This article has been reviewed according to Science X's editorial process and policies. Editors have highlighted the following attributes while ensuring the content's credibility:
fact-checked
trusted source
proofread
Bridging the gap: Computer scientists develop model to enhance water data from satellites
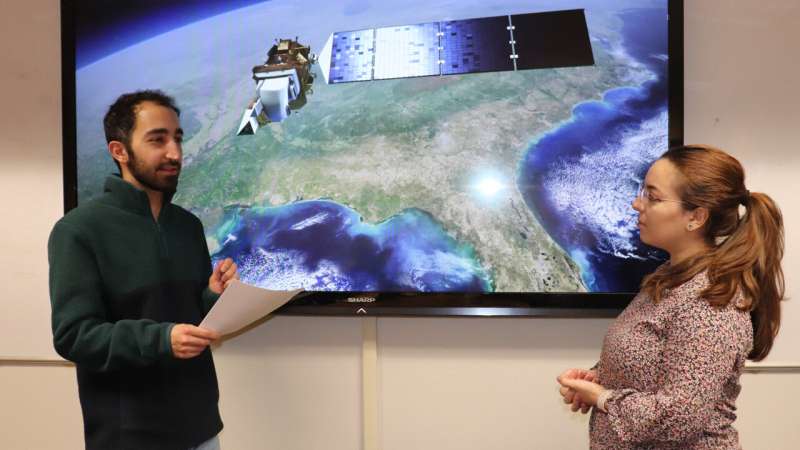
Satellites encircling the Earth collect a bounty of water data about our planet, yet distilling usable information from these sources about our oceans, lakes, rivers and streams can be a challenge.
"Water managers need accurate data for water resource management tasks, including lake coastal zone monitoring, rising seas border shift detection and erosion monitoring," says Utah State University computer scientist Pouya Hosseinzadeh. "But they face a trade-off when reviewing data from currently deployed satellites, which yield complementary data that are either of high spatial or high temporal resolutions. We're trying to integrate the data to provide more accurate information."
Varied data fusion approaches present limitations, including sensitivity to atmospheric disturbances and other climatic factors that can result in noise, outliers and missing data.
A proposed solution, say Hosseinzadeh, a doctoral student, and his faculty mentor Soukaina Filali Boubrahimi, is the Hydrological Generative Adversarial Network—known as Hydro-GAN. The scientists developed the Hydro-GAN model with USU colleagues Ashit Neema, Ayman Nassar and Shah Muhammad Hamdi, and describe this tool in the online issue of Water Resources Research.
Hydro-GAN, says Filali Boubrahimi, assistant professor in USU's Department of Computer Science, is a novel machine learning-based method that maps the available satellite data at low resolution to a high-resolution data counterpart.
"In our paper, we describe integrating data collected by MODIS, a spectroradiometer aboard the Terra Earth Observing System satellite, and the Landsat 8 satellite, both of which have varied spatial and temporal resolutions," she says. "We're trying to bridge the gap by generating new data samples from images collected by these satellites that improve the resolution of the shape of water boundaries."
The dataset used in this research consists of image data collected during a seven-year span (2015–2021) of 20 reservoirs in the United States, Australia, Mexico and other countries. The authors present a case study of Lake Tharthar, a salt water lake in Iraq, comparable in size to Great Salt Lake and facing similar climate and usage pressures.
"Using seven years of data from MODIS and Landsat 8, we evaluated our proposed Hydro-GAN model on Lake Tharthar's shrinking and expansion behaviors," Hosseinzadeh says. "Using Hydro-GAN, we were able to improve our predictions about the lake's changing area."
Such information is critical for the region's hydrologists and environmental scientists, he says, who need to monitor seasonal dynamics and make decisions about how to sustain the lake's water supply.
The scientists demonstrate Hydro-GAN can generate high-resolution data at historical time steps, which is otherwise unavailable, for situations where a large amount of historical data is needed for accurate forecasting.
"We think this will be a valuable tool for water managers and, moving forward with similar models, we can employ a multi-modal approach to provide data in addition to images, including information about topology, snow data amounts, streamflow, precipitation, temperature and other climate variables," says Hosseinzadeh, who presents the research during USU's 2024 Spring Runoff Conference March 26–27 in Logan, Utah.
More information: Soukaina Filali Boubrahimi et al, Spatiotemporal Data Augmentation of MODIS‐Landsat Water Bodies Using Adversarial Networks, Water Resources Research (2024). DOI: 10.1029/2023WR036342
Provided by Utah State University