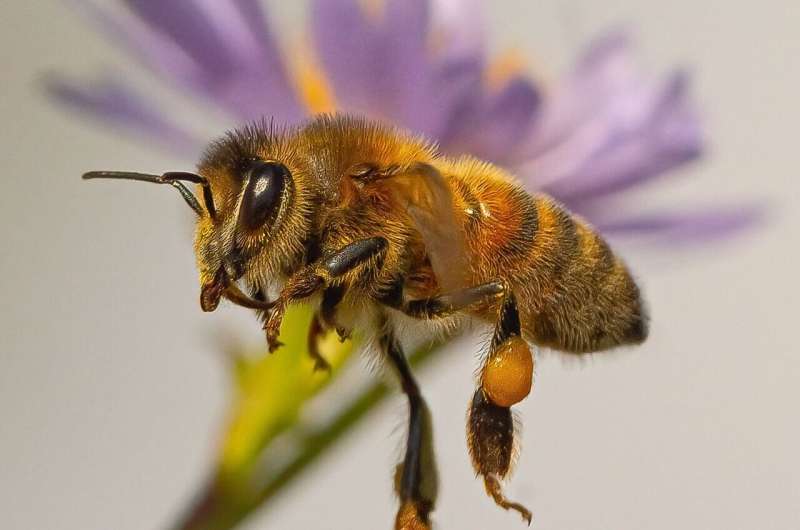
Recent research led by the University of Massachusetts Amherst evaluates how well machine learning can identify different insect species by their sound, from malaria-carrying mosquitoes and grain-hungry weevils to crop-pollinating bees and sap-sucking cicadas.
Listening in on the insect world gives us a way to monitor how populations of insects are shifting, and so can tell us about the overall health of the environment. The study, published in the Journal of Applied Ecology, suggests that machine and deep learning are becoming the gold standards for automated bioacoustics modeling, and that ecologists and machine-learning experts can fruitfully work together to develop the technology's full potential.
"Insects rule the world," says Laura Figueroa, assistant professor of environmental conservation at UMass Amherst and the paper's senior author. "Some are disease vectors and pests, while others pollinate nutritious crops and cycle nutrients. They're the foundation of ecosystems around the world, being food for animals ranging from birds and fishes to bears and humans. Everywhere we look, there are insects, but it's difficult to get a sense of how their populations are changing."
Indeed, in the age of chemical pesticides, climate change and other environmental stressors, insect populations are changing drastically. Some species—like the pollinators that are annually responsible for ecosystem services estimated at well over $200 billion worldwide—seem to be crashing, while others, like mosquitoes that can carry malaria, dengue and other diseases, seem to be surging. Yet it can be difficult to get an accurate picture how insect populations are shifting.
Many traditional methods of sampling insect populations involve sending entomologists out into the field to collect and identify individual species, and while these methods can yield reliable results, it's also time and resource intensive and often lethal to the insects that get caught. This is where AI comes into the picture.
"After working in the field for over a decade, I can tell the difference between a bee's buzz and a fly's buzz," says Figueroa. "Since many, but not all, insects emit sound, we should be able train AI models to identify them by the unique sounds they make."
In fact, such training is already happening—but which AI methods are best?
To answer this question, Figueroa and her colleagues, including lead author Anna Kohlberg, who completed this research while working in the Figueroa lab, conducted a systematic literature review to analyze studies that used different kinds of automated bioacoustics models to identify insects. They found models for 302 different species spread across nine taxonomic orders. They broke the resulting models down into three broad categories: non-machine learning, machine learning and deep learning.
The non-machine learning models match insect calls to specific markers that human researchers designate as keys for identification, such as a particular frequency band in a katydid's call. The model then "listens" for those specific, human-designated cues.
Machine learning, on the other hand, has no pre-ordained set of markers that it uses and instead relies on a flexible computational framework to find relevant patterns in the sounds, then matches those patterns to bioacoustics data that it has been trained on.
Deep learning, a specialized kind of machine learning, relies on more advanced neural computational frameworks that give the model more flexibility in effectively identifying relevant bioacoustics patterns. As it turns out, the models relying on deep learning are the most successful. Some of the best can classify hundreds of species with more than 90% accuracy.
"This doesn't mean that AI can or should replace all traditional monitoring approaches," says Kohlberg, and there are limitations in what they can do. Most of the models need huge sets of data to train on, and while they are getting better at working with smaller data sets, they remain data-intensive tools. Furthermore, not all insects emit sounds—such as aphids. And very noisy contexts, like an urban environment, can easily confuse sound-based monitoring efforts.
"Automated bioacoustics is a key tool in a multifaceted toolkit that we can use to effectively monitor these important organisms all over the world," says Kohlberg.
More information: From buzzes to bytes: A systematic review of automated bioacoustics models used to detect, classify, and monitor insects, Journal of Applied Ecology (2024). DOI: 10.1111/1365-2664.14630. besjournals.onlinelibrary.wile … 1111/1365-2664.14630
Journal information: Journal of Applied Ecology
Provided by University of Massachusetts Amherst