This article has been reviewed according to Science X's editorial process and policies. Editors have highlighted the following attributes while ensuring the content's credibility:
fact-checked
trusted source
proofread
Building ChatGPT-style tools with Earth observation
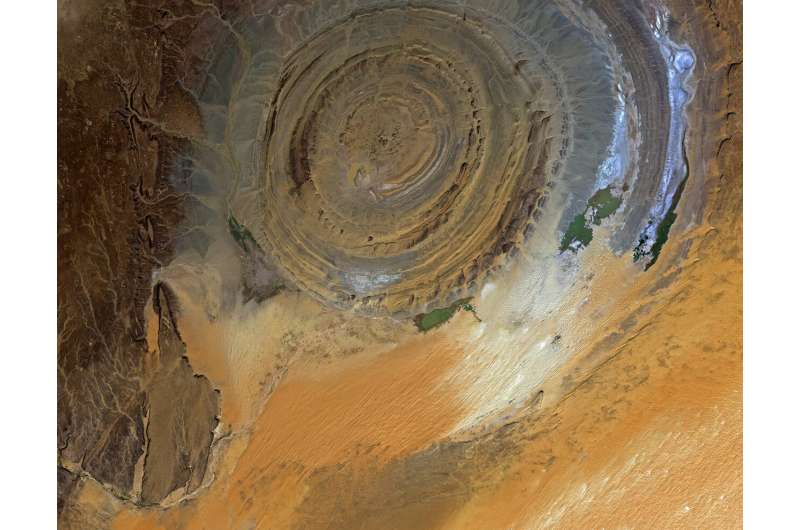
Imagine being able to ask a chatbot, "Can you make me an extremely accurate classification map of crop cultivation in Kenya?" or "Are buildings subsiding in my street?" And imagine that the information that comes back is scientifically sound and based on verified Earth observation data.
ESA, in conjunction with technology partners, is working to make such a tool a reality by developing AI applications that will revolutionize information retrieval in Earth observation.
A digital helping hand for data
Earth observation generates vast volumes of vital data every day, but it is difficult for humans alone to ensure that we obtain the best value from that data. Fortunately, AI helps in interacting with such large and complex datasets, identifying key features and presenting the information in a user-friendly format.
I*STAR for example, an activity co-funded by the ESA InCubed program, developed a platform that uses AI to monitor current events like earthquakes or volcano eruptions so that satellite operators can automatically plan the next data acquisitions for customers.
The SaferPlaces AI tool, again supported by InCubed, creates flood maps for disaster response teams by merging in situ measurements with satellite data. SaferPlaces was crucial to damage assessment efforts during last year's floods in Emilia-Romagna in Italy.
In the last few years, the progress of AI has accelerated tremendously, with the advance of tools such as ChatGPT and Gemini even surprising experts in the field. To take advantage of this transformative innovation and capture the opportunities enabled by this technology, a natural next step is to build a ChatGPT-style text-based enquiry with Earth observation data.
Along with various partners from the fields of space, computing and meteorology, ESA is currently developing an Earth observation digital assistant that will understand human queries and respond with human-like answers—known as natural language capabilities.
Not surprisingly though, there are a number of pieces of the jigsaw puzzle to complete to create such a digital assistant, starting with the powerhouse that underpins it, the foundation model.
The motor roaring under the bonnet
AI models work by training and improving over time, but in more traditional machine learning, the machine has to be fed with large sets of data that have been labeled, often by a human.
Enter foundation models, which take a very different approach. A foundation model is a machine learning model that trains, largely without human supervision, on sizeable and varied sources of unlabelled data. Foundation models are quite general, but can be tailored to specific applications.
The result is a flexible, powerful AI engine, and since their inception in 2018 foundation models have contributed to a huge transformation in machine learning, impacting many industries and society as a whole.
ESA Φ-lab has several ongoing initiatives for creating foundation models dedicated to Earth observation-related tasks. These models use data to provide information on environmentally critical topics such as methane leaks and extreme-weather-event mitigation.
One foundation model project, PhilEO, started at the beginning of 2023 and is now reaching maturity. An evaluation framework based on global Copernicus Sentinel-2 data, and soon the PhilEO model itself, are being released to the Earth observation community in order to stimulate a collaborative approach, advance development in the field and ensure the derived foundation model is extensively validated.
The image above shows the Richat Structure, the type of feature that the PhilEO model has learnt to recognize without human supervision.
The human interface
Separate ESA initiatives are looking into the human end of the jigsaw puzzle—creating the digital assistant that will take a natural language question from a user, process the right data through Earth observation foundation models and produce the answer in text and/or images.
A precursor digital twin of Earth has recently demonstrated that its digital assistant prototype can carry out multimodal tasks, searching among multiple data archives such as Sentinel-1 and 2 to compare information.
An ESA Φ-lab activity due to start in April will explore natural language processing for extracting and analyzing information from verified Earth observation text sources, together with interpreting queries from both experts and general users. This activity will ultimately lead to the creation of a fully functioning digital assistant.
"The concept of an Earth observation digital assistant that can provide a broad range of insight from varied sources is a tantalizing prospect, and as these initiatives show, there are a number of fundamental building blocks to put in place to achieve that aim," comments Head of ESA Φ-lab Giuseppe Borghi.
"Given the extremely encouraging progress already achieved with PhilEO and the digital assistant precursor, I fully expect the new projects to yield game-changing results in the near future."
Provided by European Space Agency